Parameter Efficient Multi-task Fine-tuning by Learning to Transfer Token-wise Prompts.
EMNLP 2023(2023)
摘要
Prompt tuning has been proven to be successful on various tasks by incorporating a small number of trainable parameters while freezing large pre-trained language models (PLMs).
However, it is still unsettled how to generate more proper prompts for any individual examples and how to extend prompt tuning to multi-task learning scenarios by leveraging cross-task features.
To address these challenges, we propose a token-wise prompt tuning (TPT), in which a bank of finer-grained soft prompt tokens is built for multi-task learning by memory network.
The tokens are retrieved from the bank against an input example and assembled to an instance-dependent prompt. Extensive experimental results on $14$ datasets demonstrated that the models enhanced by our TPT performed far better than full parameter fine-tuned models and achieved state-of-the-art by tuning only $0.035\%$ parameters.
更多查看译文
AI 理解论文
溯源树
样例
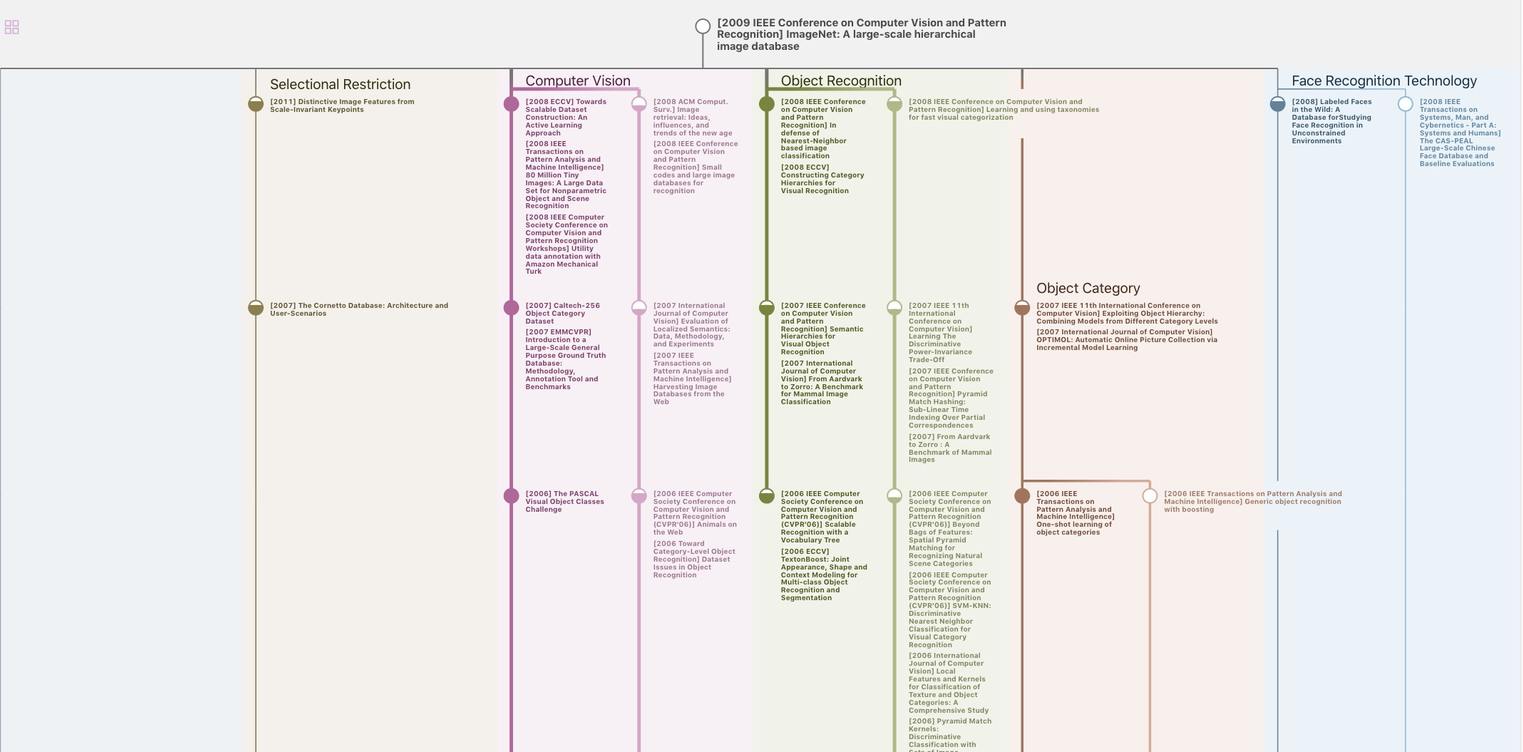
生成溯源树,研究论文发展脉络
Chat Paper
正在生成论文摘要