KRLS: Improving End-to-End Response Generation in Task Oriented Dialog with Reinforced Keywords Learning
arXiv (Cornell University)(2022)
摘要
In task-oriented dialogs (TOD), reinforcement learning (RL) algorithms train a model to directly optimize response for task-related metrics. However, RL needs to perform exploration, which can be time-consuming due to the slow auto-regressive sequence generation process. We investigate an approach to create a more efficient RL-based algorithm to improve TOD performance in an offline setting. First, we use a faster generation procedure that samples from independent next-word distributions after training the language model (LM) with supervised learning. We then introduce a fine-grained reward function to help the model focus on learning key information in a dialog, by measuring the importance and semantic closeness of each generated token. Experiments on the MultiWoZ dataset show our new training algorithm, Keywords Reinforcement Learning with Next-word Sampling (KRLS), achieves state-of-the-art performance on the end-to-end response generation task, with a 15% training time reduction compared to a standard RL algorithm using auto-regressive generation.
更多查看译文
关键词
dialog
AI 理解论文
溯源树
样例
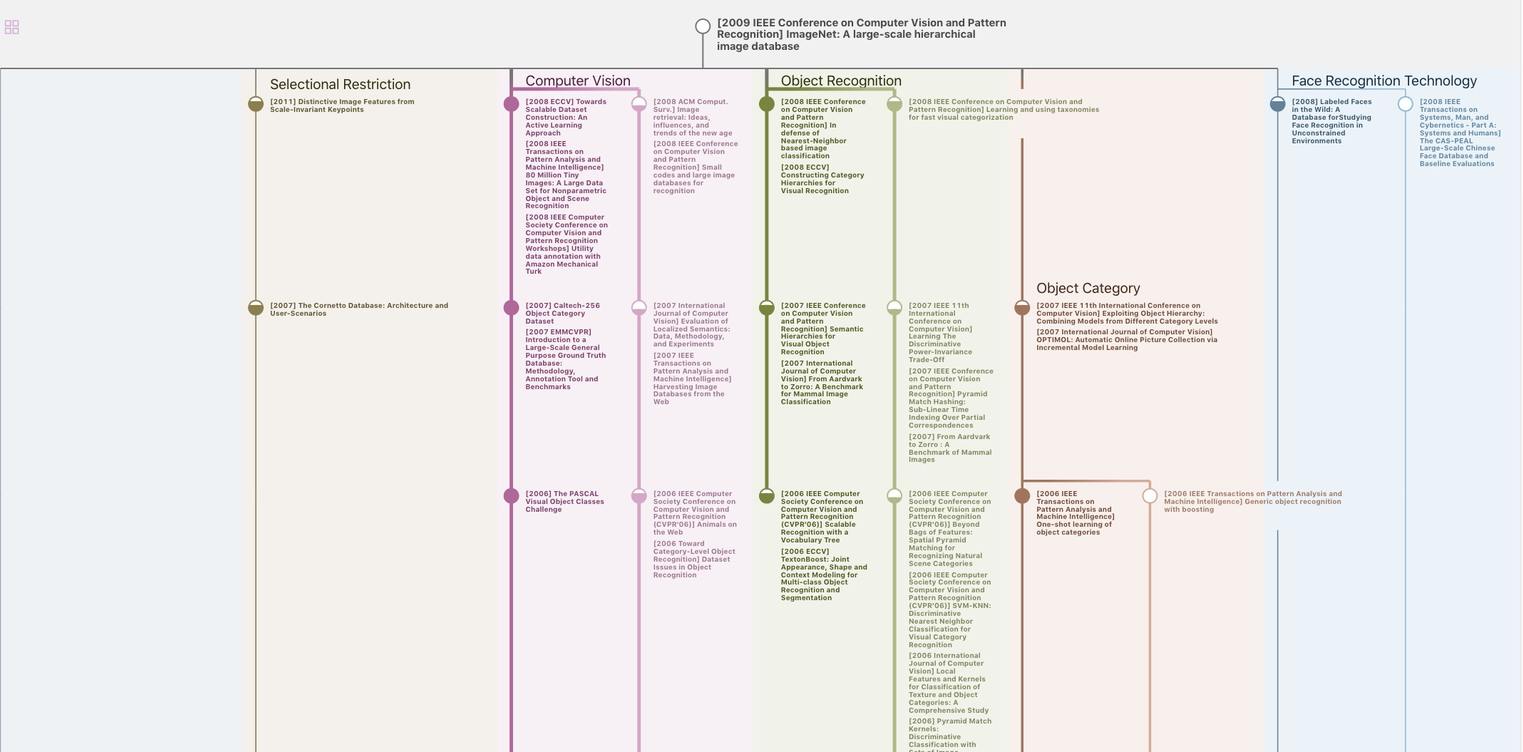
生成溯源树,研究论文发展脉络
Chat Paper
正在生成论文摘要