Development and Validation of Models to Predict Lymph Node Metastasis in Early Gastric Cancer Using Logistic Regression and Gradient Boosting Machine Methods
CANCER RESEARCH AND TREATMENT(2023)
摘要
Purpose This study aimed to identify important features of lymph node metastasis (LNM) and develop a prediction model for early gastric cancer (EGC) using a gradient boosting machine (GBM) method.Materials and Methods The clinicopathologic data of 2,556 patients with EGC who underwent gastrectomy were used as training set and the internal validation set (set 1) at a ratio of 8:2. Additionally, 548 patients with EGC who underwent endoscopic submucosal dissection (ESD) as the initial treatment were included in the external validation set (set 2). The GBM model was constructed, and its performance was compared with that of the Japanese guidelines.Results LNM was identified in 12.6% (321/2,556) of the gastrectomy group (training set & set 1) and 4.3% (24/548) of the ESD group (set 2). In the GBM analysis, the top five features that most affected LNM were lymphovascular invasion, depth, differentiation, size, and location. The accuracy, sensitivity, specificity, and the area under the receiver operating characteristics of set 1 were 0.566, 0.922, 0.516, and 0.867, while those of set 2 were 0.810, 0.958, 0.803, and 0.944, respectively. When the sensitivity of GBM was adjusted to that of Japanese guidelines (beyond the expanded criteria in set 1 [0.922] and eCuraC-2 in set 2 [0.958]), the specificities of GBM in sets 1 and 2 were 0.516 (95% confidence interval, 0.502-0.523) and 0.803 (0.795-0.805), while those of the Japanese guidelines were 0.502 (0.488-0.509) and 0.788 (0.780-0.790), respectively.Conclusion The GBM model showed good performance comparable with the eCura system in predicting LNM risk in EGCs.
更多查看译文
关键词
Early gastric cancer, Lymphatic metastasis, Prediction, Machine learning
AI 理解论文
溯源树
样例
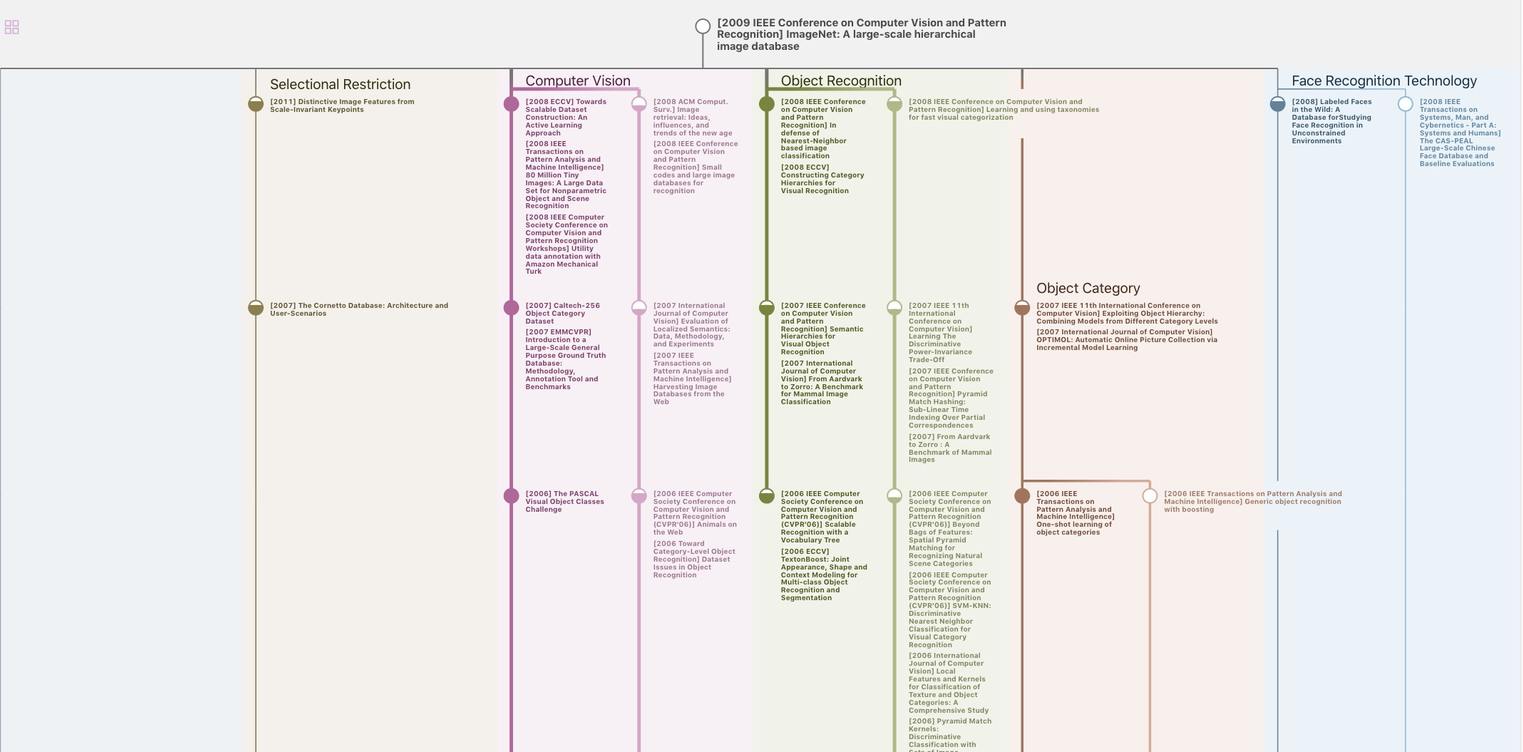
生成溯源树,研究论文发展脉络
Chat Paper
正在生成论文摘要