Bayesian Edge Regression in Undirected Graphical Models to Characterize Interpatient Heterogeneity in Cancer
arXiv (Cornell University)(2021)
摘要
Graphical models are commonly used to discover associations within gene or protein networks for complex diseases such as cancer. Most existing methods estimate a single graph for a population, while in many cases, researchers are interested in characterizing the heterogeneity of individual networks across subjects with respect to subject-level covariates. Examples include assessments of how the network varies with patient-specific prognostic scores or comparisons of tumor and normal graphs while accounting for tumor purity as a continuous predictor. In this paper, we propose a novel edge regression model for undirected graphs, which estimates conditional dependencies as a function of subject-level covariates. Bayesian shrinkage algorithms are used to induce sparsity in the underlying graphical models. We assess our model performance through simulation studies focused on comparing tumor and normal graphs while adjusting for tumor purity and a case study assessing how blood protein networks in hepatocellular carcinoma patients vary with severity of disease, measured by HepatoScore, a novel biomarker signature measuring disease severity.
更多查看译文
关键词
bayesian edge regression,undirected graphical models,interpatient heterogeneity,cancer
AI 理解论文
溯源树
样例
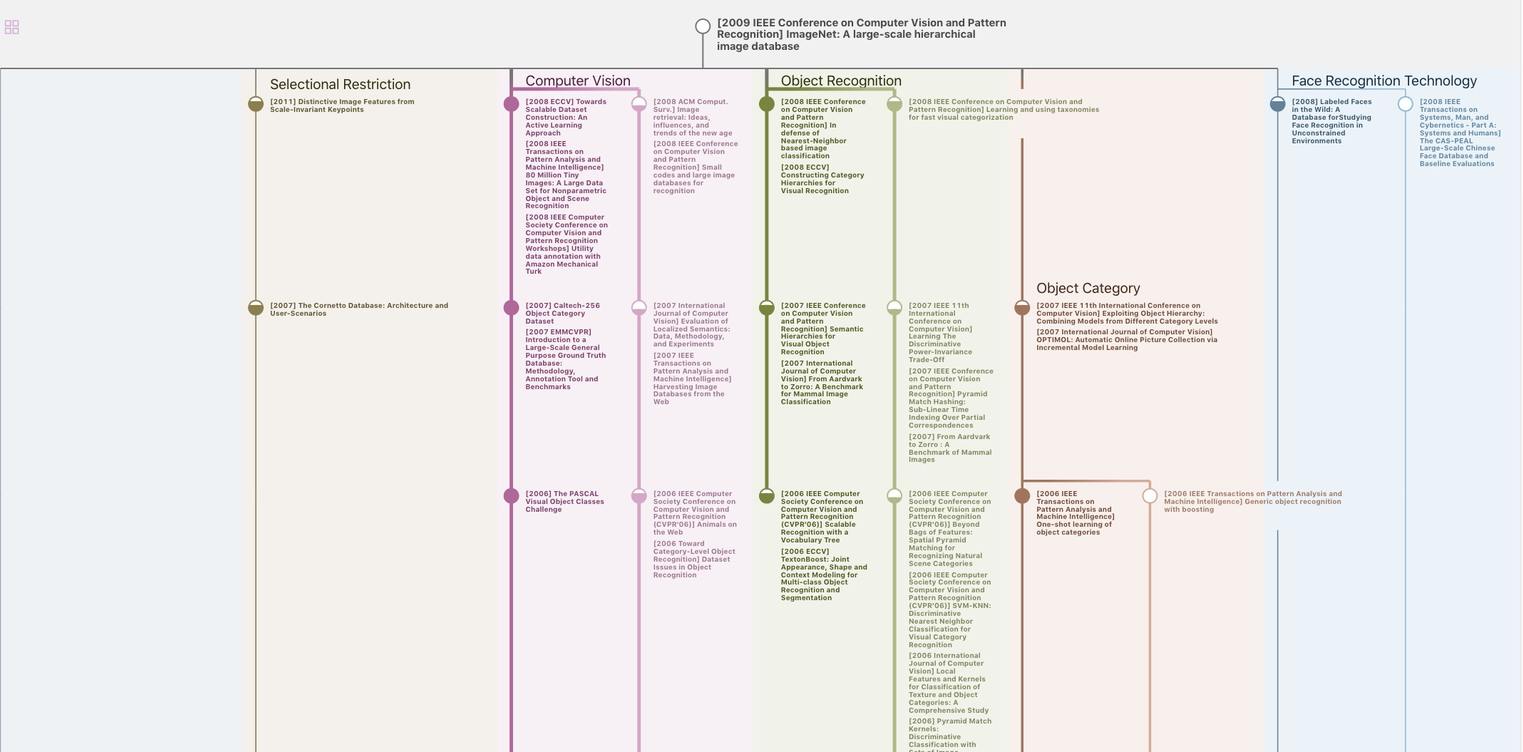
生成溯源树,研究论文发展脉络
Chat Paper
正在生成论文摘要