Zero-shot Topical Text Classification with LLMs - an Experimental Study.
EMNLP 2023(2023)
摘要
Topical Text Classification (TTC) is an ancient, yet timely research area in natural language processing, with many practical applications. The recent dramatic advancements in large LMs raise the question of how well these models can perform in this task in a zero-shot scenario.
Here, we share a first comprehensive study, comparing the zero-shot performance of a variety of LMs over TTC23, a large benchmark collection of 23 publicly available TTC datasets, covering a wide range of domains and styles. In addition, we leverage this new TTC benchmark to create LMs that are specialized in TTC, by fine-tuning these LMs over a subset of the datasets and evaluating their performance over the remaining, held-out datasets. We show that the TTC-specialized LMs obtain the top performance on our benchmark, by a significant margin. Our code and model are made available for the community. We hope that the results presented in this work will serve as a useful guide for practitioners interested in topical text classification.
更多查看译文
AI 理解论文
溯源树
样例
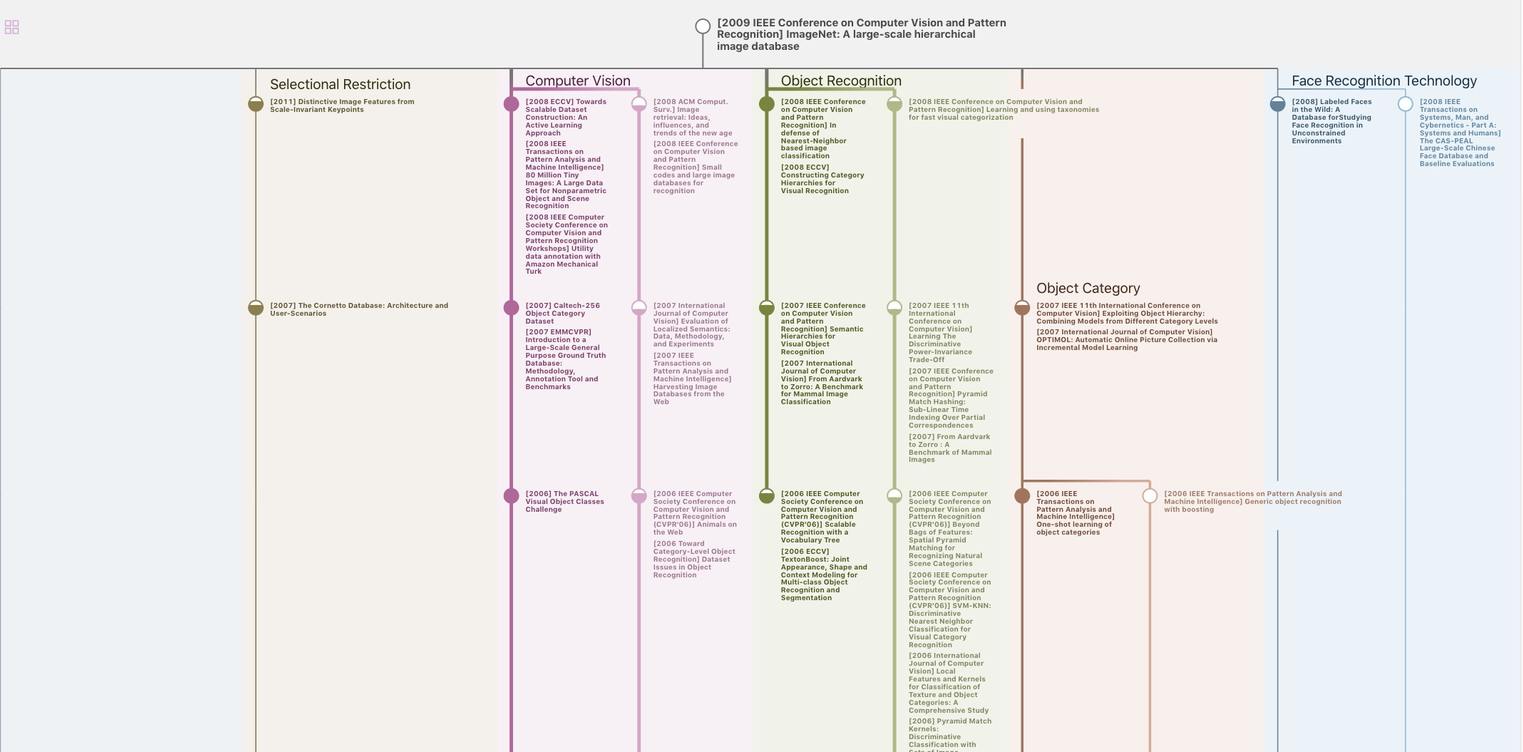
生成溯源树,研究论文发展脉络
Chat Paper
正在生成论文摘要