Predictive Fraud Detection: An Intelligent Method for Internet of Smart Grid Things Systems
JOURNAL OF INTERNET SERVICES AND APPLICATIONS(2023)
摘要
Today, grid resilience as a feature has become non-negotiable, significantly when power interruptions can impact the economy. The widespread popularity of Intelligent Electronic Devices (IED) operating as smart meters enables an immense amount of fine-grained electricity consumption data to be collected. However, risks can still exist in the Smart Grid (SG), as valuable data are exchanged among SG systems; theft or alteration of this data could violate consumer privacy. The Internet of Things for Smart Grid (IoSGT) is a promising ecosystem of different technologies that coordinate with each other to pave the way for new SG applications and services. As a use case of IoSGT for future SG applications and services, fraud detection, iNon-technical losses (NTL), emerges as an important application for Smart Grid (SG) scenarios. A substantial amount of electrical energy is lost throughout the distribution system, and these losses are divided into two types: technical and non-technical. Non-technical losses (NTL) are any electrical energy consumed and not invoiced. They may occur due to illegal connections, issues with energy meters such as delay in the installation or reading errors, contaminated, defective, or non-adapted measuring equipment, very low valid consumption estimates, faulty connections, and disregarded customers. Non-technical losses are the primary cause of revenue loss in the SG. According to a recent study, electrical utilities lose $89.3 Billion per year due to non-technical losses. This article proposes ensemble predictor-based time series classifiers for NTL detection. The proposed predictor ministers the user's energy consumption as a data input for classification, from splitting the data to executing the classifier.It encompasses the temporal aspects of energy consumption data during preprocessing, training, testing, and validation stages. The suggested predictor is Time Series (TS) oriented, from data splitting to the classifier's performance. Overall, our best results have been recorded in the fraud detectionbased time series classifiers (TSC) model scoring an improvement in the empirical performance metrics by 10% or more over the other developed models.
更多查看译文
关键词
Smart Grid,Non-technical losses,Ensemble,TSC,Edge Computing
AI 理解论文
溯源树
样例
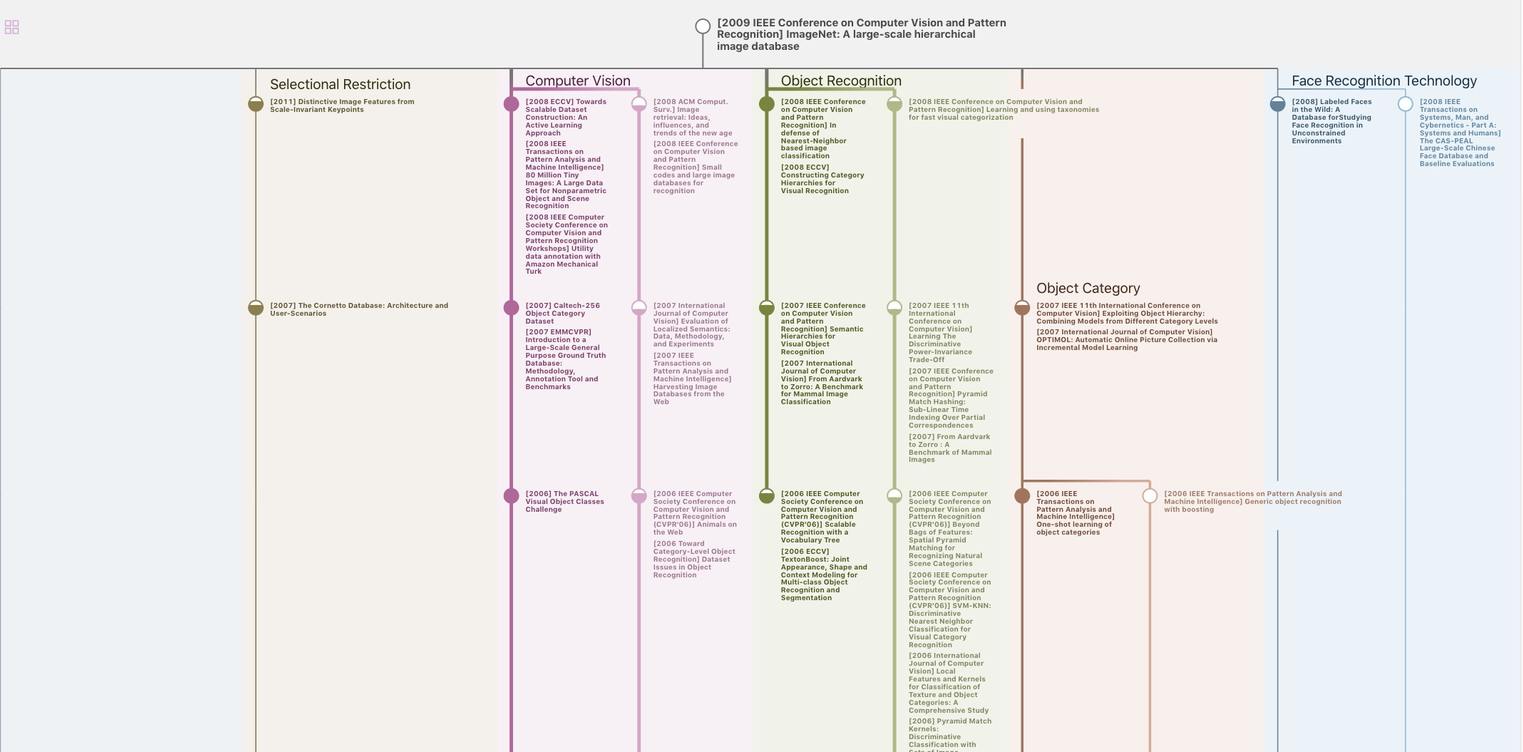
生成溯源树,研究论文发展脉络
Chat Paper
正在生成论文摘要