Vision graph u-net: geometric learning enhanced encoder for medical image segmentation and restoration
INVERSE PROBLEMS AND IMAGING(2023)
摘要
Convolutional neural networks (CNNs) are known for their powerful feature extraction ability, and have achieved great success in a variety of image processing tasks. However, convolution filters only extract local features and neglect long-range self-similarity information, which is the vital prior information commonly existing in image data. To this end, we put forward a new backbone neural network: vision graph U-Net (VGU-Net), which is the first model to construct multi-scale graph structures through the hierarchical down-sampling layers of the U-Net architecture. The graph structure is constructed by the self-attention mechanism. By replacing CNNs in the bottleneck layer and skip connection layers with the graph convolution networks (GCNs), the multi-scale graph structure visualization allows an interpretation of long-range interactions. We extend the VGU-Net backbone model for the widely considered compressed sensing MR image reconstruction task and propose a knowledge-driven deep unrolling scheme based on the half-quadratic splitting algorithm, which combines the interpretability of knowledge-driven model with the versatility of data-driven deep learning method to achieve remarkable reconstruction results. Moreover, we verify the segmentation ability of the VGU-Net backbone model on the multi-modality brain tumor segmentation dataset and white blood cell image segmentation dataset, and both achieve state-of-the-art performance. The code is publicly available at https://github.com/jyh6681/VGU-Net.
更多查看译文
关键词
Vision graph U-Net, geometric deep learning, self-attention, multi, modality medical image segmentation, knowledge-driven deep unrolling scheme
AI 理解论文
溯源树
样例
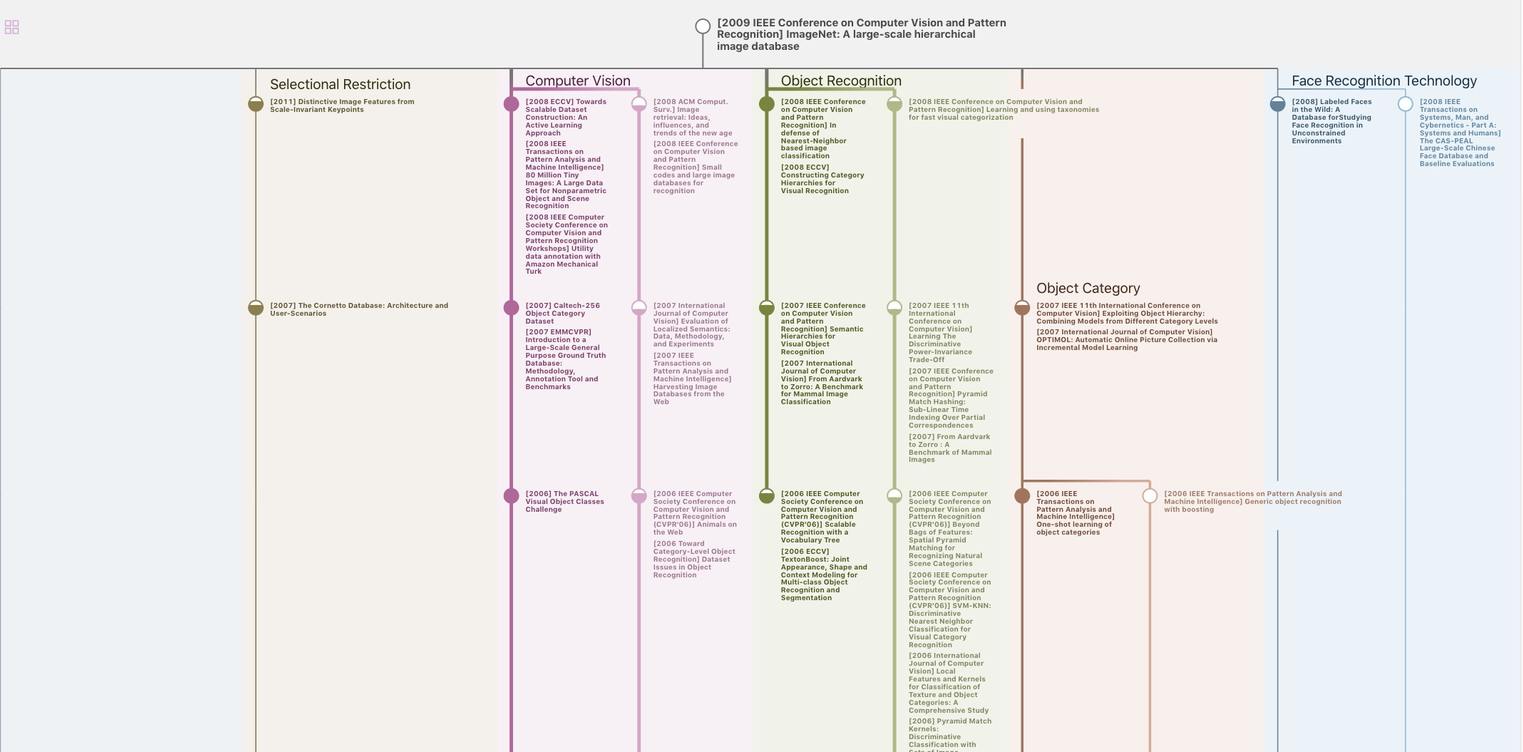
生成溯源树,研究论文发展脉络
Chat Paper
正在生成论文摘要