Large Neural Networks Learning from Scratch with Very Few Data and without Regularization
arXiv (Cornell University)(2022)
摘要
Recent findings have shown that Neural Networks generalize also in over-parametrized regimes with zero training error. This is surprising, since it is completely against traditional machine learning wisdom. In our empirical study we fortify these findings in the domain of fine-grained image classification. We show that very large Convolutional Neural Networks with millions of weights do learn with only a handful of training samples and without image augmentation, explicit regularization or pretraining. We train the architectures ResNet018, ResNet101 and VGG19 on subsets of the difficult benchmark datasets Caltech101, CUB_200_2011, FGVCAircraft, Flowers102 and StanfordCars with 100 classes and more, perform a comprehensive comparative study and draw implications for the practical application of CNNs. Finally, we show that VGG19 with 140 million weights learns to distinguish airplanes and motorbikes up to 95% accuracy with only 20 samples per class.
更多查看译文
关键词
large neural networks learning,neural networks
AI 理解论文
溯源树
样例
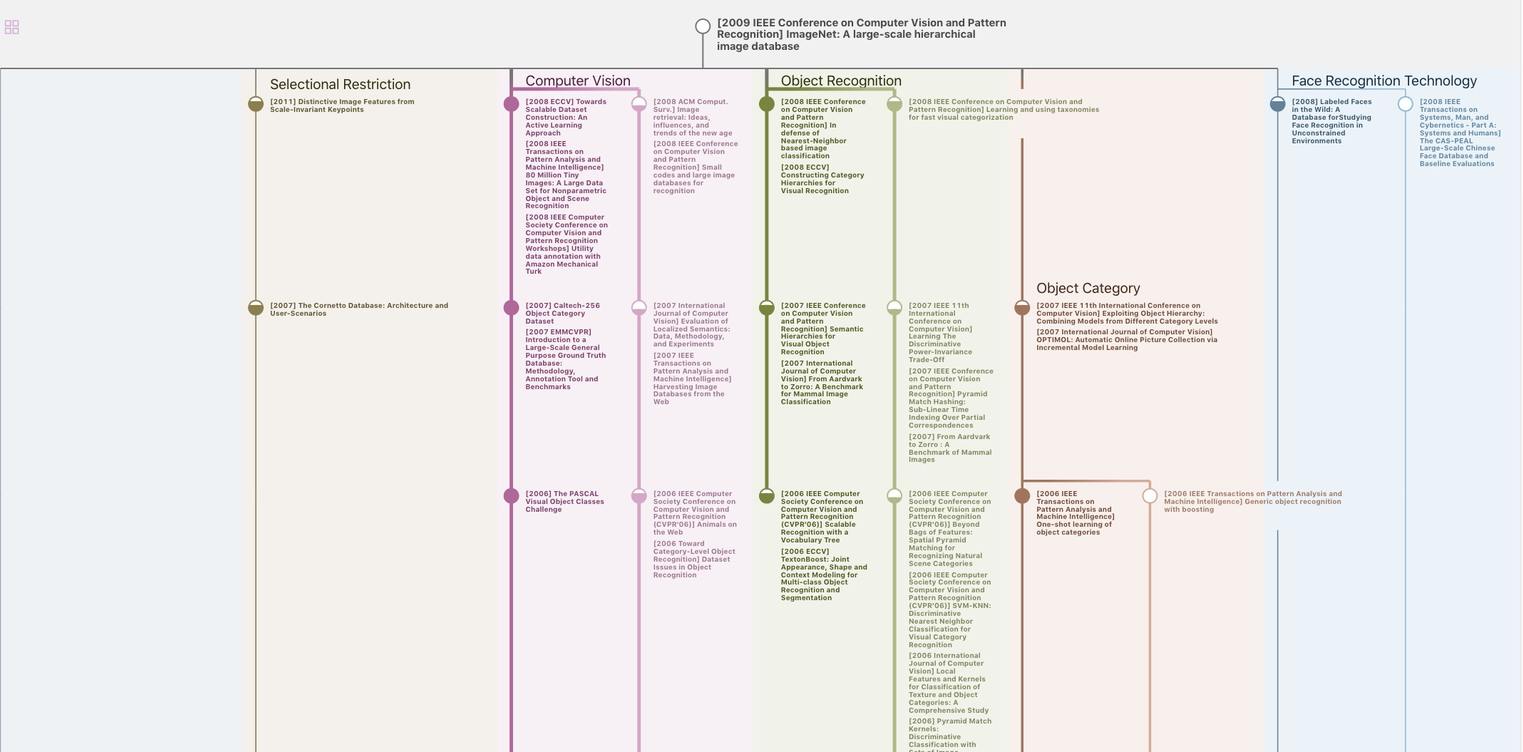
生成溯源树,研究论文发展脉络
Chat Paper
正在生成论文摘要