A multiscale bidirectional fuzzy-driven learning network for remote sensing image segmentation
INTERNATIONAL JOURNAL OF REMOTE SENSING(2023)
摘要
Semantic segmentation is a fundamental but meaningful task in the remote sensing image understanding community. Great progress has been made in optical sensor photography technology, which poses an opportunity and a challenge for remote sensing image segmentation task. But, in fact, a longstanding and intractable problem is that many hard pixels in special position, i.e. the prevalent intra-class noise and a poor boundary delineation, is difficult to classify due to their inherent uncertainty. In this paper, we comprehensively consider the characteristics of deep learning and introduce traditional pattern recognition methods to drive structure learning, which can leverage the corresponding fuzzy logic model to alleviate the aforementioned problem in remote sensing images. Specifically, this paper designs a multiscale bidirectional fuzzy-driven learning network (MBFNet), which takes advantage of both deep learning and fuzzy logic to effectively alleviate the inherent uncertainty of these hard pixels. The structure of convolutional neural networks driven by fuzzy systems also provides a new modelling paradigm for solving the uncertain problem in remote sensing images. Meanwhile, multiscale techniques and bidirectional fusion are introduced to enhance feature aggregation and avoid the potential adverse effects of fuzzy systems, respectively. Experimental results on two datasets demonstrate qualitatively and quantitatively that the proposed MBFNet is competitive.
更多查看译文
关键词
remote sensing,image segmentation,learning network,multiscale bidirectional,fuzzy-driven
AI 理解论文
溯源树
样例
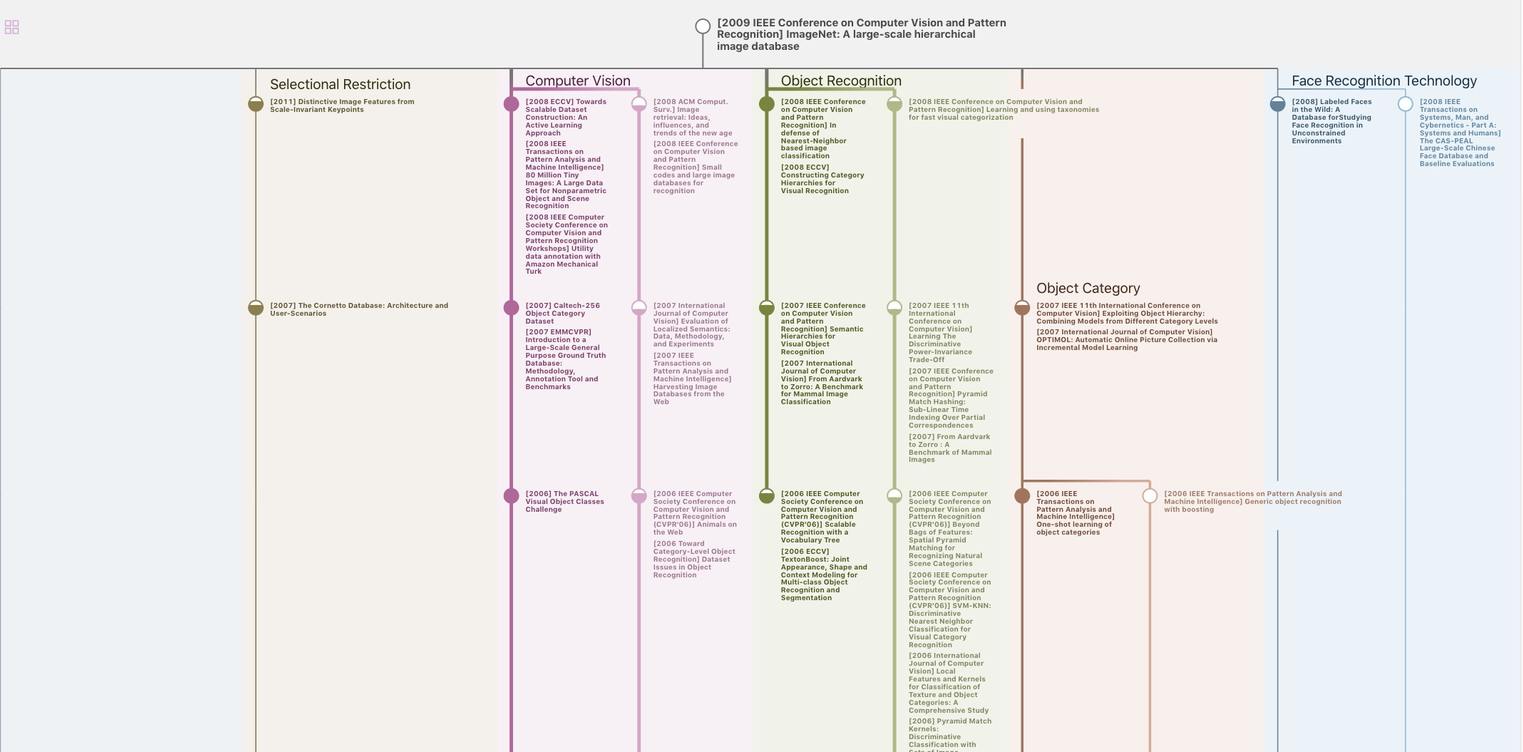
生成溯源树,研究论文发展脉络
Chat Paper
正在生成论文摘要