Conversational Speech Recognition By Learning Conversation-level Characteristics
arXiv (Cornell University)(2022)
摘要
Conversational automatic speech recognition (ASR) is a task to recognize conversational speech including multiple speakers. Unlike sentence-level ASR, conversational ASR can naturally take advantages from specific characteristics of conversation, such as role preference and topical coherence. This paper proposes a conversational ASR model which explicitly learns conversation-level characteristics under the prevalent end-to-end neural framework. The highlights of the proposed model are twofold. First, a latent variational module (LVM) is attached to a conformer-based encoder-decoder ASR backbone to learn role preference and topical coherence. Second, a topic model is specifically adopted to bias the outputs of the decoder to words in the predicted topics. Experiments on two Mandarin conversational ASR tasks show that the proposed model achieves a maximum 12% relative character error rate (CER) reduction.
更多查看译文
关键词
speech,learning,recognition,conversation-level
AI 理解论文
溯源树
样例
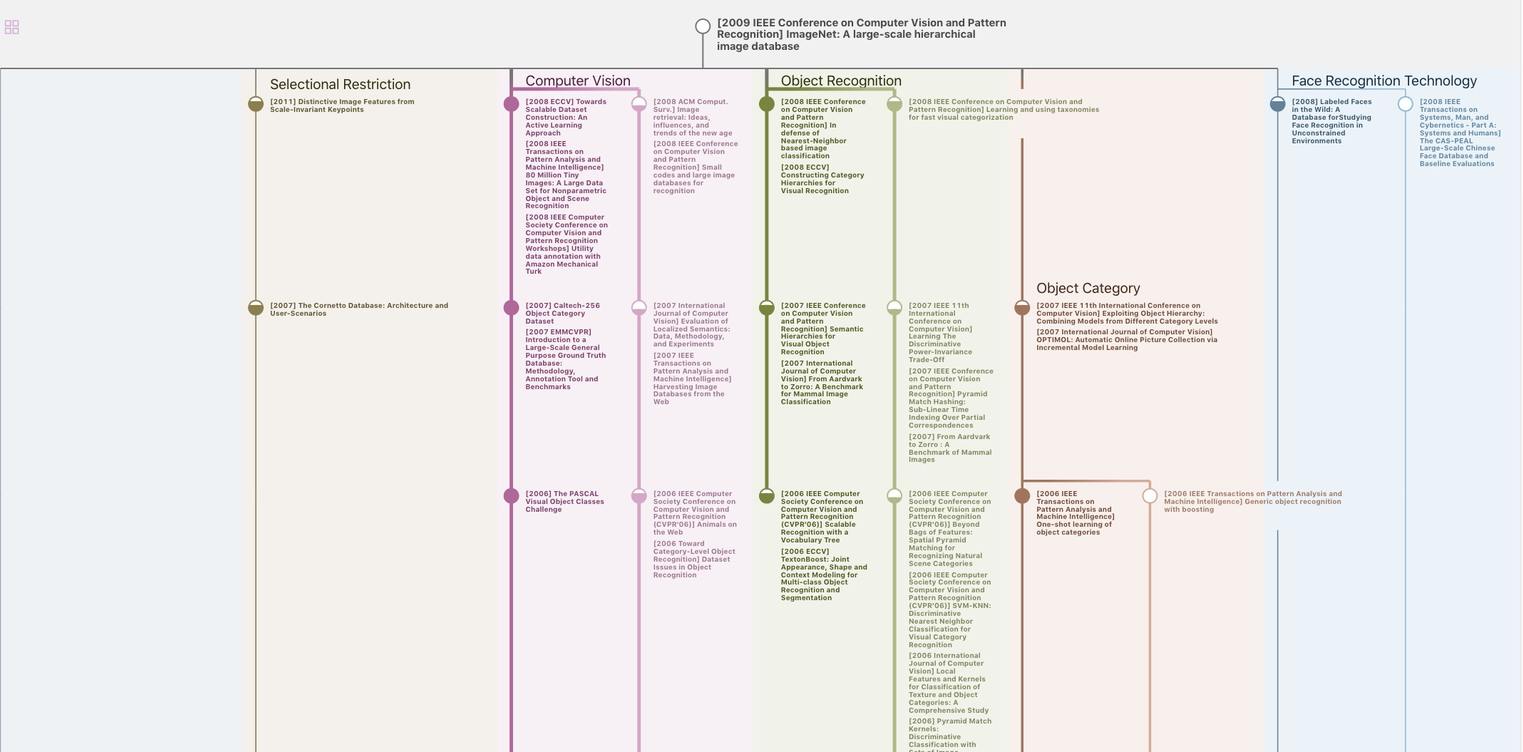
生成溯源树,研究论文发展脉络
Chat Paper
正在生成论文摘要