Detecting Polarized Topics in COVID-19 News Using Partisanship-aware Contextualized Topic Embeddings
arXiv (Cornell University)(2021)
摘要
Growing polarization of the news media has been blamed for fanning disagreement, controversy and even violence. Early identification of polarized topics is thus an urgent matter that can help mitigate conflict. However, accurate measurement of polarization is still an open research challenge. To address this gap, we propose Partisanship-aware Contextualized Topic Embeddings (PaCTE), a method to automatically detect polarized topics from partisan news sources. Specifically, we represent the ideology of a news source on a topic by corpus-contextualized topic embedding utilizing a language model that has been finetuned on recognizing partisanship of the news articles, and measure the polarization between sources using cosine similarity. We apply our method to a corpus of news about COVID-19 pandemic. Extensive experiments on different news sources and topics demonstrate the effectiveness of our method to precisely capture the topical polarization and alignment between different news sources. To help clarify and validate results, we explain the polarization using the Moral Foundation Theory.
更多查看译文
关键词
topics,embeddings,topics,partisanship-aware
AI 理解论文
溯源树
样例
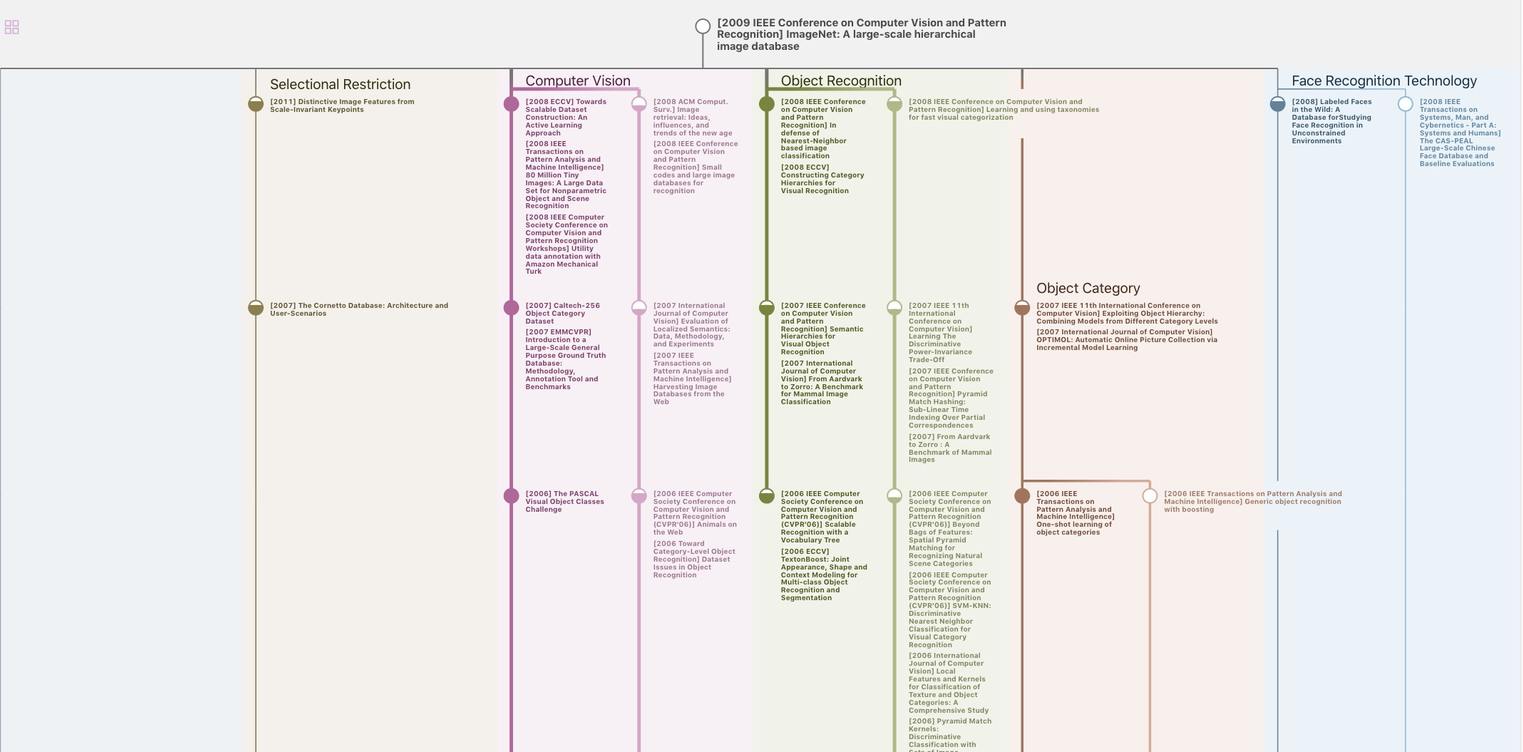
生成溯源树,研究论文发展脉络
Chat Paper
正在生成论文摘要