Toward precision psychiatry: machine learning-driven patient stratification of major depressive disorder reveals biologically distinct subtypes
EUROPEAN NEUROPSYCHOPHARMACOLOGY(2023)
摘要
Major depressive disorder (MDD) is a leading cause of disability affecting ∼322M people worldwide, yet treatments offer limited efficacy across symptoms. Patients with MDD are clinically and biologically heterogeneous, which complicates the identification of causal mechanisms that can inform therapeutic development. Our study aimed to leverage machine learning (ML) and the vast constellation of data available in the UK Biobank (UKB) to identify subtypes of individuals with probable MDD (pMDD) and assess differences in their neurobiological and genetic architecture. The study included UKB data from health records, blood and urine biomarkers, self-reported questionnaires, cognitive assessments, neuroimaging data (T1-weighted MRI, diffusion tensor imaging (DTI)), and genetics in up to 500K individuals. pMDD cases and controls were defined by inclusion/exclusion criteria based on self-reported information, clinical diagnoses, and medication use (Howard et al. 2018). An XGBoost classifier and explainable AI framework was used to select key features that classified pMDD cases vs. controls. We applied k-means clustering using the top 50 most informative features, reduced with auto-encoder, for patient subtyping. Genetic characterization of subtypes included genome-wide variant-level association analyses using REGENIE and genetic correlation analyses using HDL. Neuroanatomic characterization included Firth logistic regression and analysis of covariance to identify significant T1-wighted MRI and DTI brain regions of interest associated with pMDD subtypes. Analysis included 60,813 pMDD cases and 231,787 controls. Predictive performance of the pMDD classifier was 73%. Eight distinct clusters representing subtypes of pMDD were observed. For example, Cluster 0 was driven by mental disorders, substance abuse, and higher testosterone levels, and Cluster 2 by lipid metabolism, peripheral nerve disorders, and higher LDL cholesterol and testosterone. Cluster 7 was driven by suicidal ideation and substance abuse, reflecting a more severe MDD subtype. GWAS of pMDD subtypes revealed 6 independent, cluster-specific, significant loci, with genetic correlation revealing differences in genetic architecture across the eight clusters. Further genetic characterization of subtypes against external summary statistics for suicide attempts (Mullins et al., 2022) revealed highest genetic correlation with Cluster 7, supporting its identification as a severe subtype driven by shared genetic underpinnings for suicide risk. Neuromorphometric differences across subtypes were observed for measures of structural connectivity (DTI), but not for measures of brain thickness, area, or volume. Significant alternations in fractional anisotropy were observed in the anterior corona radiata of Cluster 2; posterior thalamic radiation and superior corona radiata of Cluster 0, and the tapetum of Cluster 7, all relative to controls. Our findings demonstrate the utility of ML-driven approaches in stratifying complex diseases into genetic and neurobiologically distinct subtypes to improve our understanding of disease etiology and reveal the underlying mechanisms driving clinical heterogeneity. Insights from research applying ML-driven approaches hold potential to enable precision psychiatry in drug development—facilitating novel target identification and informing clinical trials that ultimately pair the patient to the right drug at the right time.
更多查看译文
关键词
precision psychiatry,major depressive disorder,learning-driven
AI 理解论文
溯源树
样例
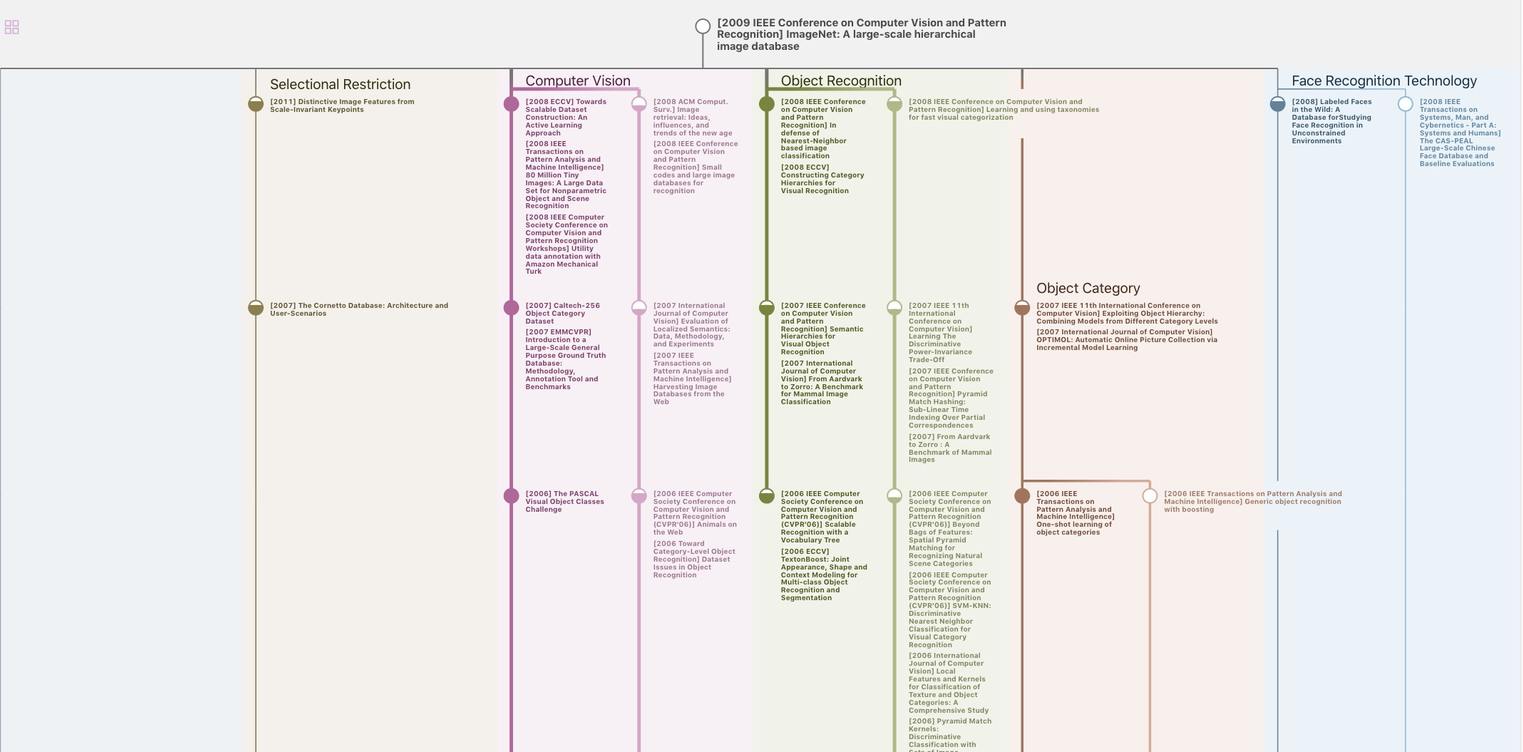
生成溯源树,研究论文发展脉络
Chat Paper
正在生成论文摘要