NIDALoc: Neurobiologically Inspired Deep LiDAR Localization
IEEE TRANSACTIONS ON INTELLIGENT TRANSPORTATION SYSTEMS(2023)
摘要
Absolute pose regression has shown great potential in LiDAR localization, which learns to regress 6-DoF LiDAR poses through deep networks. However, recent regression methods suffer from scene ambiguities in challenging scenarios, leading to inaccurate and unstable localization. Inspired by neurobiological localization mechanisms, i.e., the firing mechanism of place cells, head-direction cells, and grid cells in mammalian brains, we propose a novel LiDAR localization framework called NIDALoc to achieve more robust and accurate results. First, we propose a Hebbian memory module, motivated by place cells, to preserve historical information, which helps refine local view features to reduce scene ambiguities. Specifically, the memory module stores scene information and then recalls it when revisiting an old place. Second, we propose a novel pose constrained framework, consisting of an orientation classification task and a grid center regression task, to regularize orientation and position estimation, respectively. The framework based on head-direction cells and grid cells constrains the absolute pose regression to reduce wrong predictions. Extensive experiments on two outdoor datasets demonstrate the effectiveness of NIDALoc, which outperforms state-of-the-art localization methods, especially in large-scale challenging scenes. The source code is available on the project website at https://github.com/PSYZ1234/NIDALoc.
更多查看译文
关键词
LiDAR localization,absolute pose regression,neurobiological inspired mechanisms
AI 理解论文
溯源树
样例
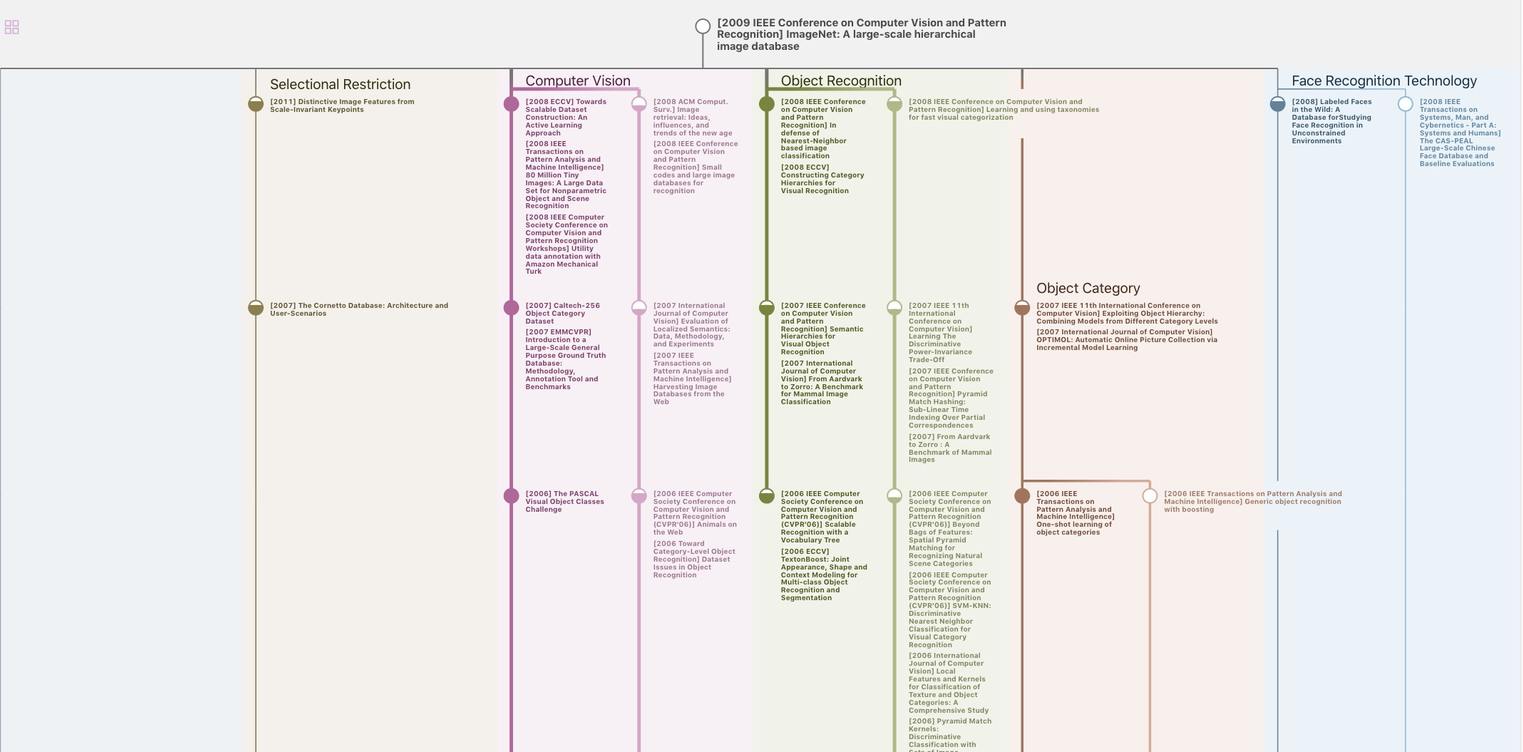
生成溯源树,研究论文发展脉络
Chat Paper
正在生成论文摘要