Multifidelity Modeling for Physics-Informed Neural Networks (PINNs)
arXiv (Cornell University)(2021)
摘要
Multifidelity simulation methodologies are often used in an attempt to judiciously combine low-fidelity and high-fidelity simulation results in an accuracy-increasing, cost-saving way. Candidates for this approach are simulation methodologies for which there are fidelity differences connected with significant computational cost differences. Physics-informed Neural Networks (PINNs) are candidates for these types of approaches due to the significant difference in training times required when different fidelities (expressed in terms of architecture width and depth as well as optimization criteria) are employed. In this paper, we propose a particular multifidelity approach applied to PINNs that exploits low-rank structure. We demonstrate that width, depth, and optimization criteria can be used as parameters related to model fidelity, and show numerical justification of cost differences in training due to fidelity parameter choices. We test our multifidelity scheme on various canonical forward PDE models that have been presented in the emerging PINNs literature.
更多查看译文
关键词
neural networks,pinns,physics-informed
AI 理解论文
溯源树
样例
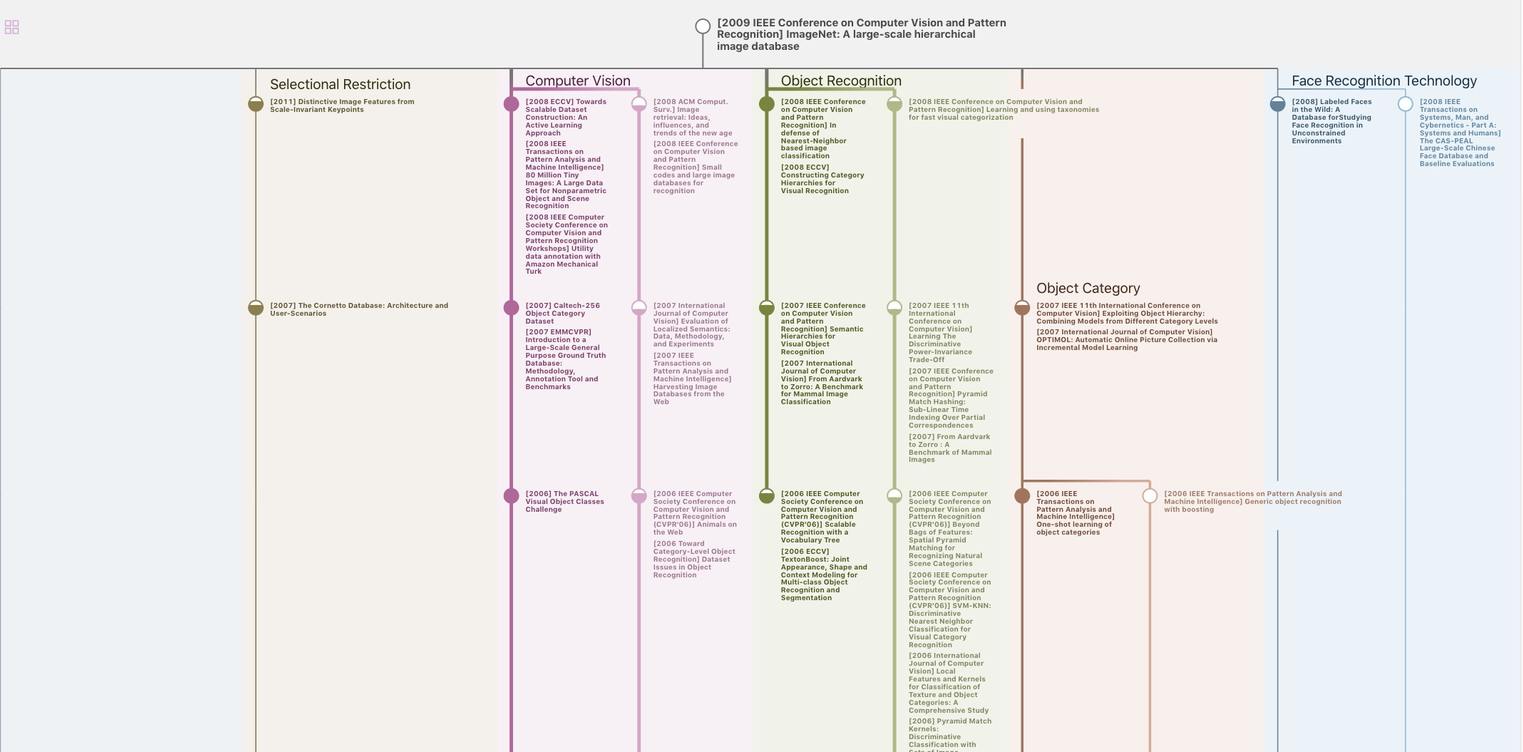
生成溯源树,研究论文发展脉络
Chat Paper
正在生成论文摘要