Inherently interpretable machine learning solutions to differential equations
ENGINEERING WITH COMPUTERS(2023)
摘要
A machine learning method for the discovery of analytic solutions to differential equations is assessed. The method utilizes an inherently interpretable machine learning algorithm, genetic programming-based symbolic regression. An advantage of its interpretability is the output of symbolic expressions that can be used to assess error in algebraic terms, as opposed to purely numerical quantities. Therefore, models output by the developed method are verified by assessing its ability to recover known analytic solutions for two differential equations, as opposed to assessing numerical error. To demonstrate its improvement, the developed method is compared to a conventional, purely data-driven genetic programming-based symbolic regression algorithm. The reliability of successful evolution of the true solution, or an algebraic equivalent, is demonstrated.
更多查看译文
关键词
Physics-informed machine learning,Symbolic regression,Genetic programming,Boundary-value problems
AI 理解论文
溯源树
样例
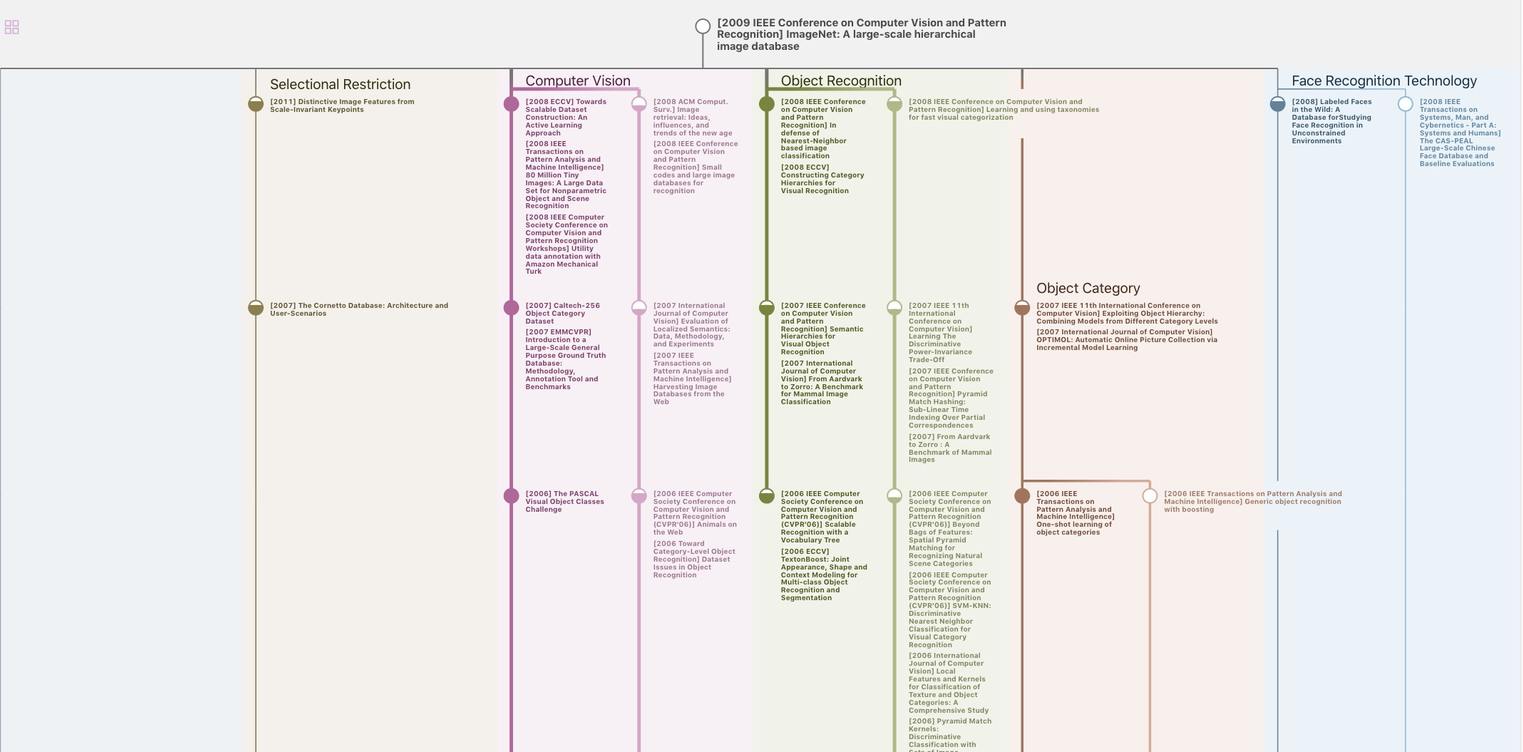
生成溯源树,研究论文发展脉络
Chat Paper
正在生成论文摘要