Federated Learning with Packet Losses
2023 26TH INTERNATIONAL SYMPOSIUM ON WIRELESS PERSONAL MULTIMEDIA COMMUNICATIONS, WPMC(2023)
摘要
This paper tackles the problem of training Federated Learning (FL) algorithms over real-world wireless networks with packet losses. Lossy communication channels between the orchestrating server and the clients affect the convergence of FL training as well as the quality of the learned model. Although many previous works investigated how to mitigate the adverse effects of packet losses, this paper demonstrates that FL algorithms over asymmetric lossy channels can still learn the optimal model, the same model that would have been trained in a lossless scenario by classic FL algorithms like FedAvg. Convergence to the optimum only requires slight changes to FedAvg: i) while FedAvg computes a new global model by averaging the received clients' models, our algorithm, UPGA-PL, updates the global model by a pseudo-gradient step; ii) UPGA-PL accounts for the potentially heterogeneous packet losses experienced by the clients to unbias the pseudo-gradient step. Still, UPGA-PL maintains the same computational and communication complexity as FedAvg. In our experiments, UPGA-PL not only outperforms existing state-of-the-art solutions for lossy channels (by more than 5 percentage points on test accuracy) but also matches FedAvg's performance in lossless scenarios after less than 150 communication rounds.
更多查看译文
关键词
Federated Learning,Packet Loss
AI 理解论文
溯源树
样例
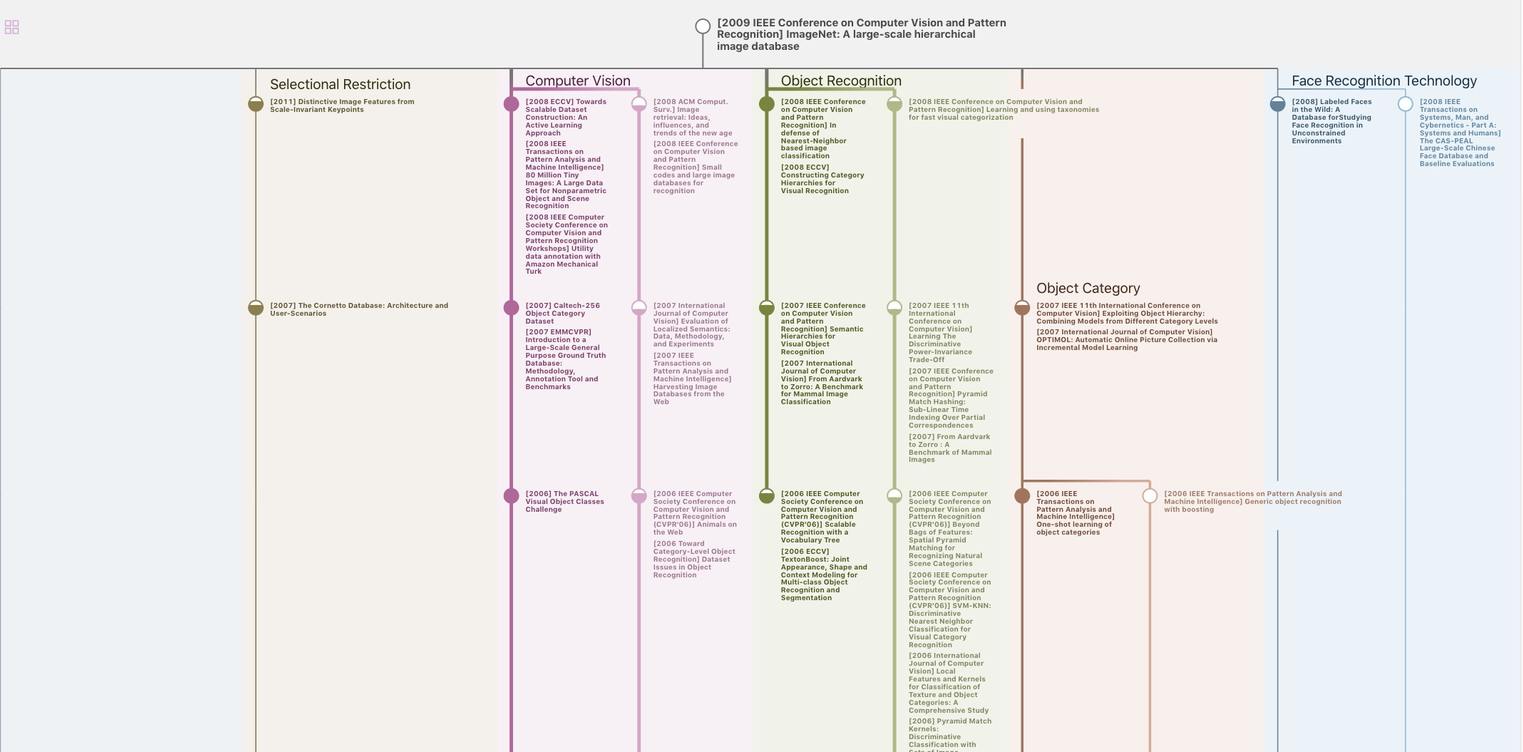
生成溯源树,研究论文发展脉络
Chat Paper
正在生成论文摘要