A novel deep time-index framework with limited data and feature extraction for battery capacity degradation prediction
QUALITY AND RELIABILITY ENGINEERING INTERNATIONAL(2024)
摘要
Accurate capacity estimation and degradation trajectory predictions are crucial part of effectively monitoring the current and future health status of lithium-ion batteries, which ensures their optimal performance and longevity. However, it is hard to acquire precise predictions under various application conditions when relying on limited historical data. In this paper, we propose a novel deep time-index framework based on meta-learning to achieve online capacity estimation and degradation trajectory predictions with only a few monitoring data from early cycles. Highly correlated health feature from lithium-ion battery degradation data is extracted for online capacity estimation. The deep time-index framework consists of three parts, Implicit Neural Representations (INRs), Long Short-Term Memory (LSTM) and Ridge regressor. Cycle numbers are selected as time feature and fed into the INRs to obtain informative representations. A LSTM module is employed to process the temporal representations and capture the long-term dependencies to Ridge regressor to generate a direct multi-step predictions. Three distinct lithium-ion battery degradation datasets are applied to validate the efficacy of the proposed approach. The experimental results validate that within the deep time-index framework, the short-term predictions show remarkable performance. This high level of accuracy makes the proposed deep time-index framework suitable for a wide range of real-world scenarios, as it demonstrates precise predictions even under varying charging and discharging conditions with limited historical data.
更多查看译文
关键词
deep time-index framework,degradation trajectory prediction,few-shot learning,lithium-ion battery,meta-learning
AI 理解论文
溯源树
样例
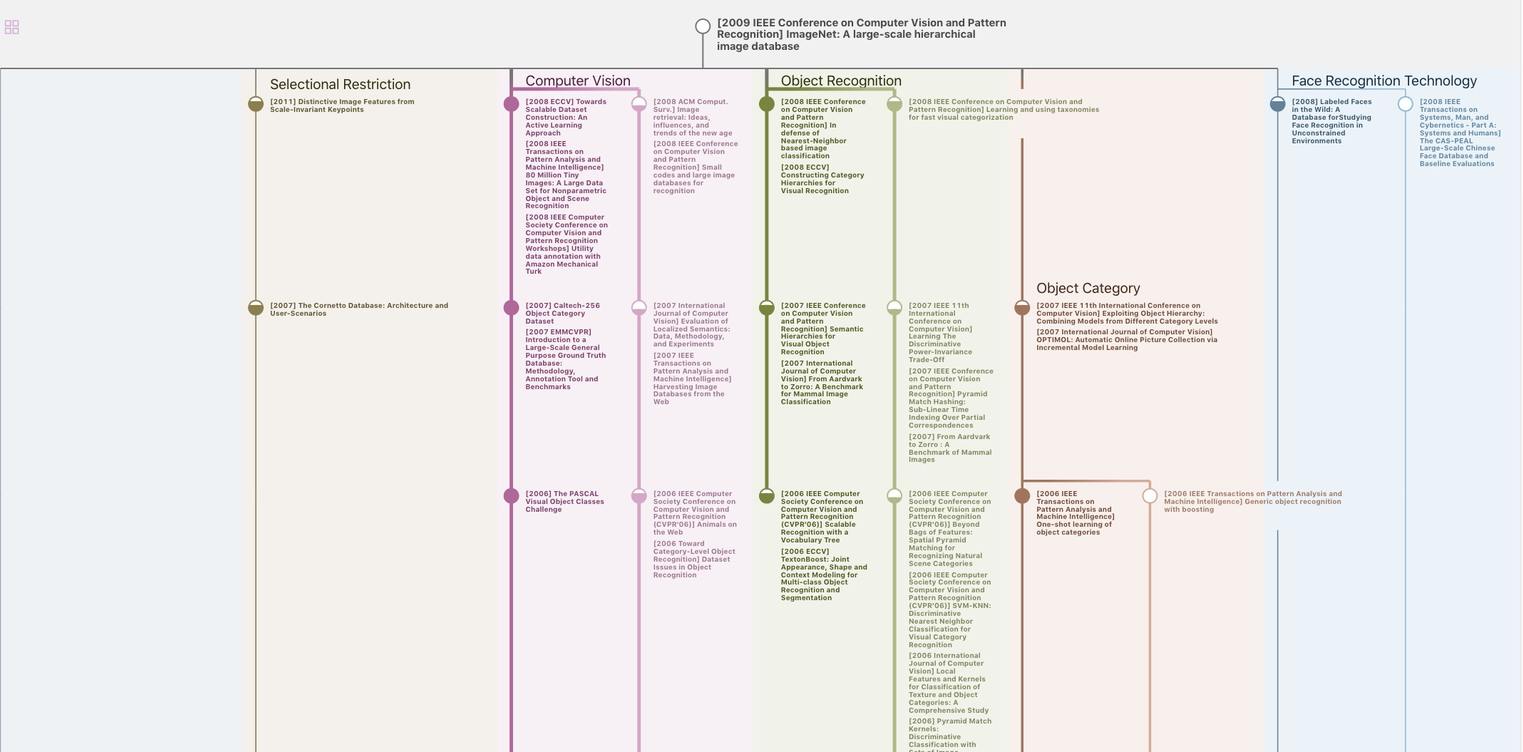
生成溯源树,研究论文发展脉络
Chat Paper
正在生成论文摘要