Depth Evaluation of Tiny Defects on or near Surface Based on Convolutional Neural Network
APPLIED SCIENCES-BASEL(2023)
摘要
Featured Application This study concentrates on the field of intelligent nondestructive testing, presenting a CNN-based method for accurately evaluating the depth of micro-defects on or near a surface. The innovation in this study lies in several key aspects: (1) The establishment of a multi-feature correlation between defect depth and ultrasound time-frequency domain characteristics; (2) The full feature extraction via CWT and region of interest delineation of ultrasound signals aiming at a high training efficiency; (3) The targeted design and optimization of the CNN model.Abstract This paper proposes a method for the detection and depth assessment of tiny defects in or near surfaces by combining laser ultrasonics with convolutional neural networks (CNNs). The innovation in this study lies in several key aspects. Firstly, a comprehensive analysis of changes in ultrasonic signal characteristics caused by variations in defect depth is conducted in both the time and frequency domains, based on discrete frequency spectra and original A-scan signals. Continuous wavelet transform (CWT) is employed to obtain wavelet time-frequency maps, demonstrating the consistent characteristics of this image with crack depth variations. A crucial innovation in this research involves the targeted design and optimization of the model based on the characteristics of ultrasonic signals and dataset size. This includes aspects such as data preparation, CNN architecture construction, and hyperparameter selection. The model is tested using a random validation set, which effectively demonstrates the CNN model's validity and high precision. The proposed method enables the recognition and depth assessment of tiny defects on or near surfaces.
更多查看译文
关键词
tiny defects,convolutional neural network,neural network,surface
AI 理解论文
溯源树
样例
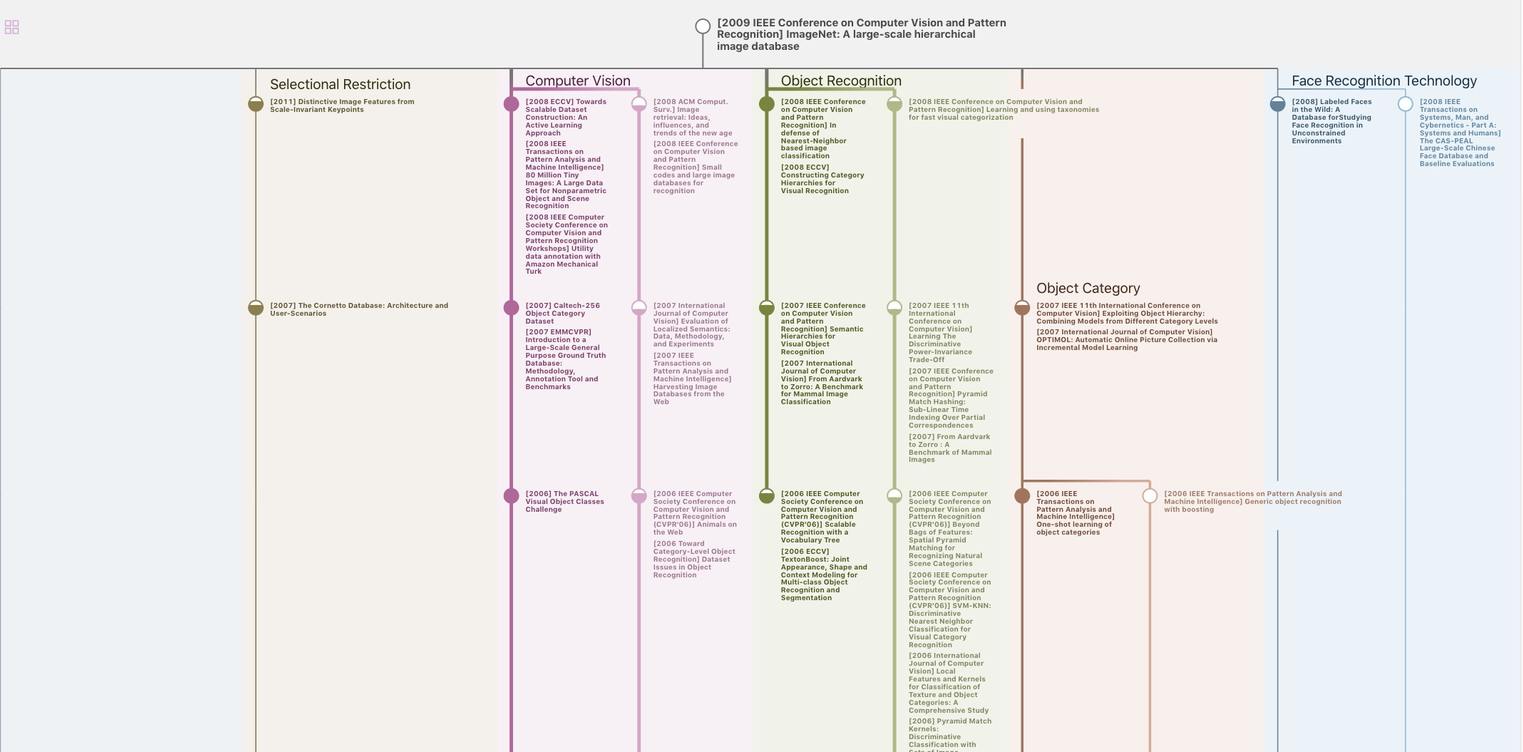
生成溯源树,研究论文发展脉络
Chat Paper
正在生成论文摘要