Deep Reinforcement Learning for Resource Constrained Multiclass Scheduling in Wireless Networks
arXiv (Cornell University)(2020)
摘要
The problem of resource constrained scheduling in a dynamic and heterogeneous wireless setting is considered here. In our setup, the available limited bandwidth resources are allocated in order to serve randomly arriving service demands, which in turn belong to different classes in terms of payload data requirement, delay tolerance, and importance/priority. In addition to heterogeneous traffic, another major challenge stems from random service rates due to time-varying wireless communication channels. Various approaches for scheduling and resource allocation can be used, ranging from simple greedy heuristics and constrained optimization to combinatorics. Those methods are tailored to specific network or application configuration and are usually suboptimal. To this purpose, we resort to deep reinforcement learning (DRL) and propose a distributional Deep Deterministic Policy Gradient (DDPG) algorithm combined with Deep Sets to tackle the aforementioned problem. Furthermore, we present a novel way to use a Dueling Network, which leads to further performance improvement. Our proposed algorithm is tested on both synthetic and real data, showing consistent gains against state-of-the-art conventional methods from combinatorics, optimization, and scheduling metrics.
更多查看译文
关键词
resource constrained multiclass,scheduling,wireless networks,reinforcement learning
AI 理解论文
溯源树
样例
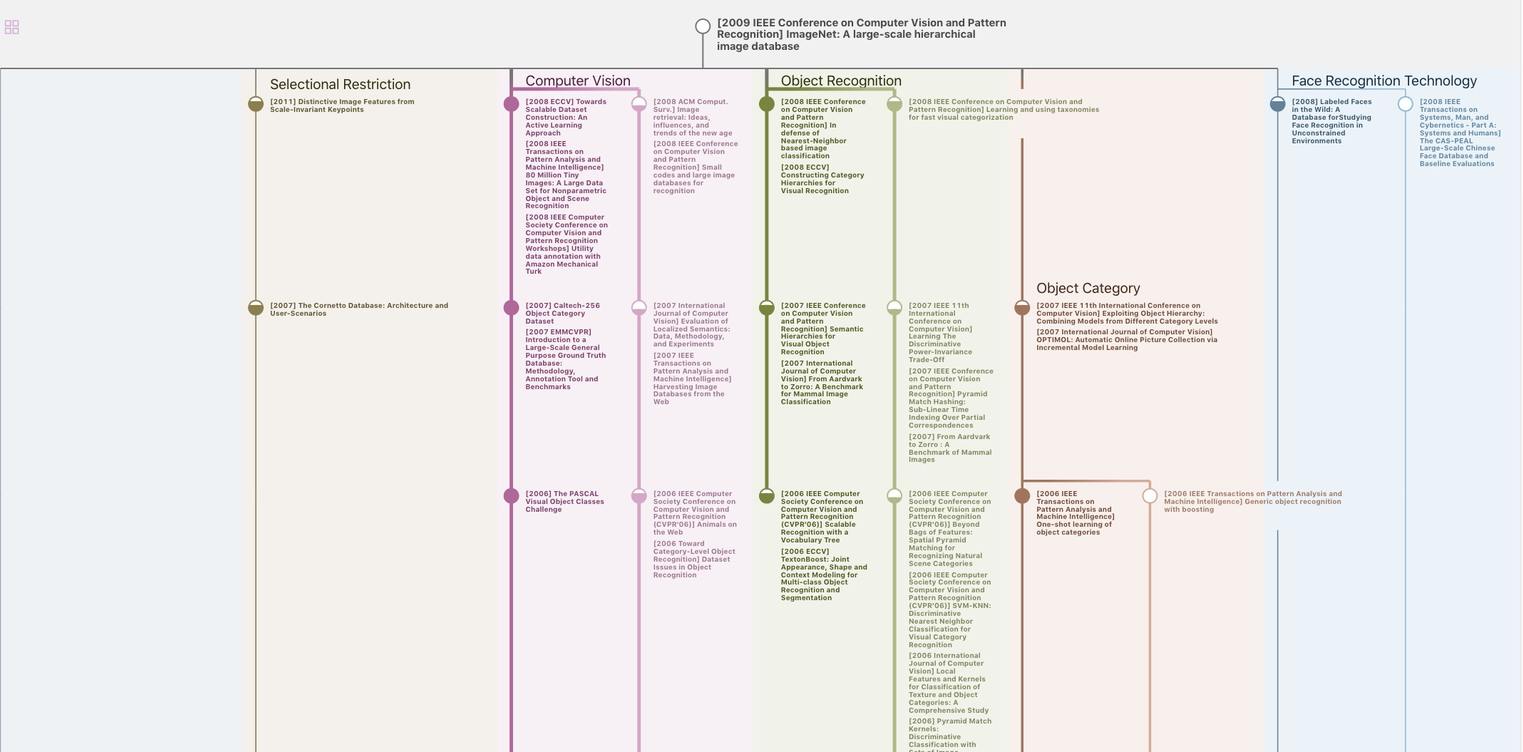
生成溯源树,研究论文发展脉络
Chat Paper
正在生成论文摘要