On the Explanation of Machine Learning Predictions in Clinical Gait Analysis
arXiv (Cornell University)(2020)
摘要
Machine learning (ML) is increasingly used to support decision-making in the healthcare sector. While ML approaches provide promising results with regard to their classification performance, most share a central limitation, namely their black-box character. Motivated by the interest to understand the functioning of ML models, methods from the field of Explainable Artificial Intelligence (XAI) have recently become important. This article investigates the usefulness of XAI methods in clinical gait classification. For this purpose, predictions of state-of-the-art classification methods are explained with an established XAI method, i.e., Layer-wise Relevance Propagation (LRP). We propose to evaluate the obtained explanations with two complementary approaches: a statistical analysis of the underlying data using Statistical Parametric Mapping and a qualitative evaluation by a clinical expert. A gait dataset comprising ground reaction force measurements from 132 patients with different lower-body gait disorders and 62 healthy controls is utilized. We investigate several gait classification tasks, employ multiple classification methods, and analyze the impact of data normalization and different signal components for classification performance and explanation quality. Our experiments show that explanations obtained by LRP exhibit promising statistical properties concerning inter-class discriminativity and are also in line with clinically relevant biomechanical gait characteristics.
更多查看译文
关键词
machine learning predictions,gait,machine learning
AI 理解论文
溯源树
样例
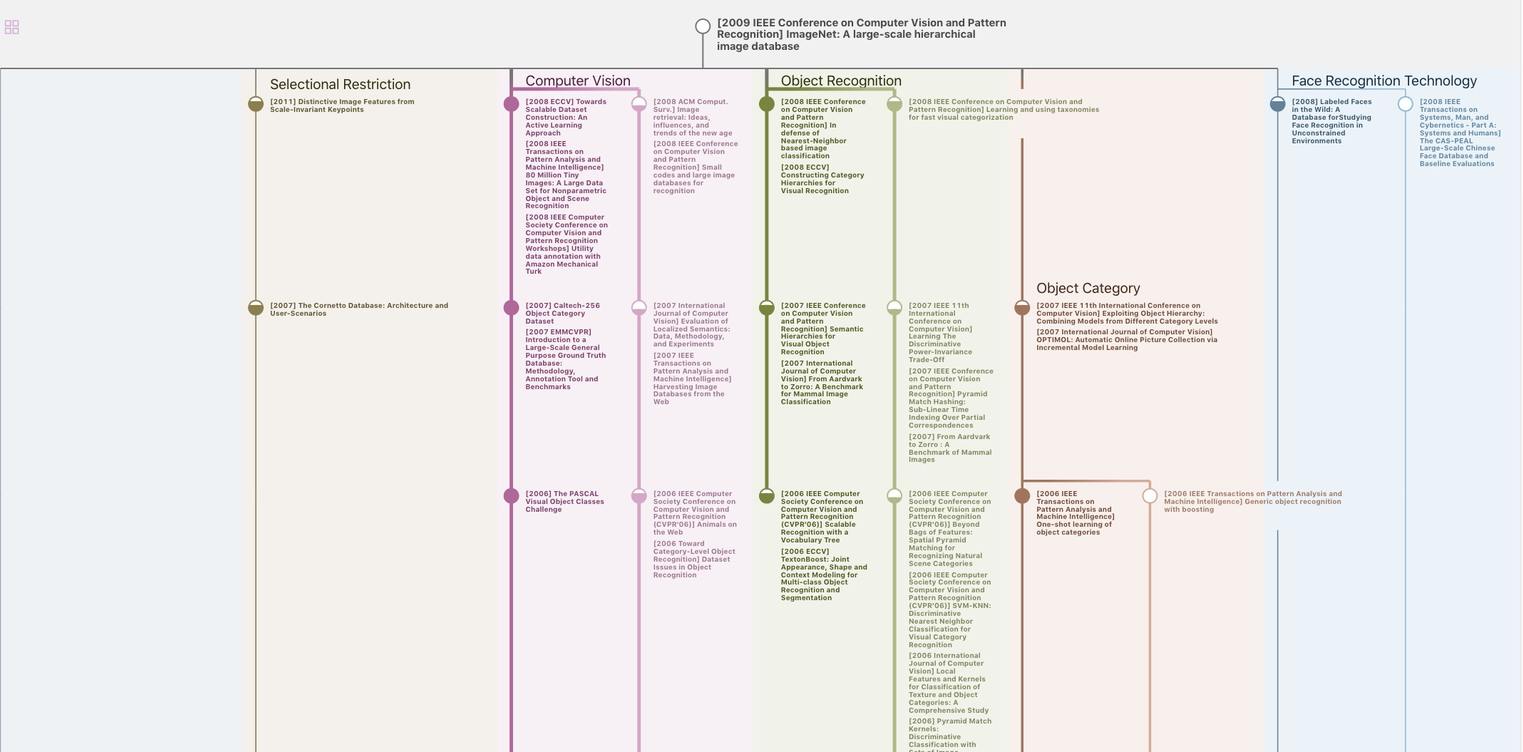
生成溯源树,研究论文发展脉络
Chat Paper
正在生成论文摘要