Enhancing security in X-ray baggage scans: A contour-driven learning approach for abnormality classification and instance segmentation
ENGINEERING APPLICATIONS OF ARTIFICIAL INTELLIGENCE(2024)
摘要
The task of automatically identifying hazardous items in luggage through X-ray scans is highly crucial, yet immensely complex. This method presents an improvement over the traditional, labor-intensive, timeconsuming, and error-prone manual evaluation of X-ray images. In the present day, an enormous volume of luggage is continually passing through airports, seaports, and land ports, making these advancements exceedingly vital. Nevertheless, numerous existing solutions grapple with the issue of data imbalance, as the frequency of dangerous items is relatively low, adversely affecting the efficiency of networks. In this study, we introduce a solution that merges a contour-driven learned model with a unique loss function known as Balanced Affinity Loss. This function equitably distributes the focus of training models towards less represented items. We tested the proposed system using three public luggage X-ray datasets, where it exceeded the performance of the most advanced methods by 34.1%, 8.64%, and 10.04% in terms of intersection-over-union for instance segmentation. Likewise, the introduced system registered improvements of 9.11% and 3.66% for abnormality classification.
更多查看译文
关键词
Affinity Loss,Baggage X-ray Scans,Contour-driven learned model,Instance Segmentation,Abnormality classification,Data imbalance
AI 理解论文
溯源树
样例
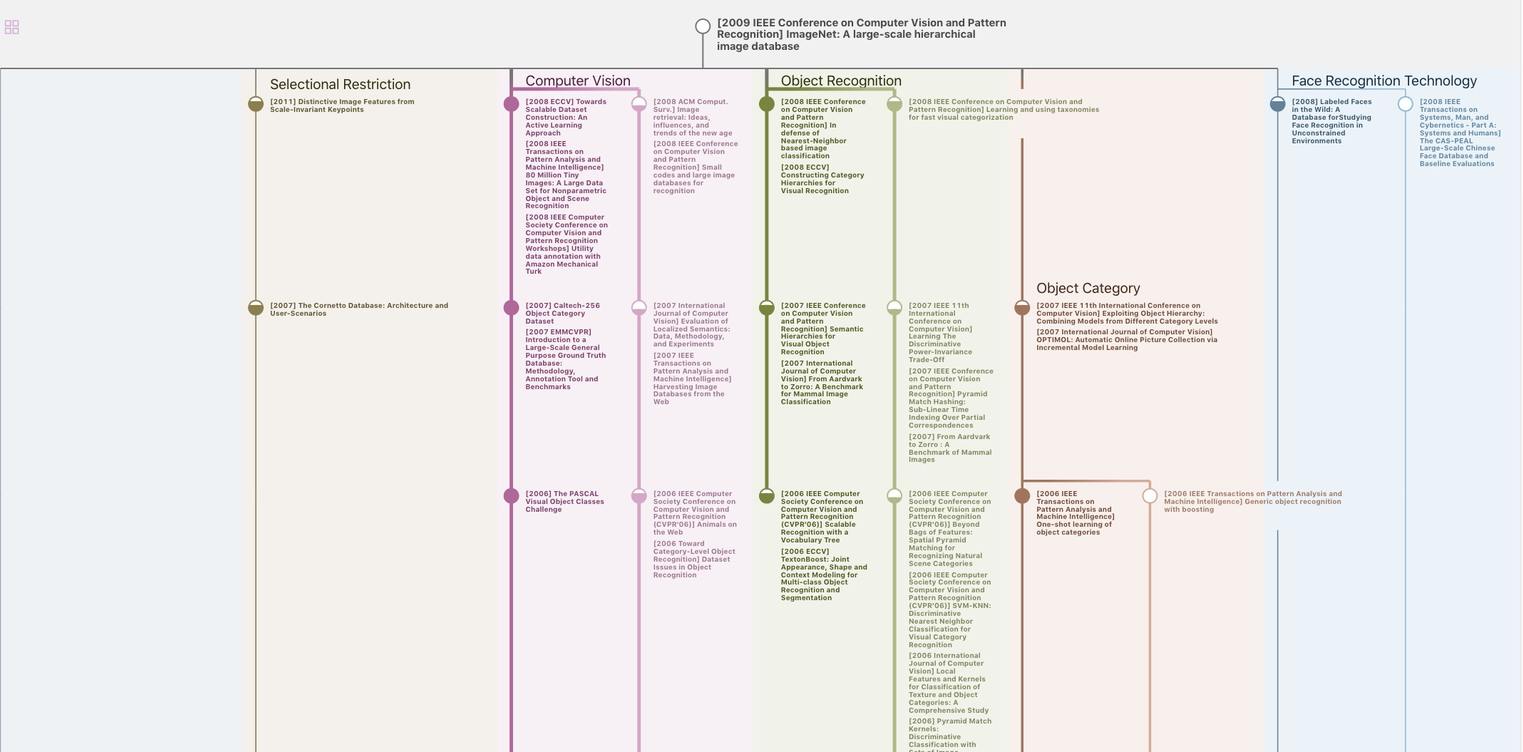
生成溯源树,研究论文发展脉络
Chat Paper
正在生成论文摘要