DiffusionRet: Diffusion-Enhanced Generative Retriever using Constrained Decoding.
EMNLP 2023(2023)
摘要
Generative retrieval, which maps from a query to its relevant document identifiers (docids), has recently emerged as a new information retrieval (IR) paradigm, however, having suffered from 1) the $\textit{lack of the intermediate reasoning step}$, caused by the manner of merely using a query to perform the hierarchical classification, and 2) the $\textit{pretrain-finetune discrepancy}$, which comes from the use of the artificial symbols of docids. To address these limitations, we propose the novel approach of using the document generation from a query as an intermediate step before the retrieval, thus presenting $\underline{diffusion}$-enhanced generative $\underline{ret}$rieval ($\textbf{DiffusionRet}$), which consists of two processing steps: 1) the $\textit{diffusion-based document generation}$, which employs the sequence-to-sequence diffusion model to produce a pseudo document sample from a query, being expected to semantically close to a relevant document; 2) $\textit{N-gram-based generative retrieval}$, which use another sequence-to-sequence model to generate n-grams that appear in the collection index for linking a generated sample to an original document. Experiment results on MS MARCO and Natural Questions dataset show that the proposed DiffusionRet significantly outperforms all the existing generative retrieval methods and leads to the state-of-the-art performances, even with much smaller number of parameters.
更多查看译文
AI 理解论文
溯源树
样例
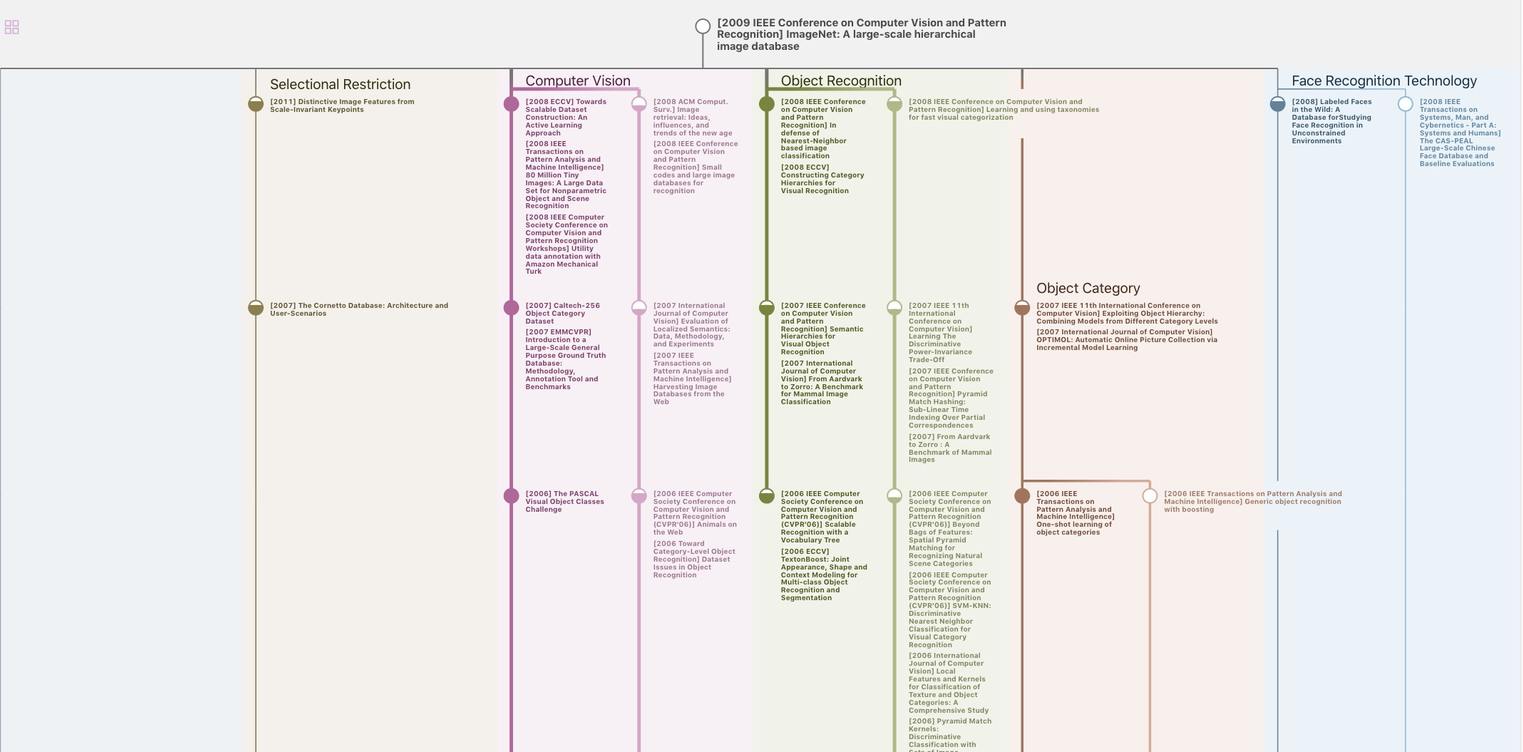
生成溯源树,研究论文发展脉络
Chat Paper
正在生成论文摘要