A novel vision transformer network for rolling bearing remaining useful life prediction
MEASUREMENT SCIENCE AND TECHNOLOGY(2024)
摘要
The accurate predictions of remaining useful life (RUL) have become a key and extremely challenging problem. Due to the limitations of the classical convolutional neural network and recurrent neural network structure, the attention mechanism has been introduced to improve feature representation of the long-term bearing degradation data. Transformer network based on attention mechanism is successfully applied in many fields and recognized as an excellent creation for deep learning models. In this paper, a novel lightweight mobile vision transformer (MobileViT) architecture based on deep networks is proposed for the RUL predictions. This new network is named prognostics separable vision Transformer (ProgSViT), which combines the separable convolution and MobileViT. In ProgSViT network, the separable convolutions are first constructed for extracting local feature from the input vibration signal, and the new vision transformer architecture is proposed to learn the global feature representations. In improved MobileViT model, the loss function is optimized, and a new training strategy is provided. Finally, the obtained features are input to the global average pool layers and the full connection layers to perform RUL estimation. Experiment results present the proposed ProgSViT network surpasses the other models in RUL predicting, which possesses higher precision and computational efficiency.
更多查看译文
关键词
remaining useful life (RUL),prediction,prognostics separable vision transformer (ProgSViT),deep network,rolling bearing
AI 理解论文
溯源树
样例
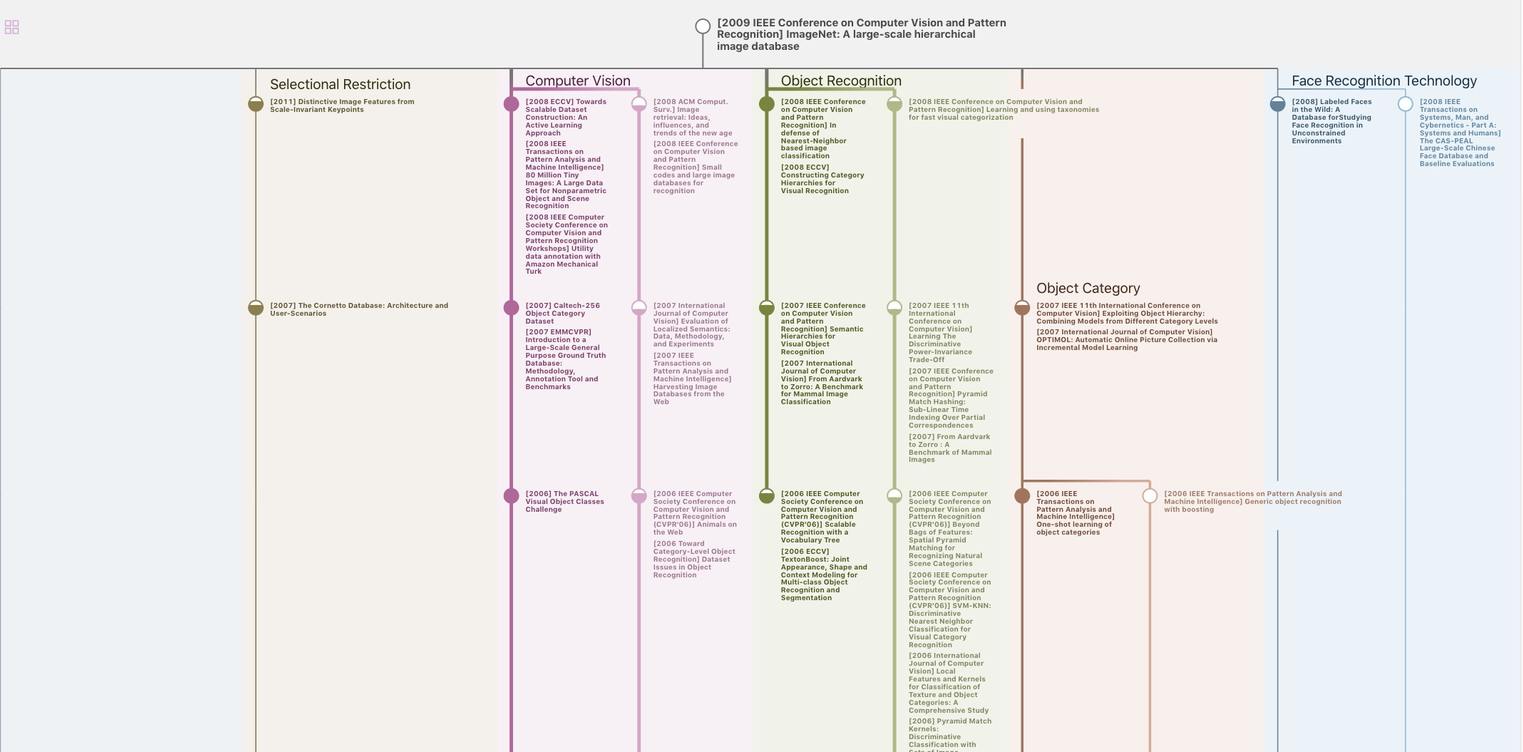
生成溯源树,研究论文发展脉络
Chat Paper
正在生成论文摘要