Distributional Reinforcement Learning via Sinkhorn Iterations
arXiv (Cornell University)(2022)
摘要
Distributional reinforcement learning~(RL) is a class of state-of-the-art algorithms that estimate the entire distribution of the total return rather than only its expectation. The empirical success of distributional RL is determined by the representation of return distributions and the choice of distribution divergence. In this paper, we propose a new class of \textit{Sinkhorn distributional RL~(SinkhornDRL)} algorithm that learns a finite set of statistics, i.e., deterministic samples, from each return distribution and then uses Sinkhorn iterations to evaluate the Sinkhorn distance between the current and target Bellmen distributions. Sinkhorn divergence features as the interpolation between the Wasserstein distance and Maximum Mean Discrepancy~(MMD). SinkhornDRL finds a sweet spot by taking advantage of the geometry of optimal transport-based distance and the unbiased gradient estimate property of MMD. Finally, compared to state-of-the-art algorithms, SinkhornDRL's competitive performance is demonstrated on the suit of 55 Atari games.
更多查看译文
关键词
sinkhorn iterations,reinforcement learning
AI 理解论文
溯源树
样例
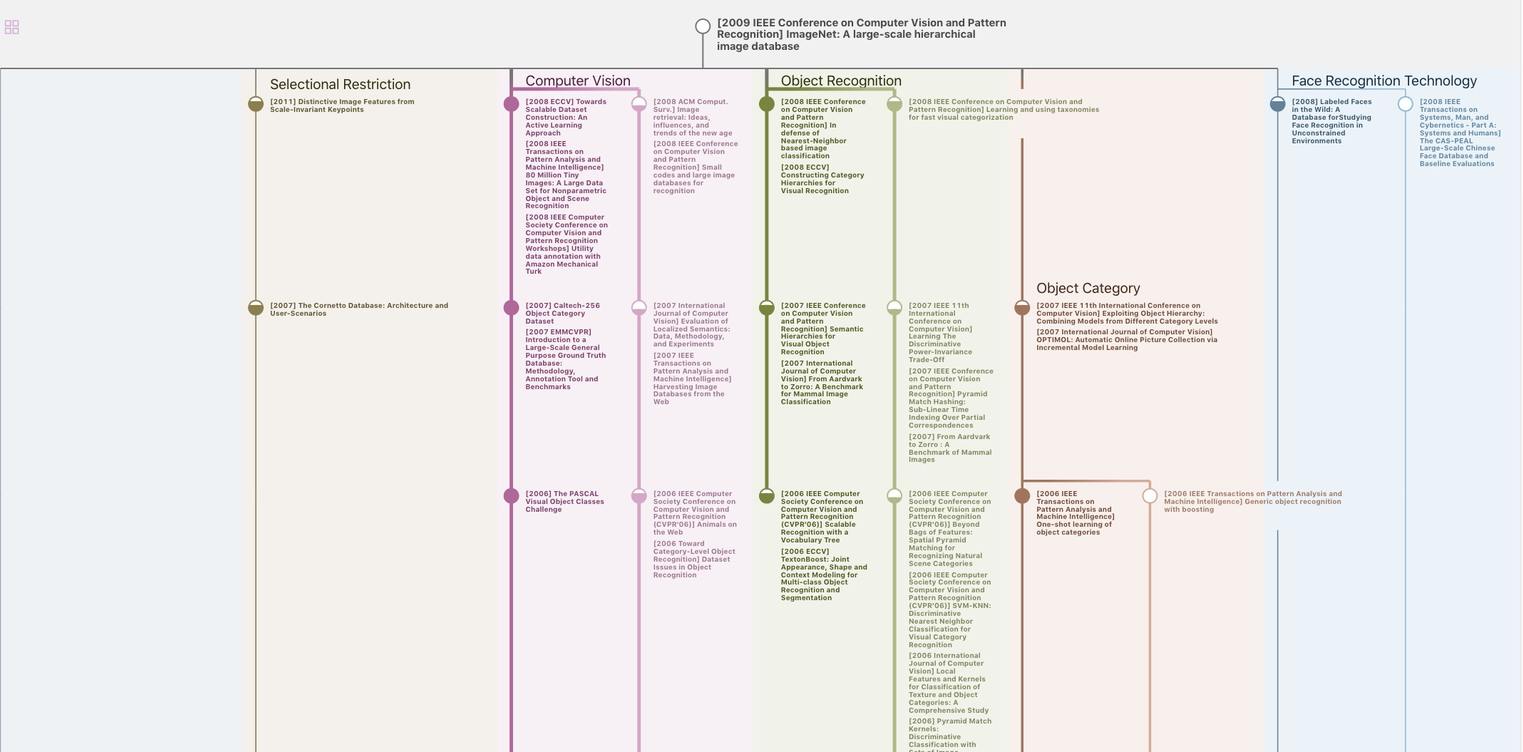
生成溯源树,研究论文发展脉络
Chat Paper
正在生成论文摘要