AutoML for Multilayer Perceptron and FPGA Co-design
arXiv (Cornell University)(2020)
摘要
State-of-the-art Neural Network Architectures (NNAs) are challenging to design and implement efficiently in hardware. In the past couple of years, this has led to an explosion in research and development of automatic Neural Architecture Search (NAS) tools. AutomML tools are now used to achieve state of the art NNA designs and attempt to optimize for hardware usage and design. Much of the recent research in the auto-design of NNAs has focused on convolution networks and image recognition, ignoring the fact that a significant part of the workload in data centers is general-purpose deep neural networks. In this work, we develop and test a general multilayer perceptron (MLP) flow that can take arbitrary datasets as input and automatically produce optimized NNAs and hardware designs. We test the flow on six benchmarks. Our results show we exceed the performance of currently published MLP accuracy results and are competitive with non-MLP based results. We compare general and common GPU architectures with our scalable FPGA design and show we can achieve higher efficiency and higher throughput (outputs per second) for the majority of datasets. Further insights into the design space for both accurate networks and high performing hardware shows the power of co-design by correlating accuracy versus throughput, network size versus accuracy, and scaling to high-performance devices.
更多查看译文
关键词
multilayer perceptron,fpga,automl,co-design
AI 理解论文
溯源树
样例
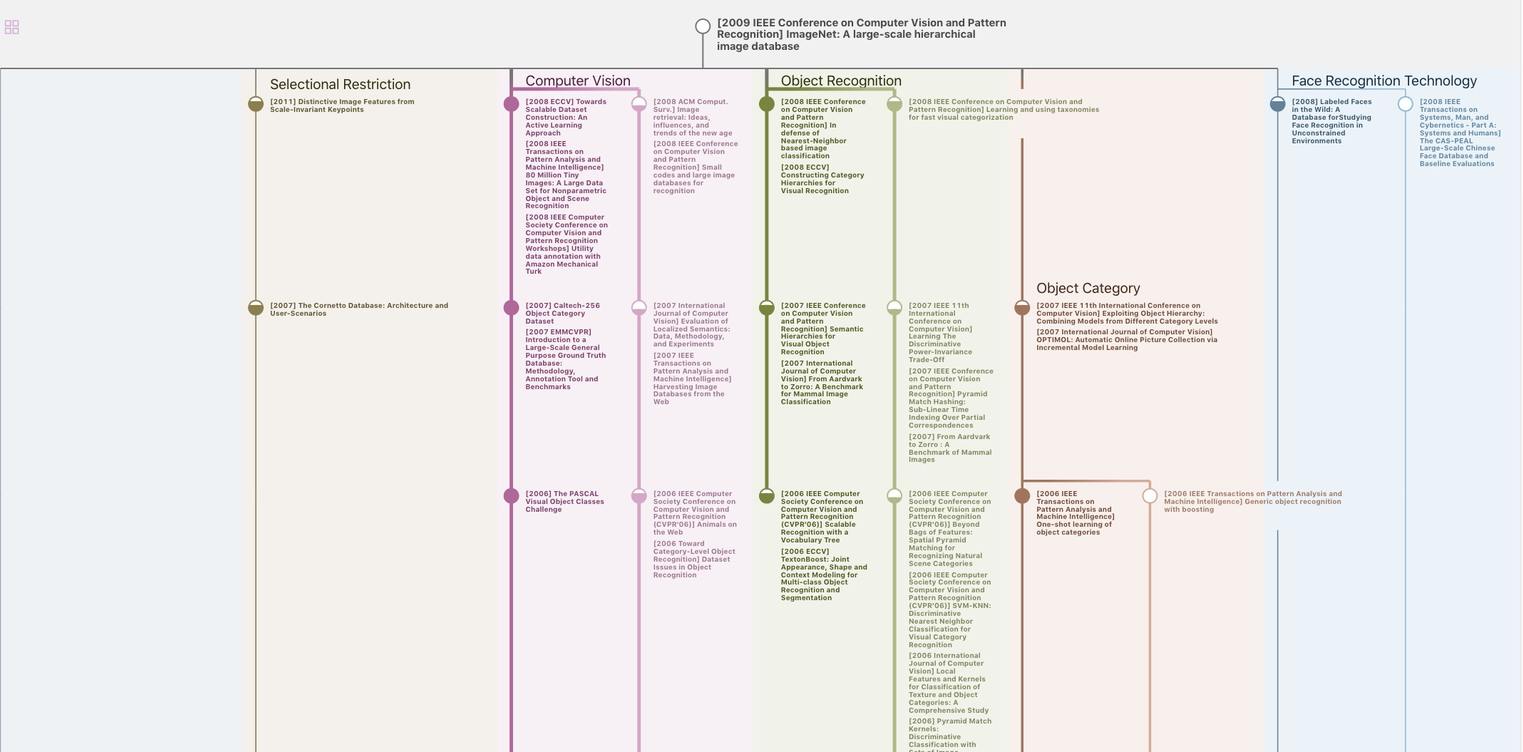
生成溯源树,研究论文发展脉络
Chat Paper
正在生成论文摘要