The Recon Approach: A New Direction for Machine Learning in Criminal Law
Social Science Research Network(2021)
摘要
Most applications of machine learning in criminal law focus on making predictions about people and using those predictions to guide decisions. For example, judges use risk assessment tools to predict the likelihood of future violence when making decisions about whom to detain pre-trial. Whereas this predictive technology analyzes people about whom decisions are made, we propose a new direction for machine learning that scrutinizes decision-making itself. Our aim is not to predict behavior, but to provide the public with data-driven opportunities to improve the fairness and consistency of human discretionary judgment. We call our approach the Recon Approach because it encompasses two functions: reconnaissance and reconsideration. Reconnaissance harnesses natural language processing to cull through thousands of hearing transcripts and illuminate factors that appear to have influenced decisions at those hearings. Reconsideration uses modeling techniques to identify cases that appear anomalous in a way that warrants a closer review of those decisions. Reconnaissance reveals patterns that may show systemic problems across a set of decisions; reconsideration flags potential errors or injustices in individual cases. As a team of computer scientists and legal scholars, we describe our early work to apply the Recon Approach to parole-release decisions in California. Drawing on that work, we discuss challenges to the Recon Approach, as well as its potential to apply to sentencing and other discretionary decision-making contexts within and beyond criminal law.
更多查看译文
关键词
criminal law,recon approach,machine learning
AI 理解论文
溯源树
样例
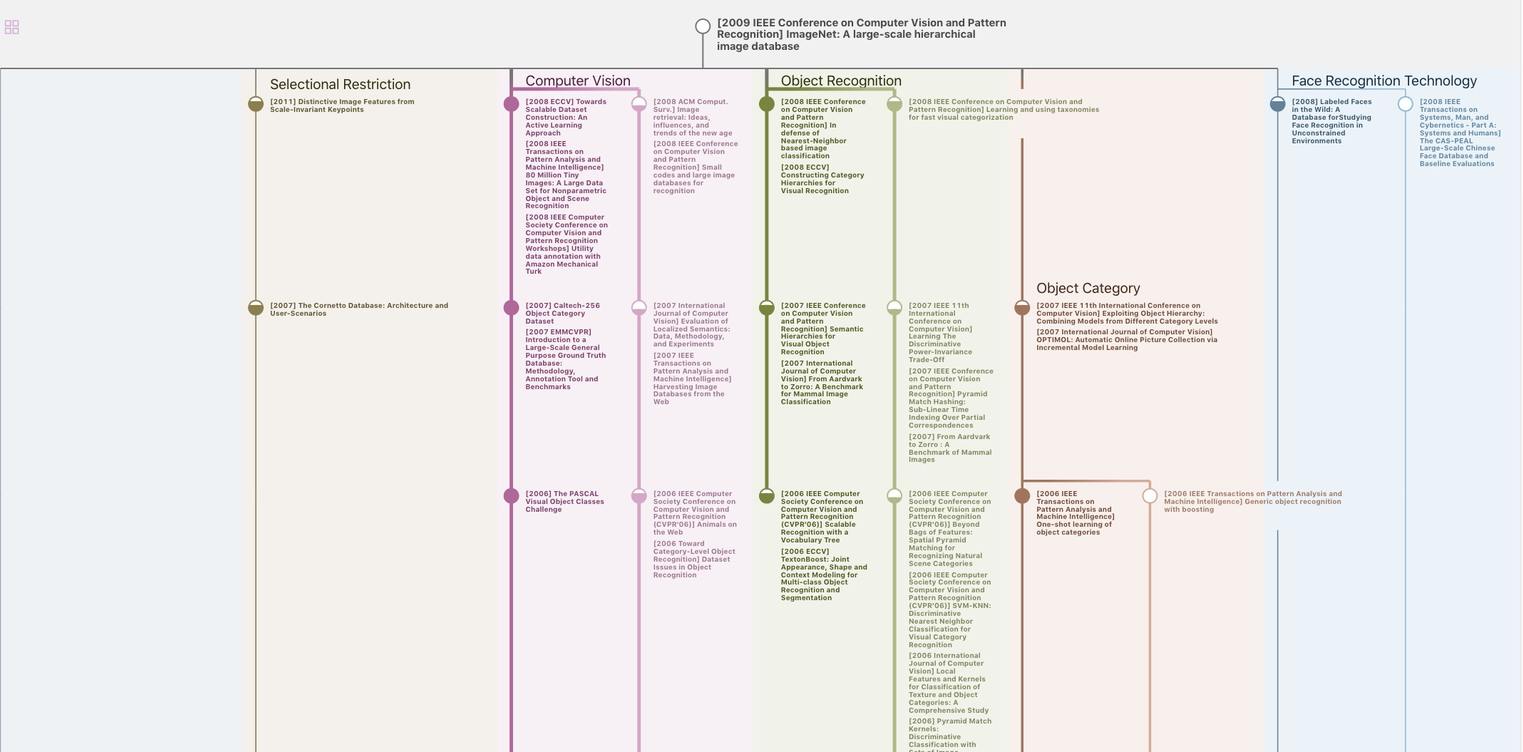
生成溯源树,研究论文发展脉络
Chat Paper
正在生成论文摘要