Discovery and Exploitation of Generalized Network Effects
arXiv (Cornell University)(2022)
摘要
Given a large graph with few node labels, how can we (a) identify whether there is generalized network-effects (GNE) of the graph or not, (b) estimate GNE to explain the interrelations among node classes, and (c) exploit GNE to improve downstream tasks such as predicting the unknown labels accurately and efficiently? The knowledge of GNE is valuable for various tasks like node classification and targeted advertising. However, identifying and understanding GNE such as homophily, heterophily or their combination is challenging in real-world graphs due to limited availability of node labels and noisy edges. We propose NetEffect, a graph mining approach to address the above issues, enjoying the following properties: (i) Principled: a statistical test to determine the presence of GNE in a graph with few node labels; (ii) General and Explainable: a closed-form solution to estimate the specific type of GNE observed; and (iii) Accurate and Scalable: the integration of GNE for accurate and fast node classification. Applied on public, real-world graphs, NetEffect discovers the unexpected absence of GNE in numerous graphs, which previously thought to exhibit heterophily. Further, we show that incorporating GNE is effective on node classification. On a large real-world graph with 1.6M nodes and 22.3M edges, NetEffect achieves over 7 times speedup (14 minutes vs. 2 hours) compared to most competitors.
更多查看译文
关键词
discovery,effects,exploitation
AI 理解论文
溯源树
样例
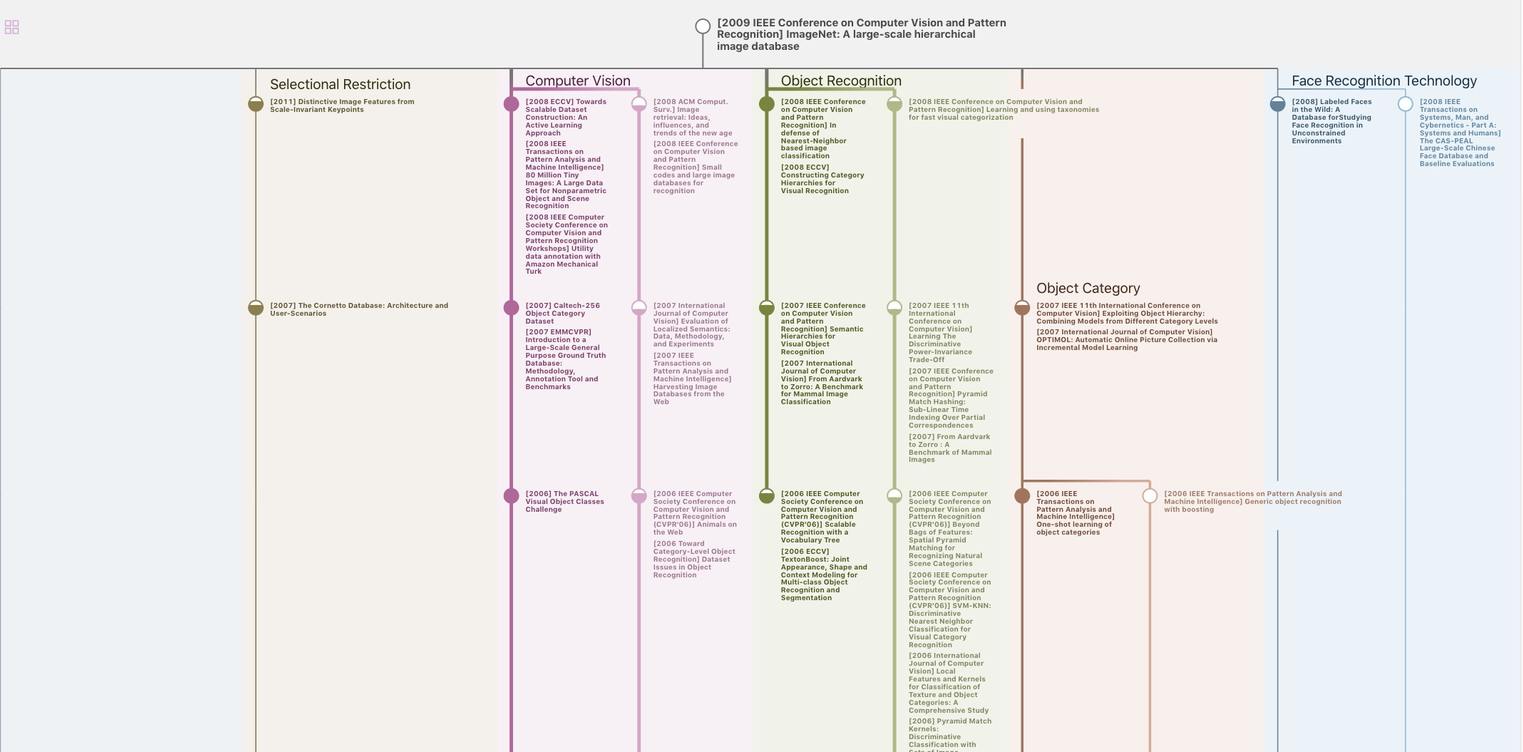
生成溯源树,研究论文发展脉络
Chat Paper
正在生成论文摘要