Dimension Independent Generalization Error by Stochastic Gradient Descent
arXiv (Cornell University)(2020)
摘要
One classical canon of statistics is that large models are prone to overfitting, and model selection procedures are necessary for high dimensional data. However, many overparameterized models, such as neural networks, perform very well in practice, although they are often trained with simple online methods and regularization. The empirical success of overparameterized models, which is often known as benign overfitting, motivates us to have a new look at the statistical generalization theory for online optimization. In particular, we present a general theory on the generalization error of stochastic gradient descent (SGD) solutions for both convex and locally convex loss functions. We further discuss data and model conditions that lead to a ``low effective dimension. Under these conditions, we show that the generalization error either does not depend on the ambient dimension $p$ or depends on $p$ via a poly-logarithmic factor. We also demonstrate that in several widely used statistical models, the ``low effective dimension'' arises naturally in overparameterized settings. The studied statistical applications include both convex models such as linear regression and logistic regression and non-convex models such as $M$-estimator and two-layer neural networks.
更多查看译文
关键词
gradient,dimension
AI 理解论文
溯源树
样例
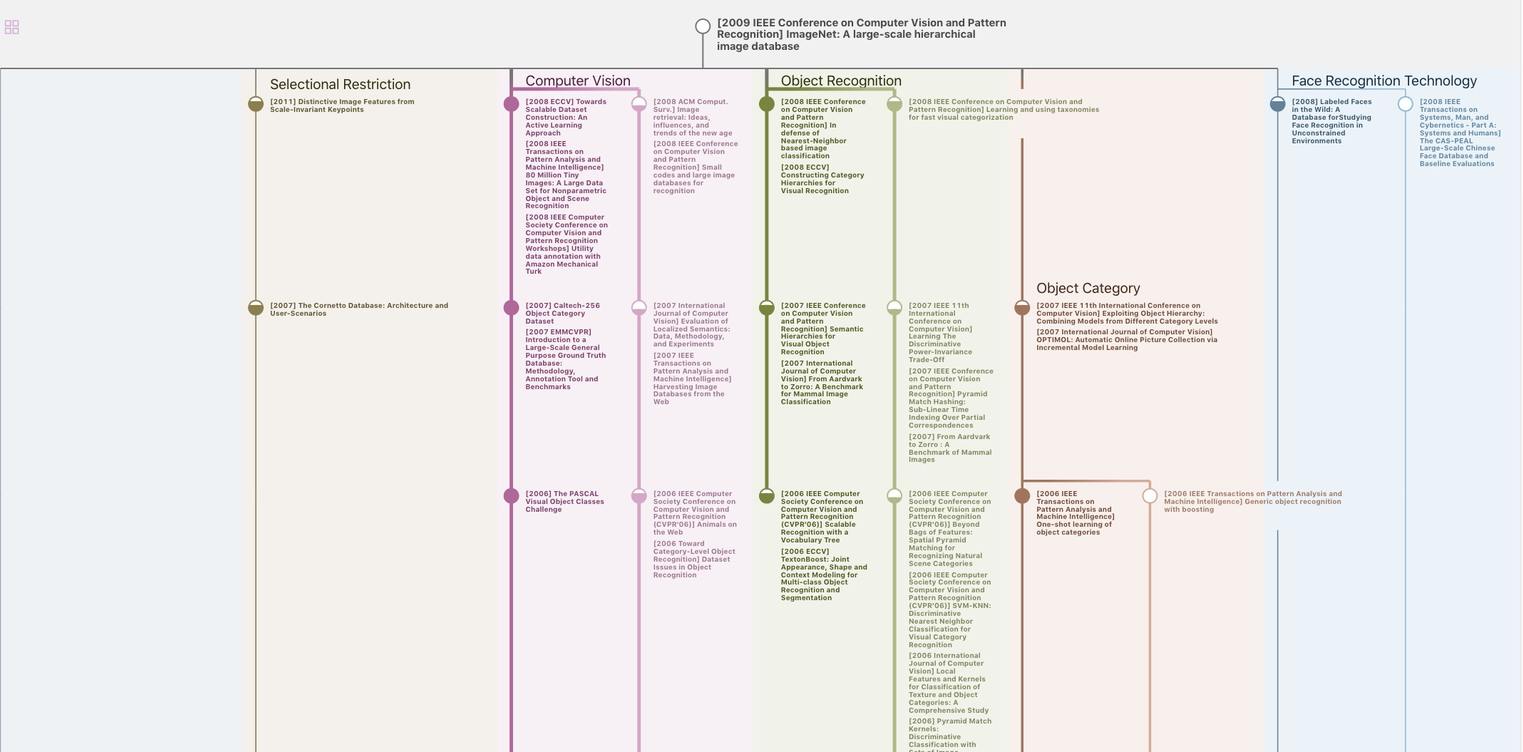
生成溯源树,研究论文发展脉络
Chat Paper
正在生成论文摘要