Deep Fusion Network Based Sparse View CT Reconstructions for Clinical Diagnostic Scanners
2023 45TH ANNUAL INTERNATIONAL CONFERENCE OF THE IEEE ENGINEERING IN MEDICINE & BIOLOGY SOCIETY, EMBC(2023)
摘要
Sparse view CT scan has the advantage of reducing radiation exposure and scanning time in clinical diagnosis. However, the limited number of x-ray projections can make the reconstruction problem ill posed and result in image artifacts. To tackle the problem, we propose a novel model-based deep fusion network(DFN) satisfying the clinical set-up. It extracts fused features encoded from both the sinogram and the preliminary reconstructed image generated by filtered back projection (FBP) to improve the quality of reconstruction. The preliminary reconstructed image endows fused features with prior knowledge that facilitate the convergence of neural network to high-quality reconstruction images. We design a custom loss for training that enforces the network to learn both the pixel value and the integrity of the tissue structure. A synthetic sparse view breast CT dataset from American Association of Physicists in Medicine(AAPM) is used for training, validation and testing. The qualitative and quantitative evaluations show that the DFN reconstruction algorithm significantly improves in balancing between the image quality and reconstruction speed, hence enables fast and high quality CT reconstruction despite the sparse view limitations.
更多查看译文
AI 理解论文
溯源树
样例
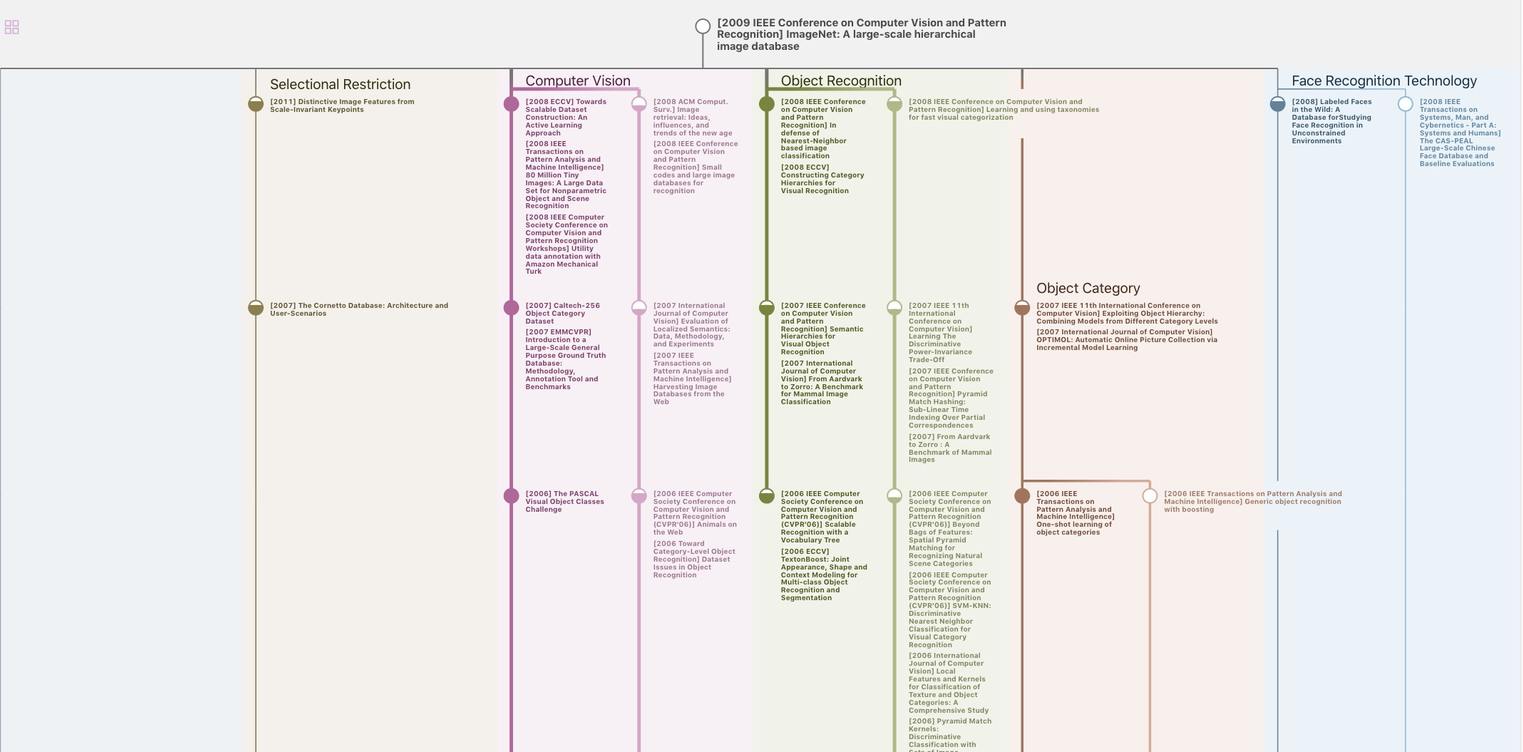
生成溯源树,研究论文发展脉络
Chat Paper
正在生成论文摘要