A Deep Learning Approach for Recognizing Activity of Daily Living (ADL) for Senior Care: Exploiting Interaction Dependency and Temporal Patterns
Social Science Research Network(2020)
摘要
Ensuring the health and safety of independent-living senior citizens is a growing societal concern. Activity of Daily Living (ADL) is a common approach to monitor these citizens’ self-care ability and disease progression. However, prevailing sensor-based ADL monitoring systems primarily rely on wearable motion sensors, capture insufficient information for accurate ADL recognition, and do not provide a comprehensive understanding of ADLs at different granularities. Current healthcare IS and mobile analytics research focuses on studying the system, device, and provided services, and needs an end-to-end solution to comprehensively recognize ADLs from mobile sensor data. This study adopts the design science paradigm and employs advanced deep learning algorithms to develop a novel hierarchical, multi-phase ADL recognition framework to model ADLs with different granularities. A novel 2D Interaction Kernel for convolutional neural networks is proposed to leverage interactions between human and object motion sensors. We rigorously evaluate each proposed module and the entire framework against state-of-the-art benchmarks (e.g., Support Vector Machines, DeepConvLSTM, Hidden Markov Models, and Topic-modeling-based ADLR) on two real-life motion sensor datasets that consist of ADLs at varying granularities: Opportunity and INTER. Results and a case study demonstrate that our framework can recognize ADLs on different levels more accurately. We discuss how stakeholders can further benefit from our proposed framework. Beyond demonstrating the practical utility, we discuss contributions to the IS knowledge base for future design-science-based cybersecurity, healthcare, and mobile analytics applications.
更多查看译文
关键词
daily living,senior care,activity,deep learning
AI 理解论文
溯源树
样例
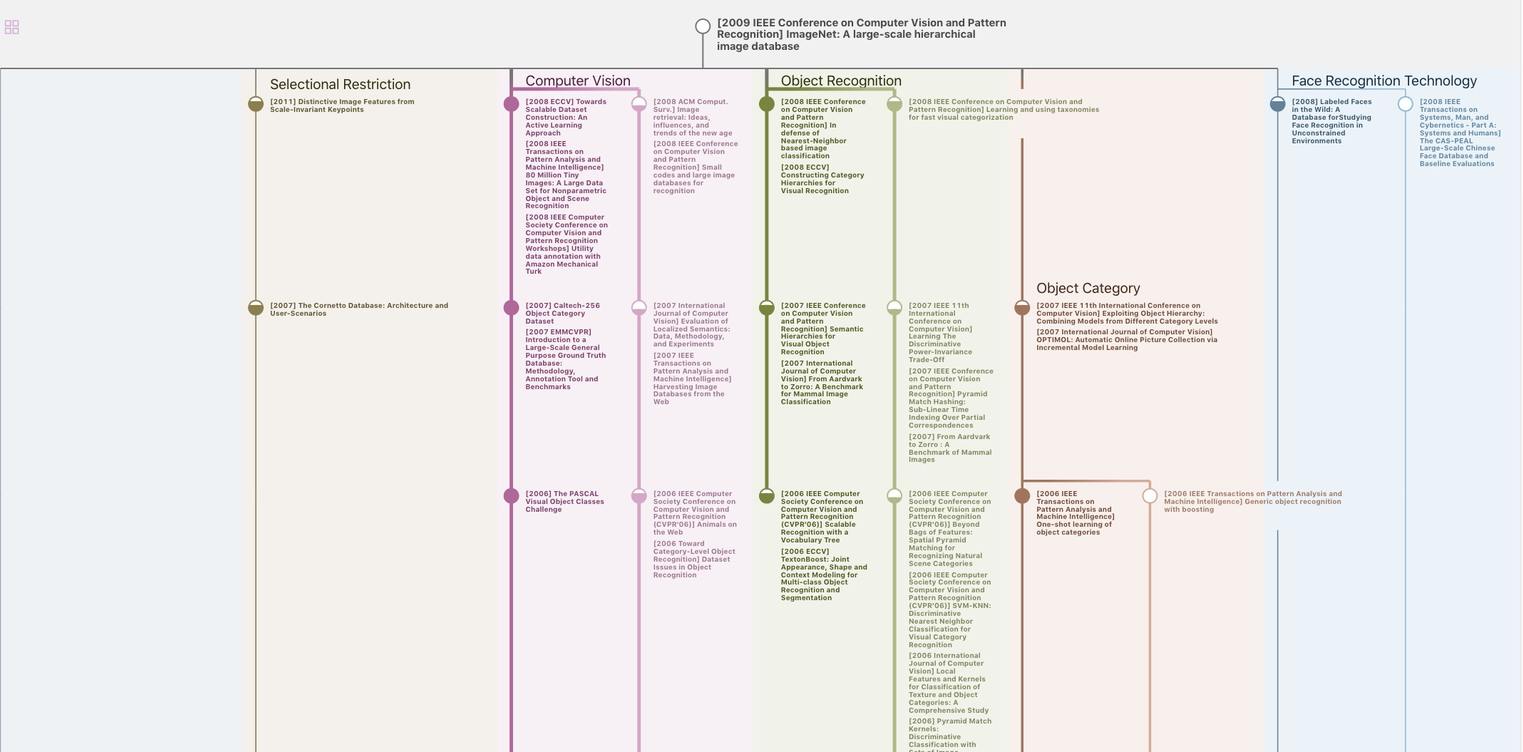
生成溯源树,研究论文发展脉络
Chat Paper
正在生成论文摘要