DiffCMR: Fast Cardiac MRI Reconstruction with Diffusion Probabilistic Models
CoRR(2023)
摘要
Performing magnetic resonance imaging (MRI) reconstruction from under-sampled
k-space data can accelerate the procedure to acquire MRI scans and reduce
patients' discomfort. The reconstruction problem is usually formulated as a
denoising task that removes the noise in under-sampled MRI image slices.
Although previous GAN-based methods have achieved good performance in image
denoising, they are difficult to train and require careful tuning of
hyperparameters. In this paper, we propose a novel MRI denoising framework
DiffCMR by leveraging conditional denoising diffusion probabilistic models.
Specifically, DiffCMR perceives conditioning signals from the under-sampled MRI
image slice and generates its corresponding fully-sampled MRI image slice.
During inference, we adopt a multi-round ensembling strategy to stabilize the
performance. We validate DiffCMR with cine reconstruction and T1/T2 mapping
tasks on MICCAI 2023 Cardiac MRI Reconstruction Challenge (CMRxRecon) dataset.
Results show that our method achieves state-of-the-art performance, exceeding
previous methods by a significant margin. Code is available at
https://github.com/xmed-lab/DiffCMR.
更多查看译文
AI 理解论文
溯源树
样例
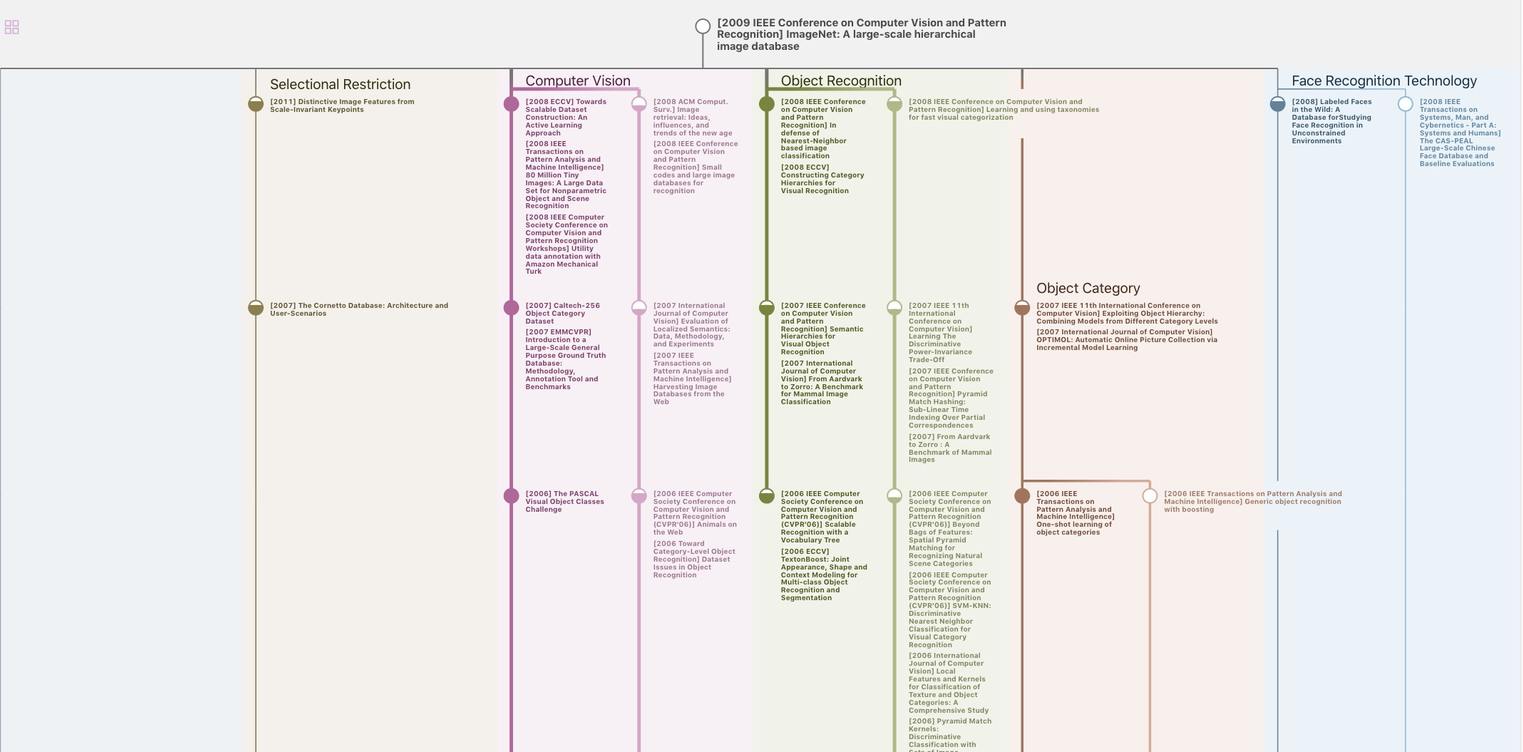
生成溯源树,研究论文发展脉络
Chat Paper
正在生成论文摘要