Adaptive Intelligence for Batteryless Sensors Using So.ware-Accelerated Tsetlin Machines
PROCEEDINGS OF THE TWENTIETH ACM CONFERENCE ON EMBEDDED NETWORKED SENSOR SYSTEMS, SENSYS 2022(2022)
摘要
Tsetlin Machine (TM) is a new machine learning algorithm that encodes propositional logic into learning automata-a set of logical expressions composed of boolean input features-to recognise patterns. The simplicity, efficiency, and accuracy of this logic-based algorithm encourage rethinking the application of traditional arithmetic-based neural networks (NNs) in intelligent sensors design. Indeed, TM is a promising candidate for embedding intelligence into tiny batteryless sensors with the potential to address two critical challenges: (1) computing under resource constraints and (2) demand for dynamic adaptation to the unpredictable nature of harvested energy. However, its structural model complexity manifests in two conflicting issues: large memory footprint and long latency. This paper addresses these shortcomings by proposing adaptive compression techniques exploiting the inherent redundancies observed in trained models. Through dynamically scaling the computational complexity based on available energy, our techniques significantly reduce the memory footprint and speed up the runtime execution. We evaluate our techniques against standard TMs and binarized neural networks (BNNs) for vision and acoustic workloads deployed on a TI MSP430 MCU operating under intermittent power supply conditions. We show that our techniques can achieve up to 99% compression of TM models and offer 13.5. latency and energy reductions when compared with the most efficient neural network configuration without compromising accuracy.
更多查看译文
关键词
Tsetlin Machines, Neural Networks, Energy Efficiency, Intermittent Computing, Battery-free
AI 理解论文
溯源树
样例
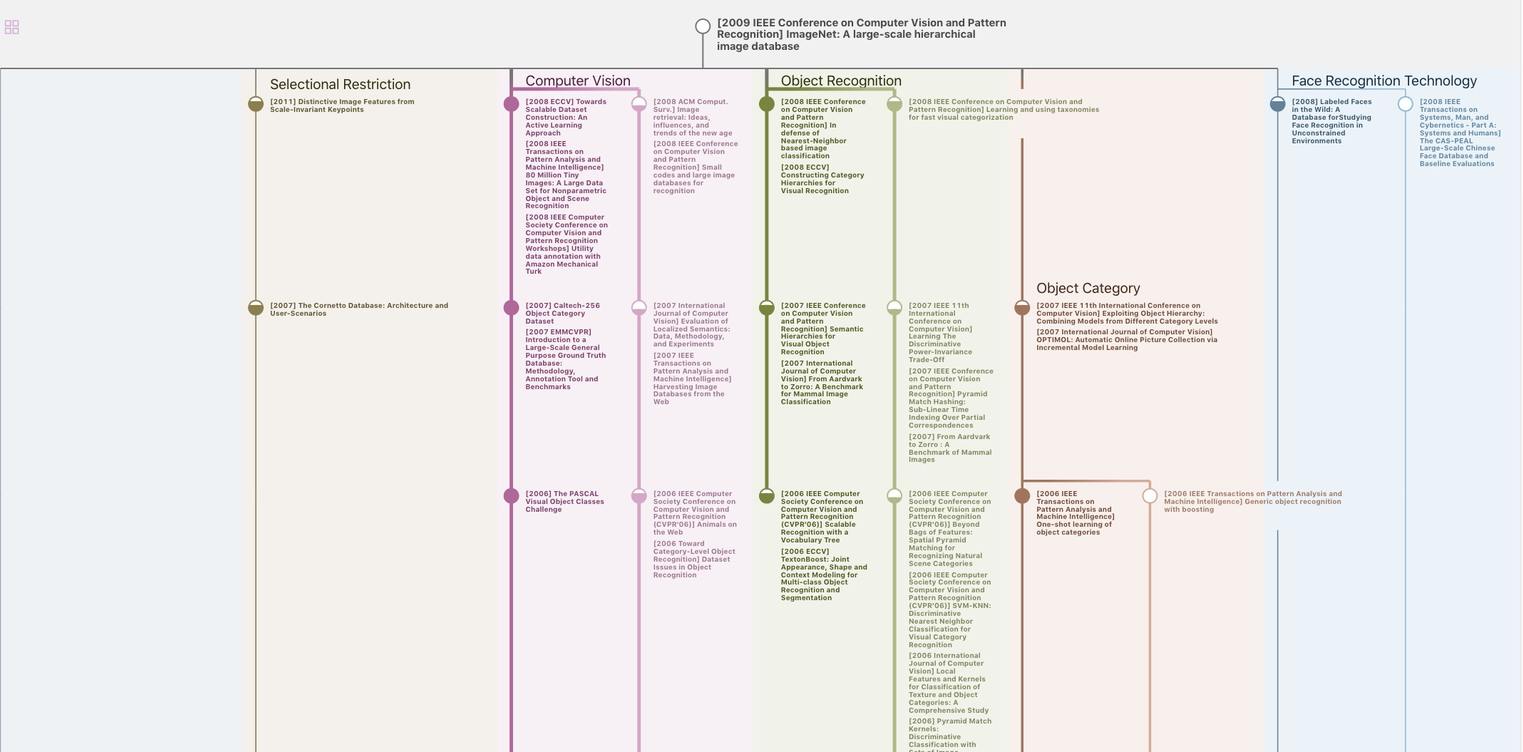
生成溯源树,研究论文发展脉络
Chat Paper
正在生成论文摘要