Complete identification of complex salt-geometries from inaccurate migrated images using Deep Learning
arXiv (Cornell University)(2022)
摘要
Delimiting salt inclusions from migrated images is a time-consuming activity that relies on highly human-curated analysis and is subject to interpretation errors or limitations of the methods available. We propose to use migrated images produced from an inaccurate velocity model (with a reasonable approximation of sediment velocity, but without salt inclusions) to predict the correct salt inclusions shape using a Convolutional Neural Network (CNN). Our approach relies on subsurface Common Image Gathers to focus the sediments' reflections around the zero offset and to spread the energy of salt reflections over large offsets. Using synthetic data, we trained a U-Net to use common-offset subsurface images as input channels for the CNN and the correct salt-masks as network output. The network learned to predict the salt inclusions masks with high accuracy; moreover, it also performed well when applied to synthetic benchmark data sets that were not previously introduced. Our training process tuned the U-Net to successfully learn the shape of complex salt bodies from partially focused subsurface offset images.
更多查看译文
关键词
deep learning,images,identification,salt-geometries
AI 理解论文
溯源树
样例
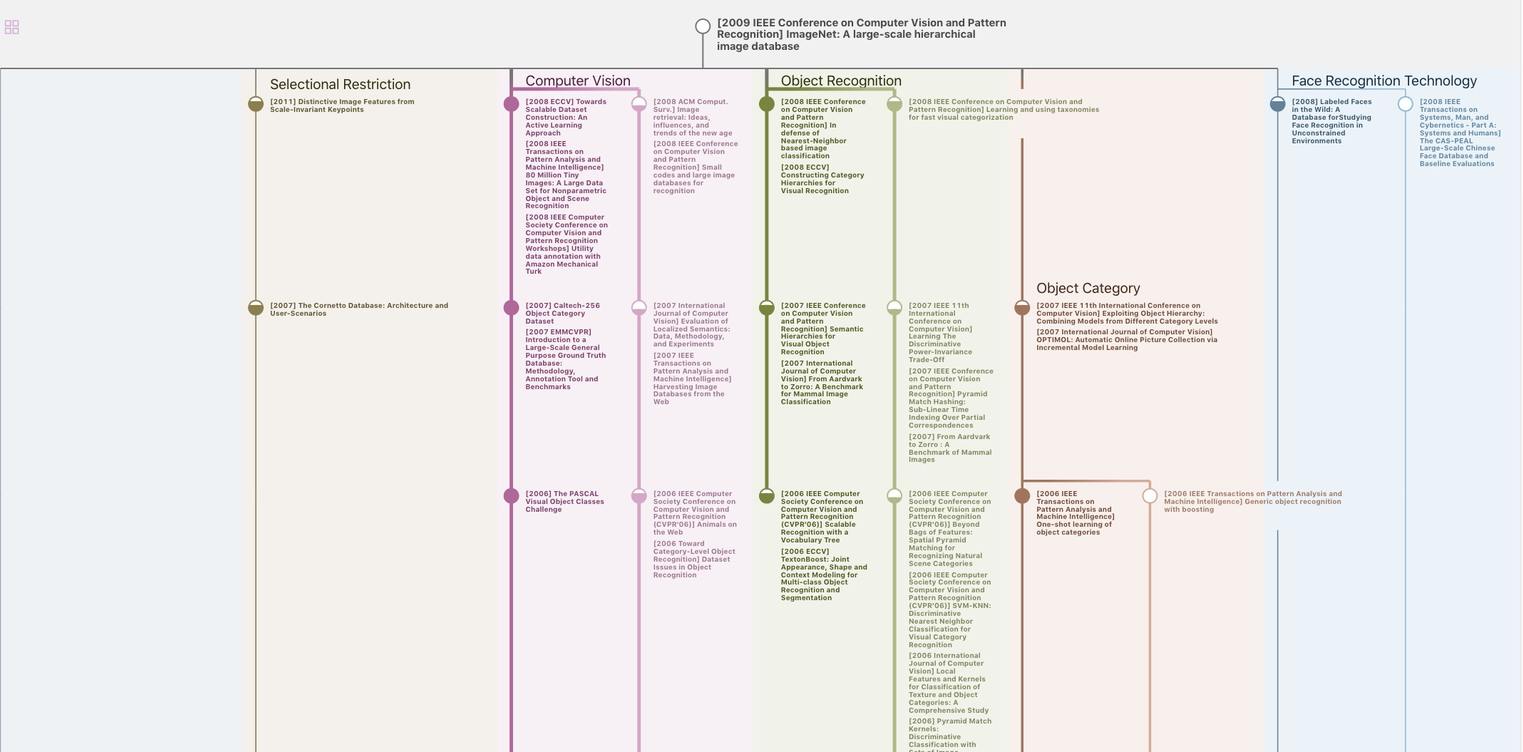
生成溯源树,研究论文发展脉络
Chat Paper
正在生成论文摘要