Learn2Reg: comprehensive multi-task medical image registration challenge, dataset and evaluation in the era of deep learning
arXiv (Cornell University)(2021)
摘要
To date few studies have comprehensively compared medical image registration approaches on a wide-range of complementary clinically relevant tasks. This limits the adoption of advances in research into practice and prevents fair benchmarks across competing approaches. Many newer learning-based methods have been explored within the last five years, but the question which optimisation, architectural or metric strategy is ideally suited remains open. Learn2Reg covers a wide range of anatomies: brain, abdomen and thorax, modalities: ultrasound, CT, MRI, populations: intra- and inter-patient and levels of supervision. We established a lower entry barrier for training and validation of 3D registration, which helped us compile results of over 65 individual method submissions from more than 20 unique teams. Our complementary set of metrics, including robustness, accuracy, plausibility and speed enables unique insight into the current-state-of-the-art of medical image registration. Further analyses into transferability, bias and importance of supervision question the superiority of primarily deep learning based approaches and open exiting new research directions into hybrid methods that leverage GPU-accelerated conventional optimisation.
更多查看译文
关键词
deep learning,dataset,multi-task
AI 理解论文
溯源树
样例
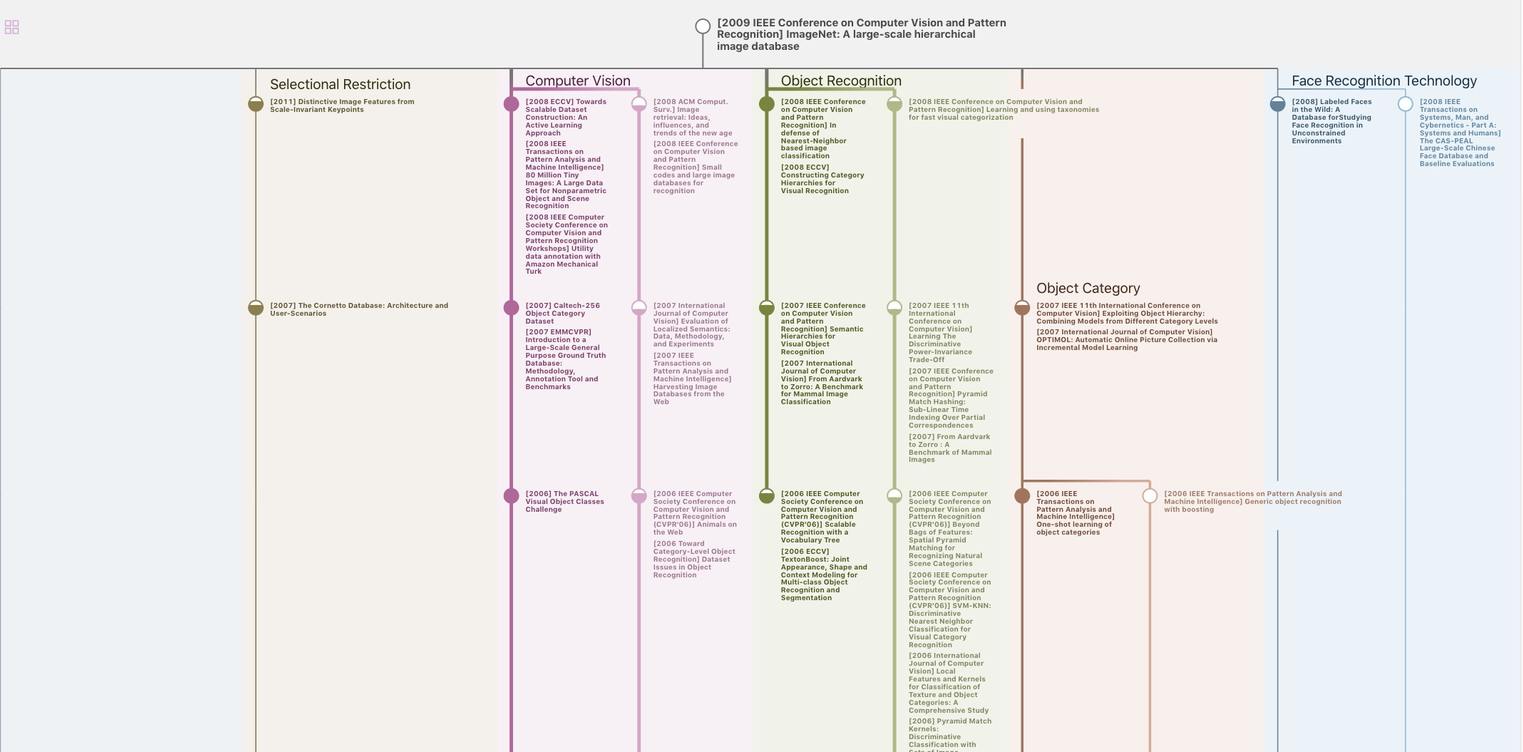
生成溯源树,研究论文发展脉络
Chat Paper
正在生成论文摘要