SPNet: An RGB-D Sequence Progressive Network for Road Semantic Segmentation
2023 IEEE 25TH INTERNATIONAL WORKSHOP ON MULTIMEDIA SIGNAL PROCESSING, MMSP(2023)
摘要
Road semantic segmentation is an essential component of autonomous driving and blind navigation. Although many excellent RGB-based road semantic segmentation algorithms have been proposed, these methods may not detect correctly due to the lack of geometric information. Recently, RGB-D road semantic segmentation methods attract more research attention. However, the existing RGB-D methods ignore the impact of unknown noise in sensors. To solve this problem, we propose an RGB-D Sequence Progressive Network (SPNet) for road semantic segmentation. Specifically, we first propose a sequence-based RGB-D feature extractor to alleviate the effect of noise. Then, We propose a multi-modal feature fusion (MMFF) module to enhance the feature representation of multi-modal data by further alleviating the effect of noise. Finally, we propose a semantic flow prediction (SFP) module that aims to align the multi-modal features in the decoder. Extensive experiments are conducted on several challenging datasets, including KITTI and GMRP. Our method achieves an F-score of 97.21% on the KITTI official leaderboard and ranked third in the official leaderboard.
更多查看译文
关键词
Road semantic segmentation,RGB-D,Feature Extractor
AI 理解论文
溯源树
样例
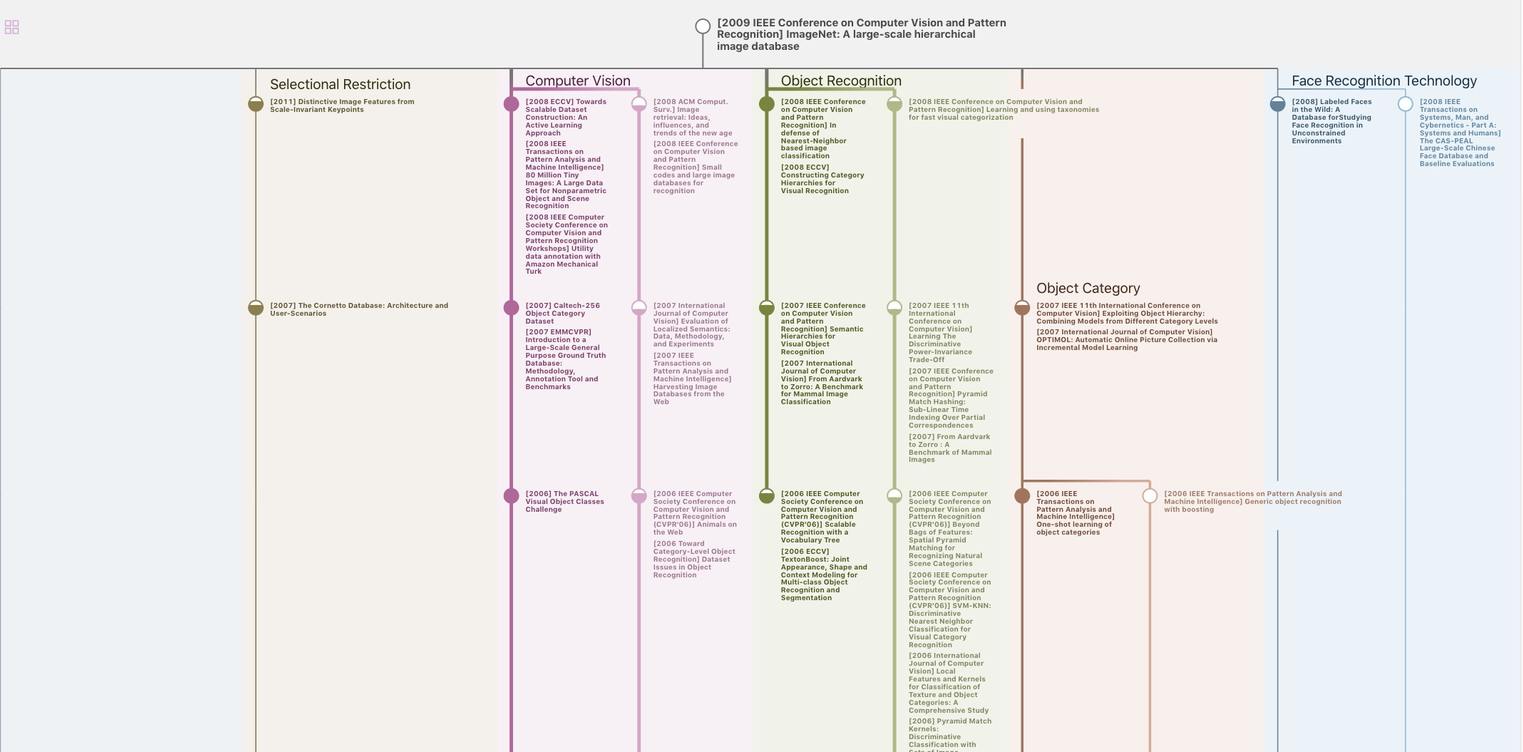
生成溯源树,研究论文发展脉络
Chat Paper
正在生成论文摘要