On Multi-objective Policy Optimization as a Tool for Reinforcement Learning.
arXiv (Cornell University)(2021)
摘要
Many advances that have improved the robustness and efficiency of deep reinforcement learning (RL) algorithms can, in one way or another, be understood as introducing additional objectives, or constraints, in the policy optimization step. This includes ideas as far ranging as exploration bonuses, entropy regularization, and regularization toward teachers or data priors when learning from experts or in offline RL. Often, task reward and auxiliary objectives are in conflict with each other and it is therefore natural to treat these examples as instances of multi-objective (MO) optimization problems. We study the principles underlying MORL and introduce a new algorithm, Distillation of a Mixture of Experts (DiME), that is intuitive and scale-invariant under some conditions. We highlight its strengths on standard MO benchmark problems and consider case studies in which we recast offline RL and learning from experts as MO problems. This leads to a natural algorithmic formulation that sheds light on the connection between existing approaches. For offline RL, we use the MO perspective to derive a simple algorithm, that optimizes for the standard RL objective plus a behavioral cloning term. This outperforms state-of-the-art on two established offline RL benchmarks.
更多查看译文
关键词
reinforcement learning,optimization,policy,multi-objective
AI 理解论文
溯源树
样例
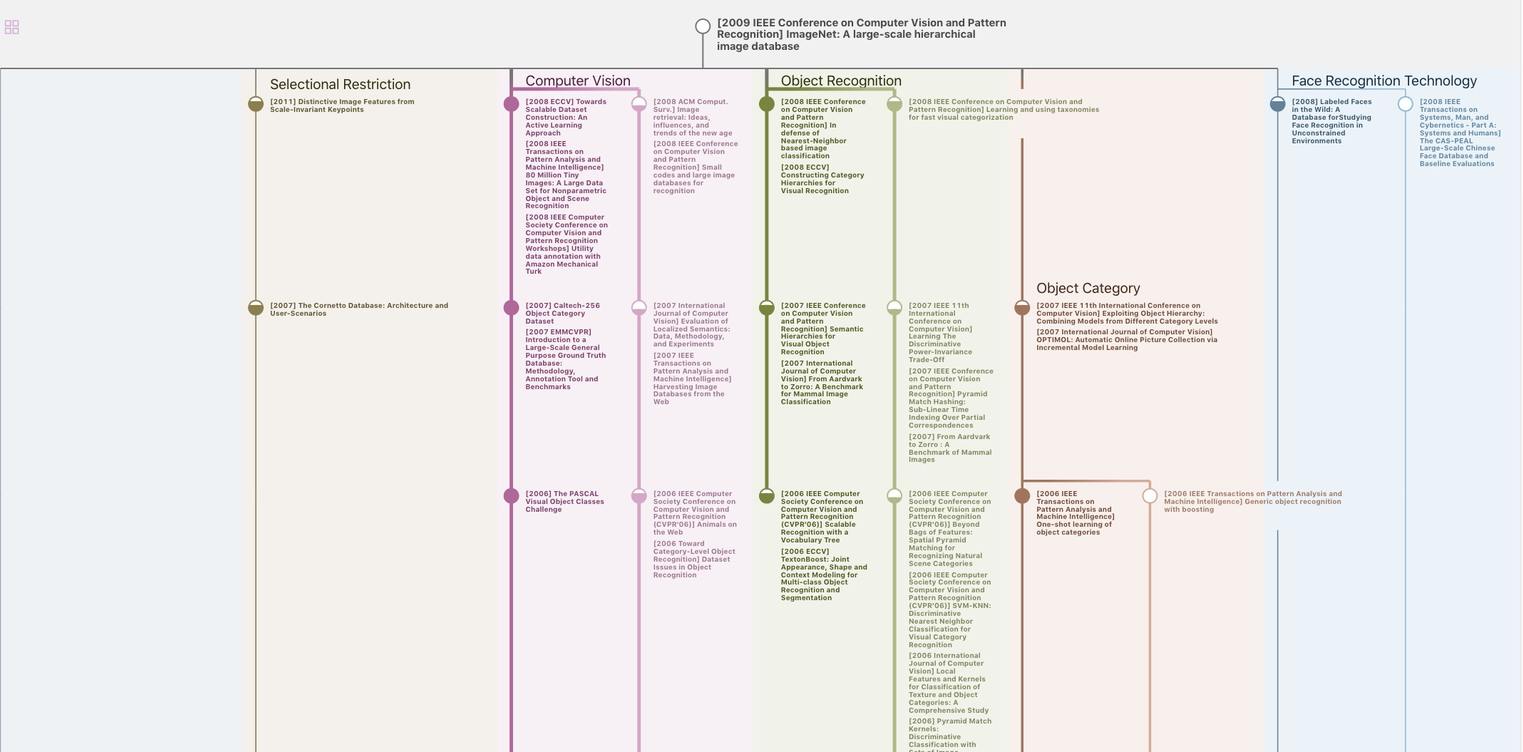
生成溯源树,研究论文发展脉络
Chat Paper
正在生成论文摘要