Pseudo-grading of tumor subclones using phenotype algebra
bioRxiv (Cold Spring Harbor Laboratory)(2022)
摘要
Abstract Robust characterization of cellular phenotypes from single-cell gene expression data is of paramount importance in studying complex biological systems and diseases. Single-cell RNA-sequencing (scRNA- seq), coupled with robust computational analysis, facilitates characterization of phenotypic heterogeneity in tumors. Current scRNA-seq analysis pipelines are capable of accurately identifying a myriad of malignant and non-malignant cell subtypes from single-cell profiling of tumor microenvironments. Unfortunately, given the extent of phenotypic heterogeneity, it is not straightforward to assess the risk associated with individual malignant cell subpopulations in a tumor, primarily due to the complexity of the cancer phenotype space and the lack of clinical annotations associated with tumor scRNA-seq studies, involving prospectively collected tissue samples. Effective risk-stratification of individual malignant subclones holds promise for formulating tailored therapeutic interventions. To this end, we present SCellBOW, a computational approach that facilitates risk-stratification by leveraging scRNA-seq profiles and language modeling techniques. We compared SCellBOW with existing best practice methods for its ability to precisely represent phenotypically divergent cell types across multiple scRNA-seq datasets, including our in-house generated human splenocyte and matched peripheral blood mononuclear cell (PBMC) dataset. SCellBOW offers a remarkable feature for executing algebraic operations such as ’+’ and ’–’ on single-cells in the latent space while preserving the biological meanings. This feature catalyzes the simulation of the residual phenotype of tumors, following positive and negative selection of specific malignant cell subtypes in a tumor. As a proof of concept, we tested and validated phenotype algebra across three independent cancer types – glioblastoma multiforme, breast cancer and metastatic prostate cancer. In particular, we demonstrate how the negative selection of specific clones may lead to variable prognosis. From the metastatic prostate cancer scRNA-seq data, SCellBOW identifies a hitherto unknown and pervasive AR−/NE low (androgen receptor negative, neuroendocrine-low) malignant cell subpopulation with a conspicuously high predictive risk score. We could trace this back in a large-scale spatial omics atlas of 141 well-characterized metastatic prostate cancer samples at the spot resolution.
更多查看译文
关键词
tumor subclones,phenotype,pseudo-grading
AI 理解论文
溯源树
样例
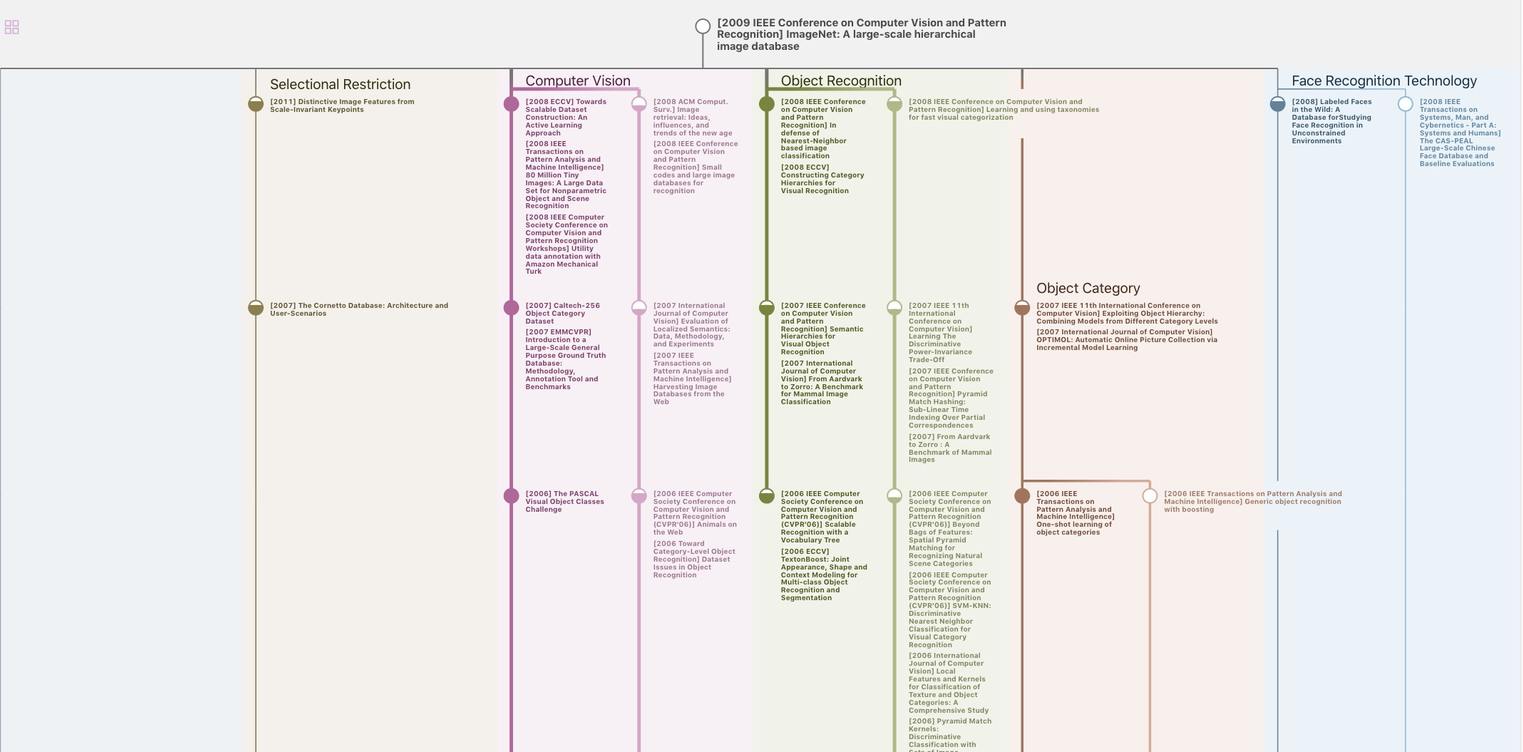
生成溯源树,研究论文发展脉络
Chat Paper
正在生成论文摘要