Federated PCA on Grassmann Manifold for Anomaly Detection in IoT Networks
arXiv (Cornell University)(2022)
摘要
In the era of Internet of Things (IoT), network-wide anomaly detection is a crucial part of monitoring IoT networks due to the inherent security vulnerabilities of most IoT devices. Principal Components Analysis (PCA) has been proposed to separate network traffics into two disjoint subspaces corresponding to normal and malicious behaviors for anomaly detection. However, the privacy concerns and limitations of devices' computing resources compromise the practical effectiveness of PCA. We propose a federated PCA-based Grassmannian optimization framework that coordinates IoT devices to aggregate a joint profile of normal network behaviors for anomaly detection. First, we introduce a privacy-preserving federated PCA framework to simultaneously capture the profile of various IoT devices' traffic. Then, we investigate the alternating direction method of multipliers gradient-based learning on the Grassmann manifold to guarantee fast training and the absence of detecting latency using limited computational resources. Empirical results on the NSL-KDD dataset demonstrate that our method outperforms baseline approaches. Finally, we show that the Grassmann manifold algorithm is highly adapted for IoT anomaly detection, which permits drastically reducing the analysis time of the system. To the best of our knowledge, this is the first federated PCA algorithm for anomaly detection meeting the requirements of IoT networks.
更多查看译文
关键词
grassmann manifold,anomaly detection,federated pca,iot
AI 理解论文
溯源树
样例
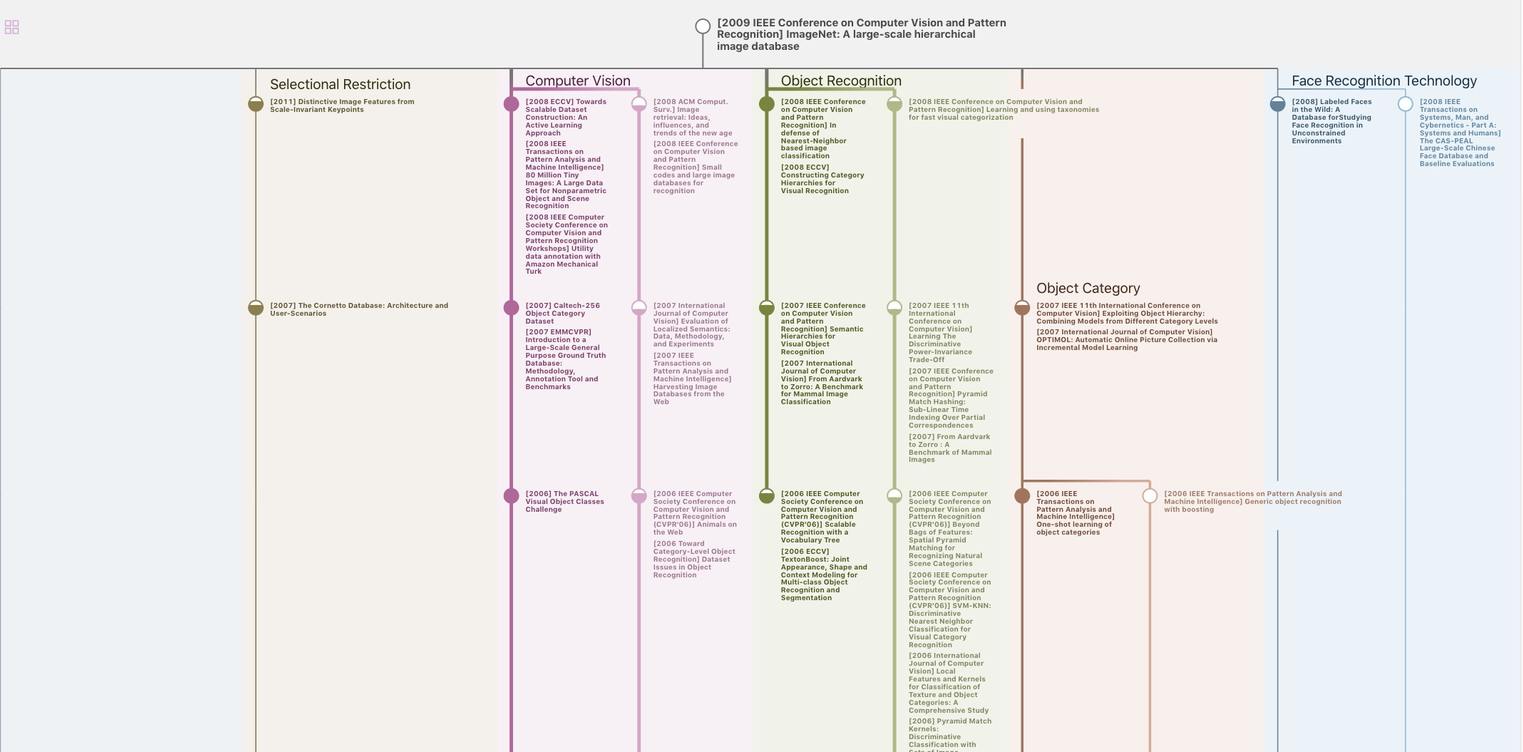
生成溯源树,研究论文发展脉络
Chat Paper
正在生成论文摘要