Direct Simultaneous Speech-to-Speech Translation with Variational Monotonic Multihead Attention
arXiv (Cornell University)(2021)
摘要
We present a direct simultaneous speech-to-speech translation (Simul-S2ST) model, Furthermore, the generation of translation is independent from intermediate text representations. Our approach leverages recent progress on direct speech-to-speech translation with discrete units, in which a sequence of discrete representations, instead of continuous spectrogram features, learned in an unsupervised manner, are predicted from the model and passed directly to a vocoder for speech synthesis on-the-fly. We also introduce the variational monotonic multihead attention (V-MMA), to handle the challenge of inefficient policy learning in speech simultaneous translation. The simultaneous policy then operates on source speech features and target discrete units. We carry out empirical studies to compare cascaded and direct approach on the Fisher Spanish-English and MuST-C English-Spanish datasets. Direct simultaneous model is shown to outperform the cascaded model by achieving a better tradeoff between translation quality and latency.
更多查看译文
关键词
attention,variational
AI 理解论文
溯源树
样例
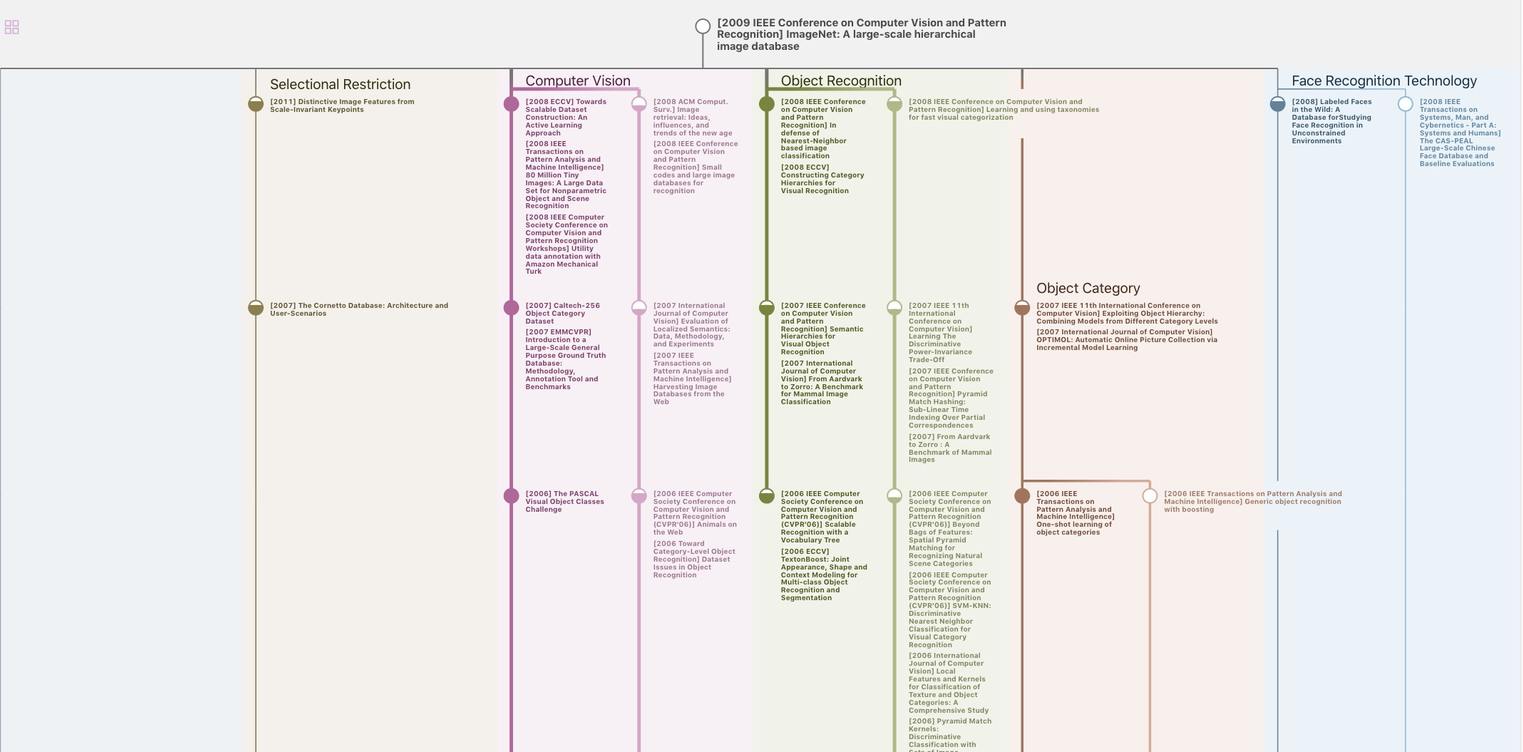
生成溯源树,研究论文发展脉络
Chat Paper
正在生成论文摘要