Cyclical Compound Domain Test-time Adaptation via Continual Domain-Matching Algorithm
arXiv (Cornell University)(2022)
摘要
Test-time adaptation (TTA), a key component of lifelong learning in edge devices, refers to the ability of a pre-trained model to adapt itself to new environments during test time. Due to its practical ability, TTA has attracted significant attention and experienced a rapid performance boost these days. In this paper, we present an under-explored yet more realistic TTA scenario and provide a strong baseline favorable to this scenario, named cyclical compound domain (CCD). The CCD represents the real-world scenario in which the target domain contains multiple sub-target domains (i.e., compound domain due to weather or time change) and the sub-target domains are likely to rise cyclically. Unfortunately, existing works do not faithfully account for this plausible scenario, only focusing on adapting to the current sub-target domain while discarding the past knowledge acquired from repeated sub-target domains. Therefore, we first propose a lightweight domain-matching algorithm that allows the TTA model to manage knowledge from the compound domain. This algorithm identifies the type of domain among sub-target domains by continuously matching the current image's distribution with reference domain points. Moreover, our newly proposed regularization method compares the present distribution with source one in order to regularize the adaptation pace according to each data in sub-target domains. Qualitatively, we demonstrate that our simple-yet-effective approach improves the adaptation performance on various benchmarks, including image classification on ImageNet-C and semantic segmentation on GTA5, C-driving datasets, and Cityscapes with corruptions.
更多查看译文
关键词
adaptation,test-time,domain-matching
AI 理解论文
溯源树
样例
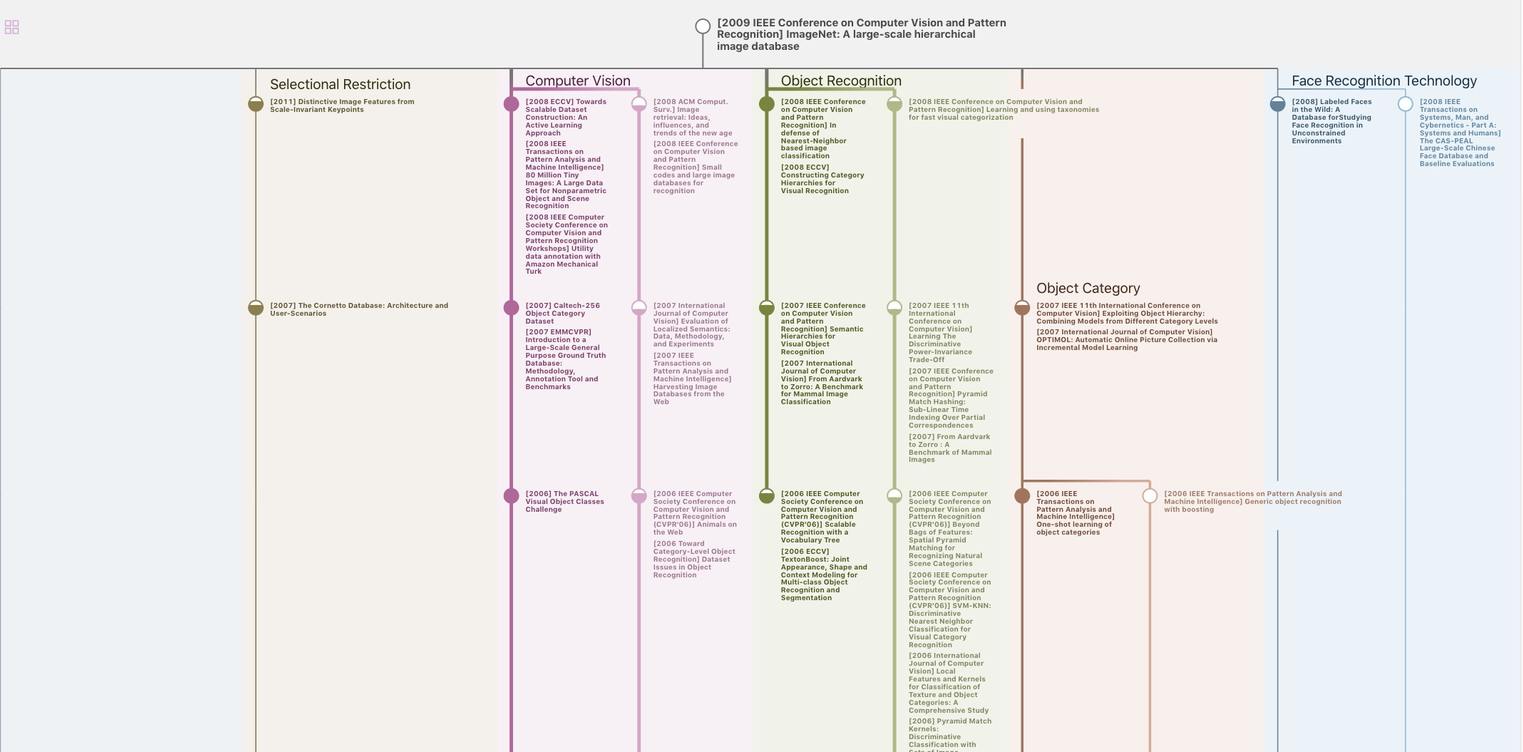
生成溯源树,研究论文发展脉络
Chat Paper
正在生成论文摘要