IntrinsicNeRF: Learning Intrinsic Neural Radiance Fields for Editable Novel View Synthesis
2023 IEEE/CVF INTERNATIONAL CONFERENCE ON COMPUTER VISION, ICCV(2023)
摘要
Existing inverse rendering combined with neural rendering methods can only perform editable novel view synthesis on object-specific scenes, while we present intrinsic neural radiance fields, dubbed IntrinsicNeRF, which introduce intrinsic decomposition into the NeRF-based neural rendering method and can extend its application to room-scale scenes. Since intrinsic decomposition is a fundamentally under-constrained inverse problem, we propose a novel distance-aware point sampling and adaptive reflectance iterative clustering optimization method, which enables IntrinsicNeRF with traditional intrinsic decomposition constraints to be trained in an unsupervised manner, resulting in multi-view consistent intrinsic decomposition results. To cope with the problem that different adjacent instances of similar reflectance in a scene are incorrectly clustered together, we further propose a hierarchical clustering method with coarse-to-fine optimization to obtain a fast hierarchical indexing representation. It supports compelling real-time augmented applications such as recoloring and illumination variation. Extensive experiments and editing samples on both object-specific/room-scale scenes and synthetic/real-word data demonstrate that we can obtain consistent intrinsic decomposition results and high-fidelity novel view synthesis even for challenging sequences.
更多查看译文
关键词
intrinsicnerf neural radiance fields,view,synthesis,learning
AI 理解论文
溯源树
样例
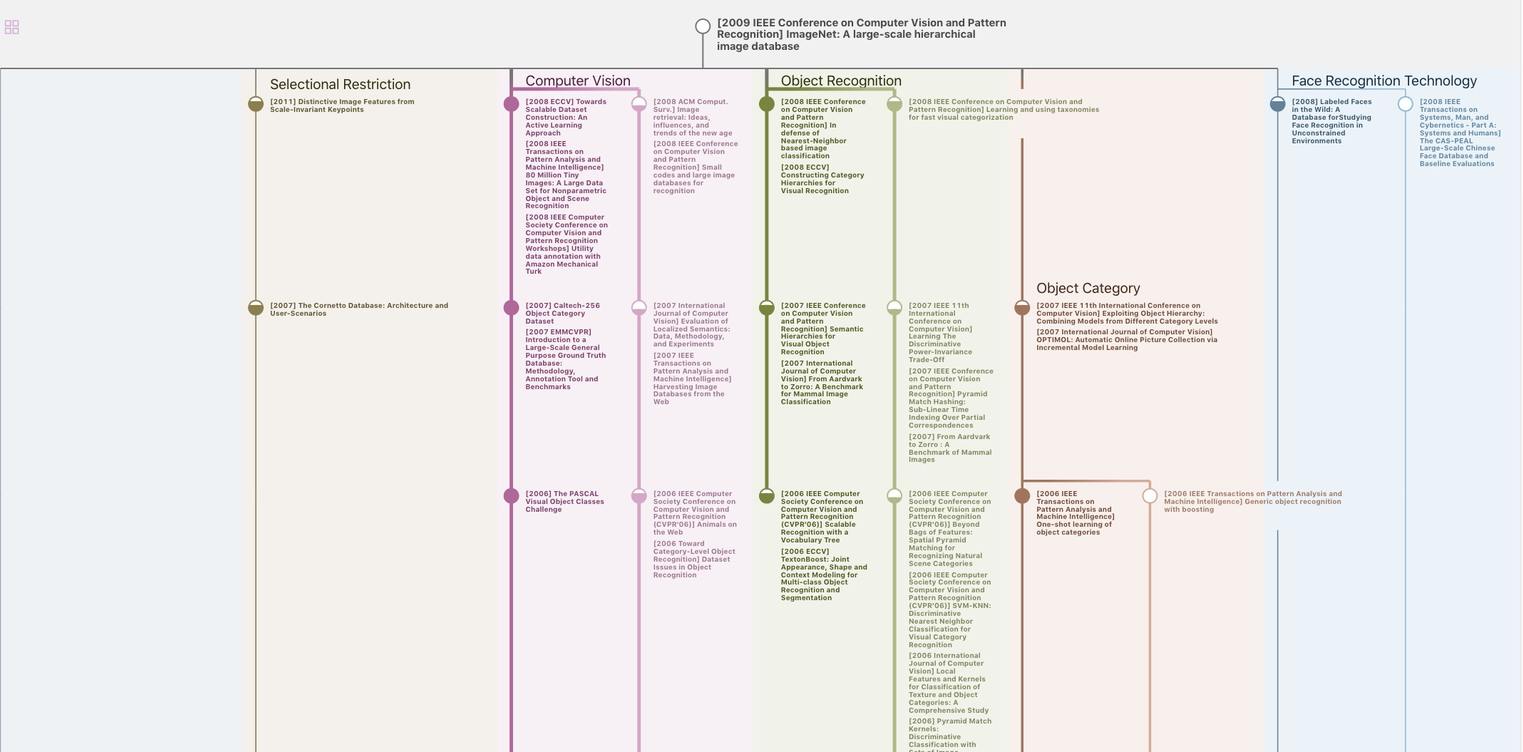
生成溯源树,研究论文发展脉络
Chat Paper
正在生成论文摘要