Bridging the Gap between Spatial and Spectral Domains: A Theoretical Framework for Graph Neural Networks
arXiv (Cornell University)(2021)
摘要
During the past decade, deep learning's performance has been widely recognized in a variety of machine learning tasks, ranging from image classification, speech recognition to natural language understanding. Graph neural networks (GNN) are a type of deep learning that is designed to handle non-Euclidean issues using graph-structured data that are difficult to solve with traditional deep learning techniques. The majority of GNNs were created using a variety of processes, including random walk, PageRank, graph convolution, and heat diffusion, making direct comparisons impossible. Previous studies have primarily focused on classifying current models into distinct categories, with little investigation of their internal relationships. This research proposes a unified theoretical framework and a novel perspective that can methodologically integrate existing GNN into our framework. We survey and categorize existing GNN models into spatial and spectral domains, as well as show linkages between subcategories within each domain. Further investigation reveals a strong relationship between the spatial, spectral, and subgroups of these domains.
更多查看译文
关键词
graph neural networks,spectral domains,neural networks
AI 理解论文
溯源树
样例
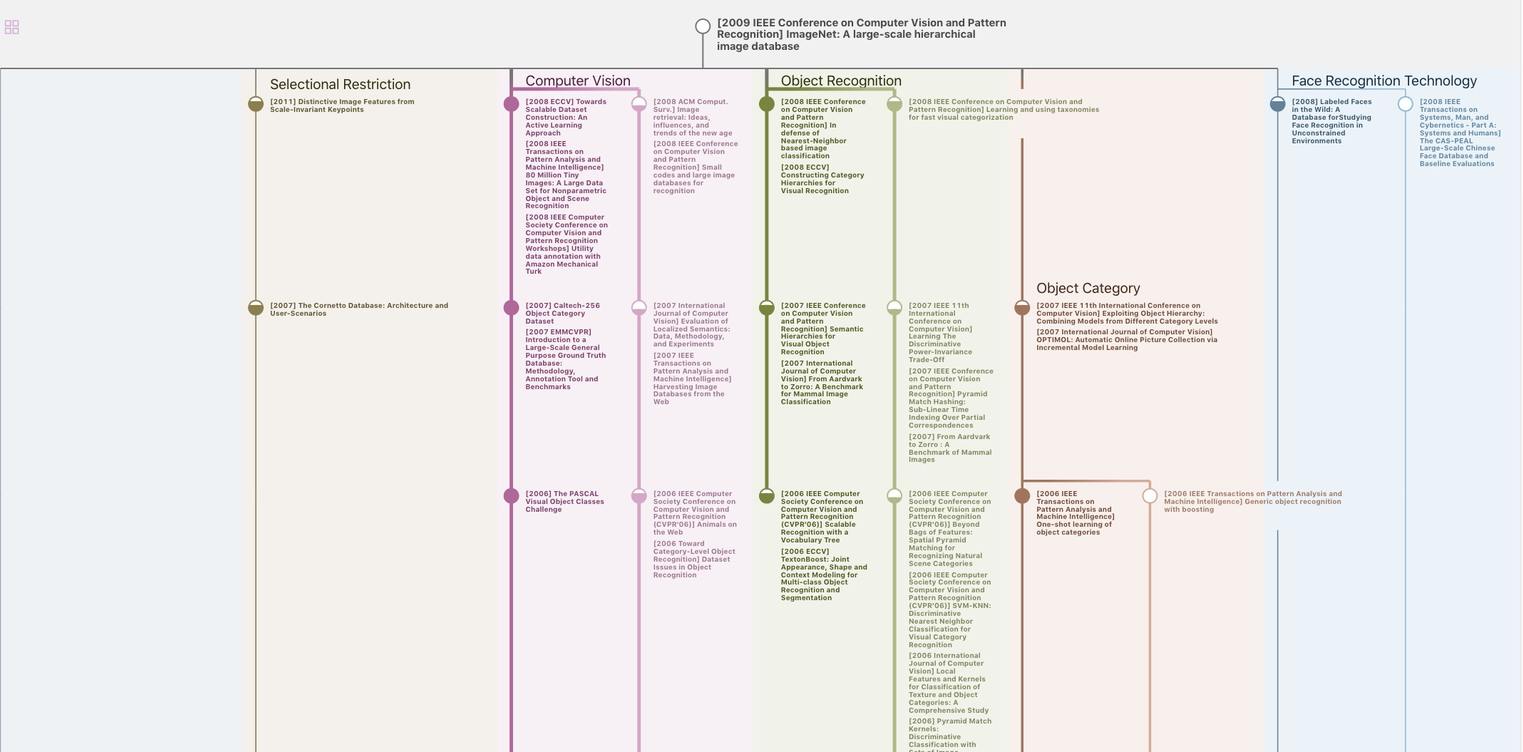
生成溯源树,研究论文发展脉络
Chat Paper
正在生成论文摘要