Heteroscedastic Bayesian optimization using generalized product of experts
JOURNAL OF GLOBAL OPTIMIZATION(2023)
摘要
In many real world optimization problems observations are corrupted by a heteroscedastic noise, which depends on the input location. Bayesian optimization (BO) is an efficient approach for global optimization of black-box functions, but the performance of using a Gaussian process (GP) model can degrade with changing levels of noise due to a homoscedastic noise assumption. However, a generalized product of experts (GPOE) model allows us to build independent GP experts on the subsets of observations with individual set of hyperparameters, which is flexible enough to capture the changing levels of noise. In this paper we propose a heteroscedastic Bayesian optimization algorithm by combining the GPOE model with two modifications of existing acquisition functions, which are capable of representing and penalizing heteroscedastic noise across the input space. We compare and evaluate the performance of GPOE based BO (GPOEBO) model on 6 synthetic global optimization functions corrupted with the heteroscedastic noise as well as on two real-world scientific datasets. The results show that GPOEBO is able to improve the accuracy compared to other methods.
更多查看译文
关键词
Heteroscedastic Bayesian optimization,Gaussian processes,Generalized product of experts,Augmented expected improvement
AI 理解论文
溯源树
样例
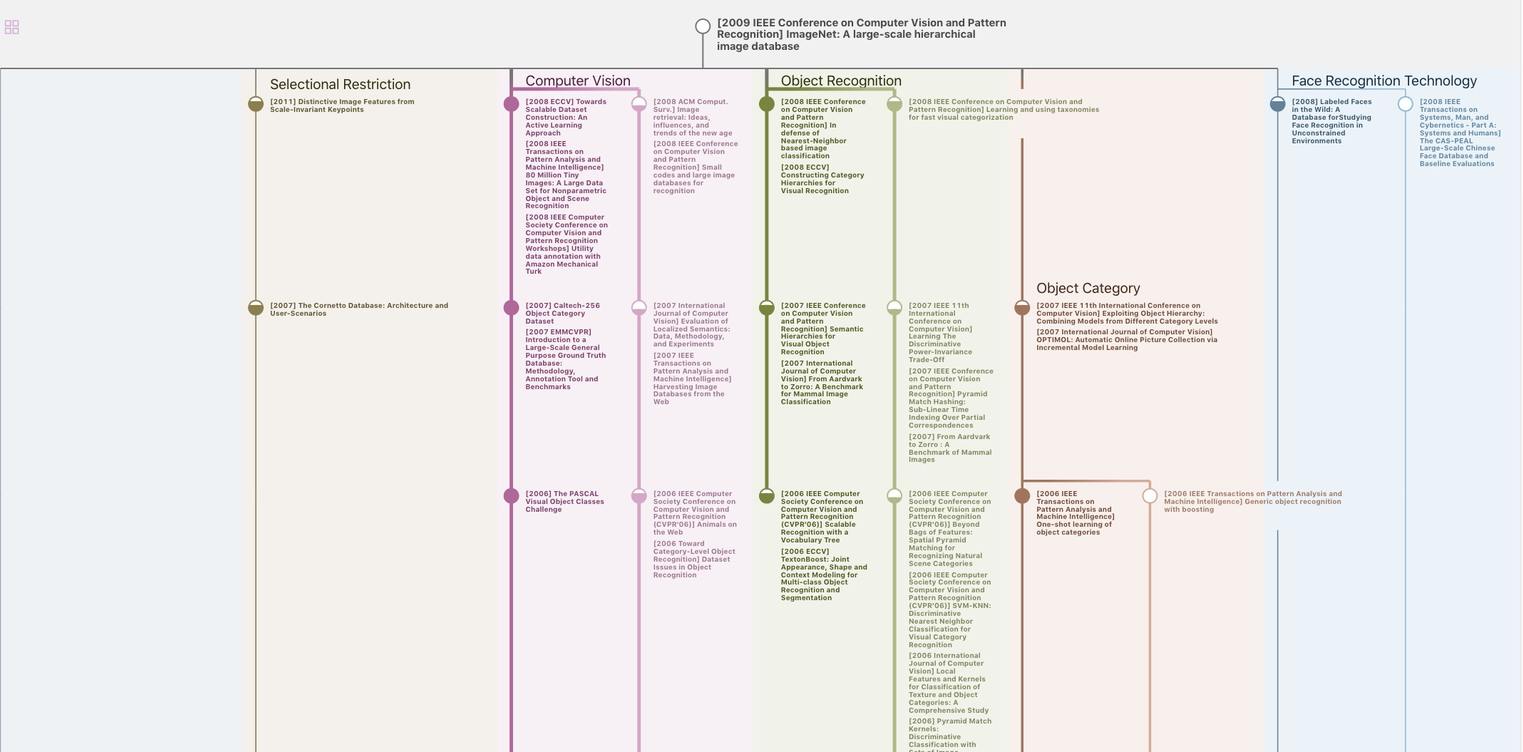
生成溯源树,研究论文发展脉络
Chat Paper
正在生成论文摘要