Collinear datasets augmentation using Procrustes validation sets
CoRR(2023)
摘要
In this paper, we propose a new method for the augmentation of numeric and
mixed datasets. The method generates additional data points by utilizing
cross-validation resampling and latent variable modeling. It is particularly
efficient for datasets with moderate to high degrees of collinearity, as it
directly utilizes this property for generation. The method is simple, fast, and
has very few parameters, which, as shown in the paper, do not require specific
tuning. It has been tested on several real datasets; here, we report detailed
results for two cases, prediction of protein in minced meat based on near
infrared spectra (fully numeric data with high degree of collinearity) and
discrimination of patients referred for coronary angiography (mixed data, with
both numeric and categorical variables, and moderate collinearity). In both
cases, artificial neural networks were employed for developing the regression
and the discrimination models. The results show a clear improvement in the
performance of the models; thus for the prediction of meat protein, fitting the
model to the augmented data resulted in a reduction in the root mean squared
error computed for the independent test set by 1.5 to 3 times.
更多查看译文
AI 理解论文
溯源树
样例
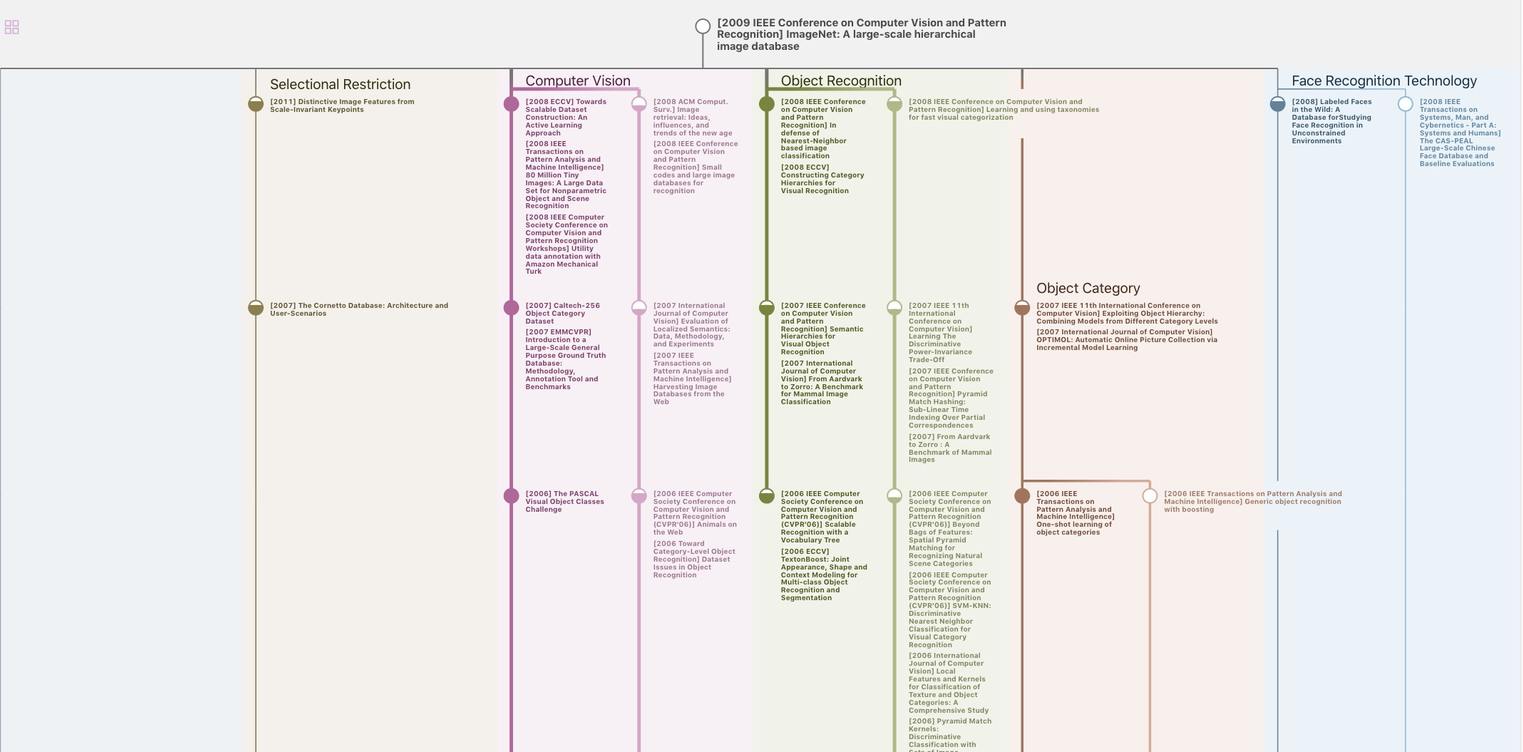
生成溯源树,研究论文发展脉络
Chat Paper
正在生成论文摘要