An Energy Efficient All-Digital Time-Domain Compute-in-Memory Macro Optimized for Binary Neural Networks
IEEE TRANSACTIONS ON CIRCUITS AND SYSTEMS I-REGULAR PAPERS(2024)
摘要
The deployment of neural networks on edge devices has created a growing need for energy-efficient computing. In this paper, we propose an all-digital standard cell-based time-domain compute-in-memory (TDCIM) macro for binary neural networks (BNNs) that is compatible with commercial digital design flow. The TDCIM macro utilizes multiple computing chains that share one threshold chain, and supports double-edge operation, parallel computing and data reuse. Time-domain wave-pipelining technique is introduced to enhance throughput while preserving accuracy. Regular placement (RP) and custom routing (CR) are employed during place and route (P&R) to reduce systematic variations. We show computing delay, POOL computation accuracy, and network test accuracy at different voltages, indicating that the proposed TDCIM macro can maintain high accuracy under PVT variations. We implemented two versions of the TDCIM macro in 22nm FDSOI technology using foundry-provided delay cells DLY40 and DLY60, respectively. At a voltage of 0.5V, the TDCIM macro achieved an energy efficiency of 1.2 (1.05) POPS/W for DLY40 (DLY60), while maintaining a baseline accuracy of 98.9% on the MNIST dataset for both designs.
更多查看译文
关键词
Time-domain,compute-in-memory,binary neural network,all-digital implementation,double-edge operation,wave-pipelining
AI 理解论文
溯源树
样例
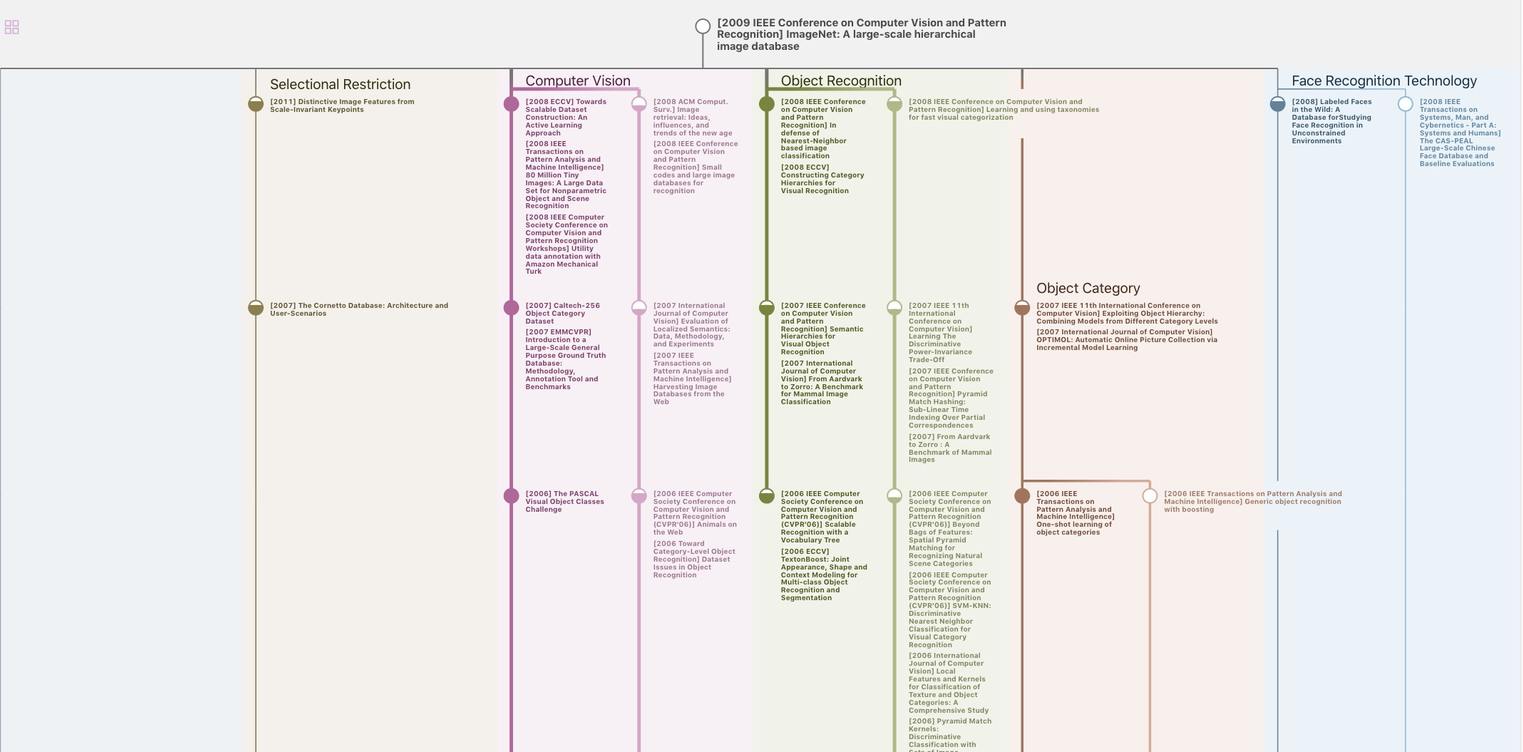
生成溯源树,研究论文发展脉络
Chat Paper
正在生成论文摘要