AutoGPart: Intermediate Supervision Search for Generalizable 3D Part Segmentation
arXiv (Cornell University)(2022)
摘要
Training a generalizable 3D part segmentation network is quite challenging but of great importance in real-world applications. To tackle this problem, some works design task-specific solutions by translating human understanding of the task to machine's learning process, which faces the risk of missing the optimal strategy since machines do not necessarily understand in the exact human way. Others try to use conventional task-agnostic approaches designed for domain generalization problems with no task prior knowledge considered. To solve the above issues, we propose AutoGPart, a generic method enabling training generalizable 3D part segmentation networks with the task prior considered. AutoGPart builds a supervision space with geometric prior knowledge encoded, and lets the machine to search for the optimal supervisions from the space for a specific segmentation task automatically. Extensive experiments on three generalizable 3D part segmentation tasks are conducted to demonstrate the effectiveness and versatility of AutoGPart. We demonstrate that the performance of segmentation networks using simple backbones can be significantly improved when trained with supervisions searched by our method.
更多查看译文
关键词
generalizable 3d autogpart,segmentation,intermediate supervision search
AI 理解论文
溯源树
样例
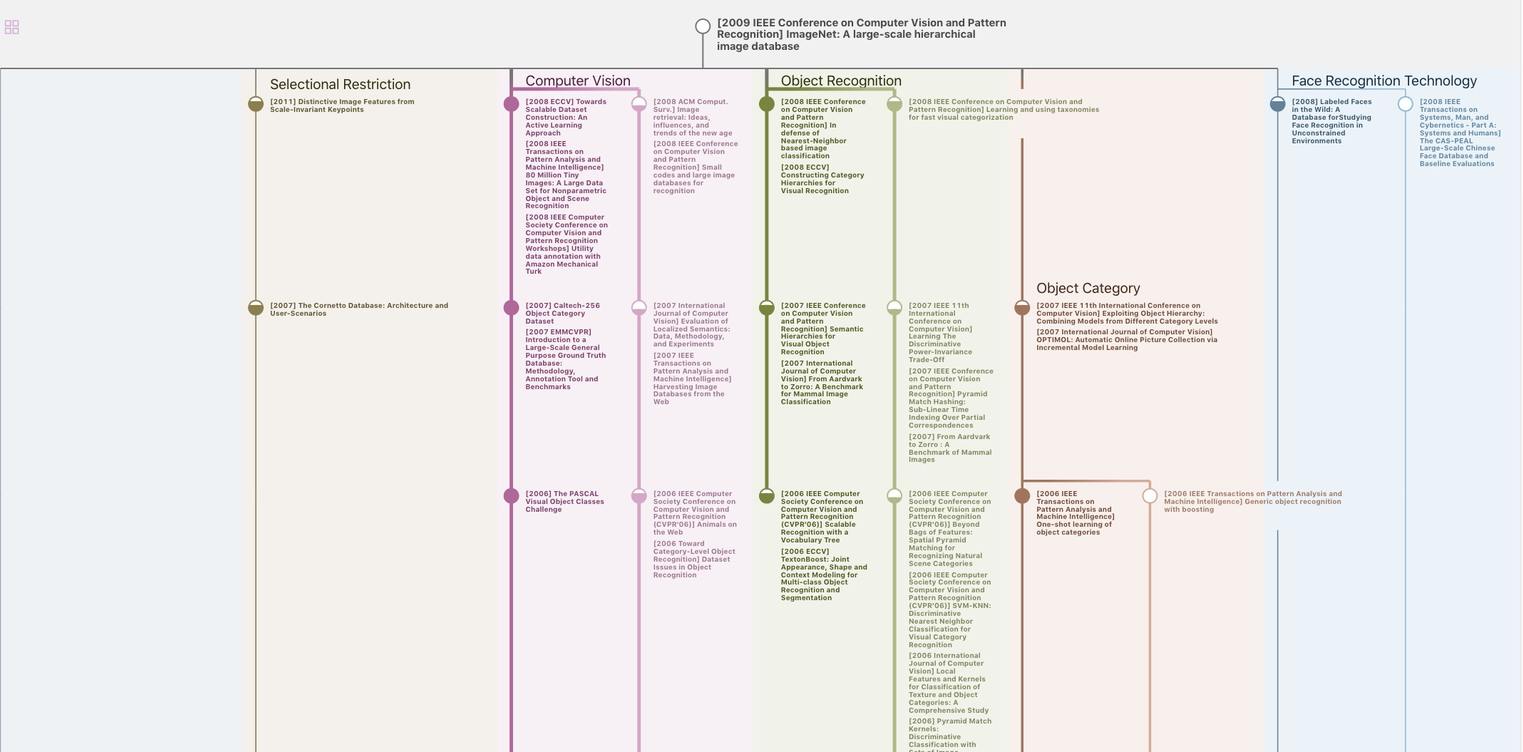
生成溯源树,研究论文发展脉络
Chat Paper
正在生成论文摘要