GNN-PT: Enhanced Prediction of Compound-protein Interactions by Integrating Protein Transformer
arXiv (Cornell University)(2020)
摘要
The prediction of protein interactions (CPIs) is crucial for the in-silico screening step in drug discovery. Recently, many end-to-end representation learning methods using deep neural networks have achieved significantly better performance than traditional machine learning algorithms. Much effort has focused on the compound representation or the information extraction from the compound-protein interaction to improve the model capability by taking the advantage of the neural attention mechanism. However, previous studies have paid little attention to representing the protein sequences, in which the long-range interactions of residue pairs are essential for characterizing the structural properties arising from the protein folding. We incorporate the self-attention mechanism into the protein representation module for CPI modeling, which aims at capturing the long-range interaction information within proteins. The proposed module concerning protein representation, called Protein Transformer, with an integration with an existing CPI model, has shown a significant improvement in the prediction performance when compared with several existing CPI models.
更多查看译文
关键词
enhanced prediction,compound-protein
AI 理解论文
溯源树
样例
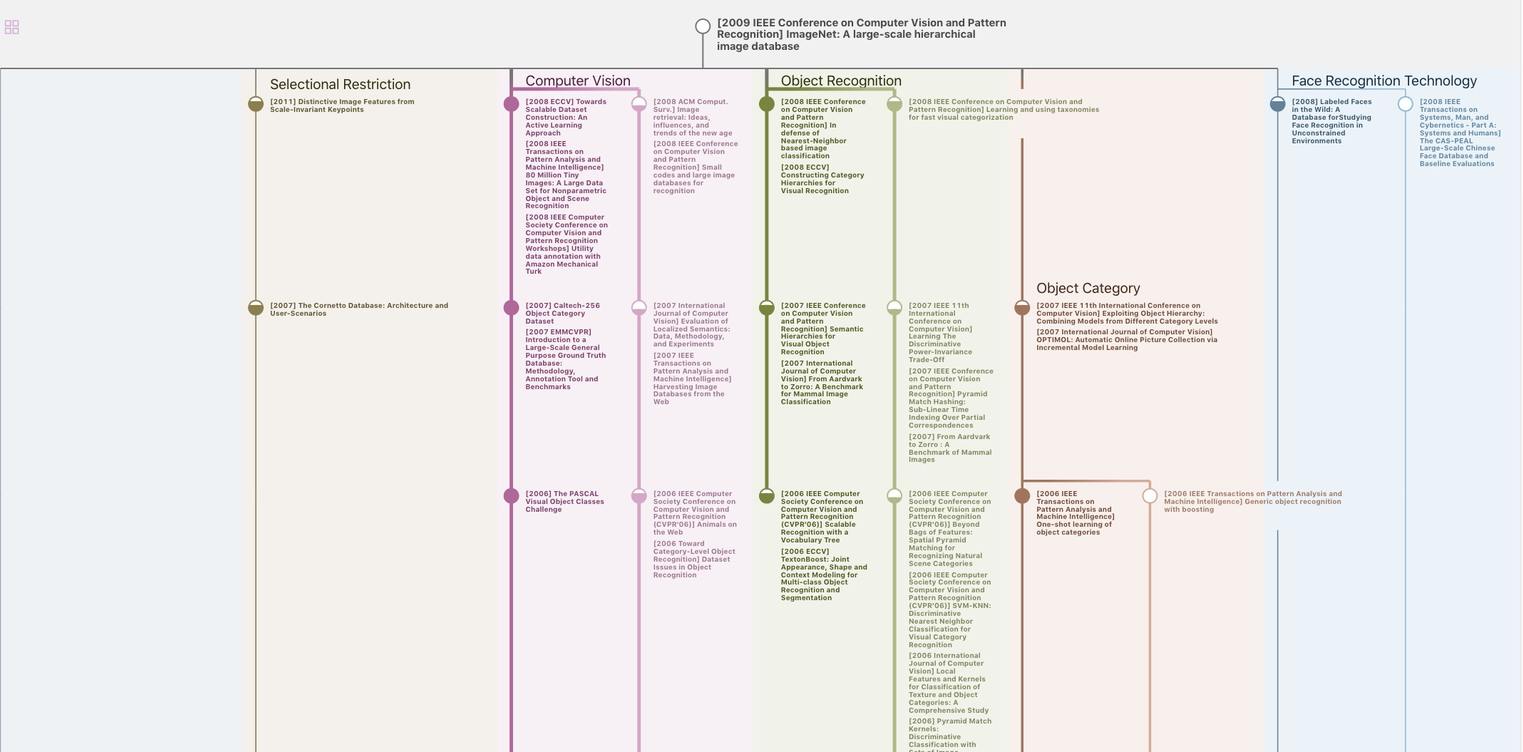
生成溯源树,研究论文发展脉络
Chat Paper
正在生成论文摘要