Robotic Arm Control and Task Training through Deep Reinforcement Learning
arXiv (Cornell University)(2020)
摘要
This paper proposes a detailed and extensive comparison of the Trust Region Policy Optimization and DeepQ-Network with Normalized Advantage Functions with respect to other state of the art algorithms, namely Deep Deterministic Policy Gradient and Vanilla Policy Gradient. Comparisons demonstrate that the former have better performances then the latter when asking robotic arms to accomplish manipulation tasks such as reaching a random target pose and pick &placing an object. Both simulated and real-world experiments are provided. Simulation lets us show the procedures that we adopted to precisely estimate the algorithms hyper-parameters and to correctly design good policies. Real-world experiments let show that our polices, if correctly trained on simulation, can be transferred and executed in a real environment with almost no changes.
更多查看译文
关键词
deep reinforcement,task training,arm,learning
AI 理解论文
溯源树
样例
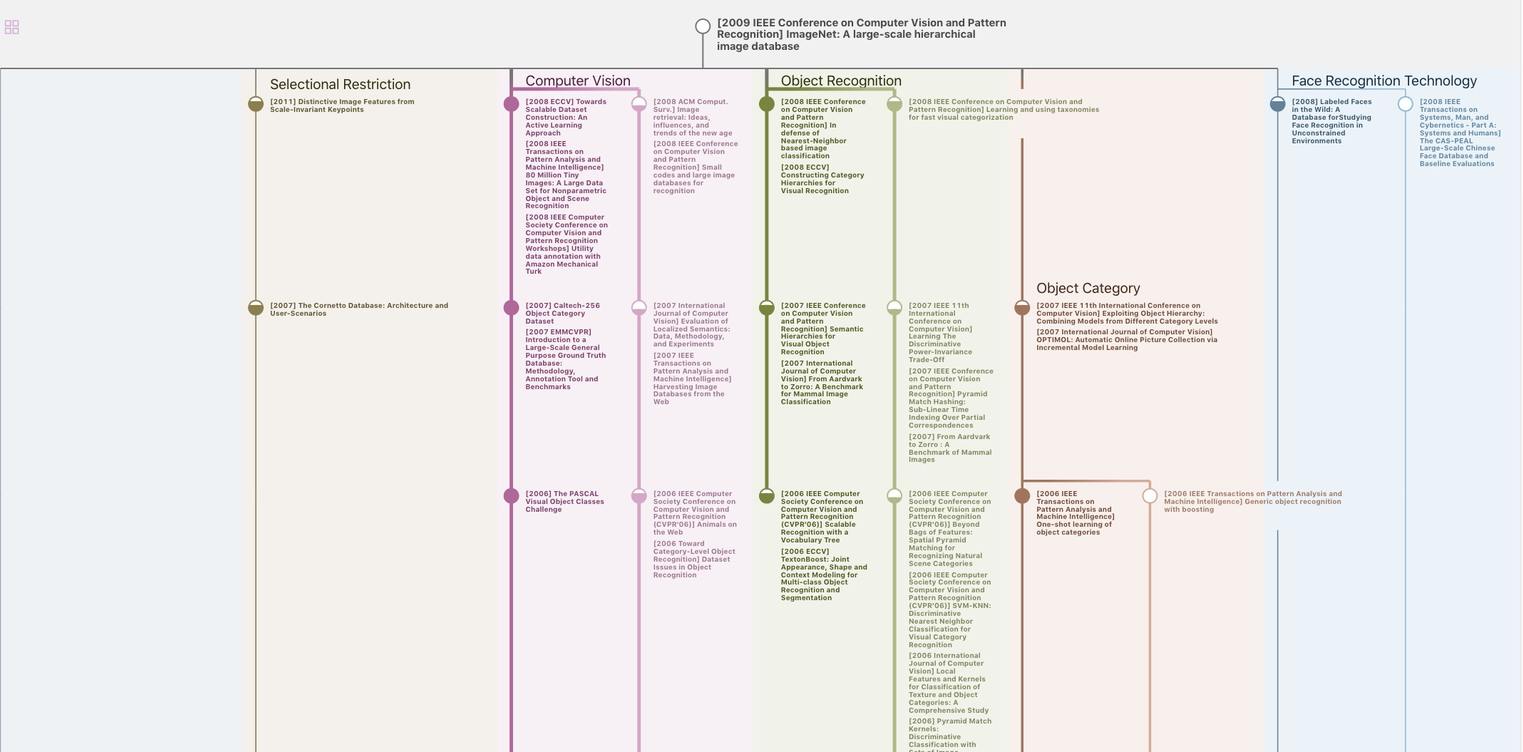
生成溯源树,研究论文发展脉络
Chat Paper
正在生成论文摘要