Adaptive Multi-Feature Fusion Graph Convolutional Network for Hyperspectral Image Classification
REMOTE SENSING(2023)
摘要
Graph convolutional networks (GCNs) are a promising approach for addressing the necessity for long-range information in hyperspectral image (HSI) classification. Researchers have attempted to develop classification methods that combine strong generalizations with effective classification. However, the current HSI classification methods based on GCN present two main challenges. First, they overlook the multi-view features inherent in HSIs, whereas multi-view information interacts with each other to facilitate classification tasks. Second, many algorithms perform a rudimentary fusion of extracted features, which can result in information redundancy and conflicts. To address these challenges and exploit the strengths of multiple features, this paper introduces an adaptive multi-feature fusion GCN (AMF-GCN) for HSI classification. Initially, the AMF-GCN algorithm extracts spectral and textural features from the HSIs and combines them to create fusion features. Subsequently, these three features are employed to construct separate images, which are then processed individually using multi-branch GCNs. The AMG-GCN aggregates node information and utilizes an attention-based feature fusion method to selectively incorporate valuable features. We evaluated the model on three widely used HSI datasets, i.e., Pavia University, Salinas, and Houston-2013, and achieved accuracies of 97.45%, 98.03%, and 93.02%, respectively. Extensive experimental results show that the classification performance of the AMF-GCN on benchmark HSI datasets is comparable to those of state-of-the-art methods.
更多查看译文
关键词
attention mechanism,graph convolution network,hyperspectral image classification,multi-view
AI 理解论文
溯源树
样例
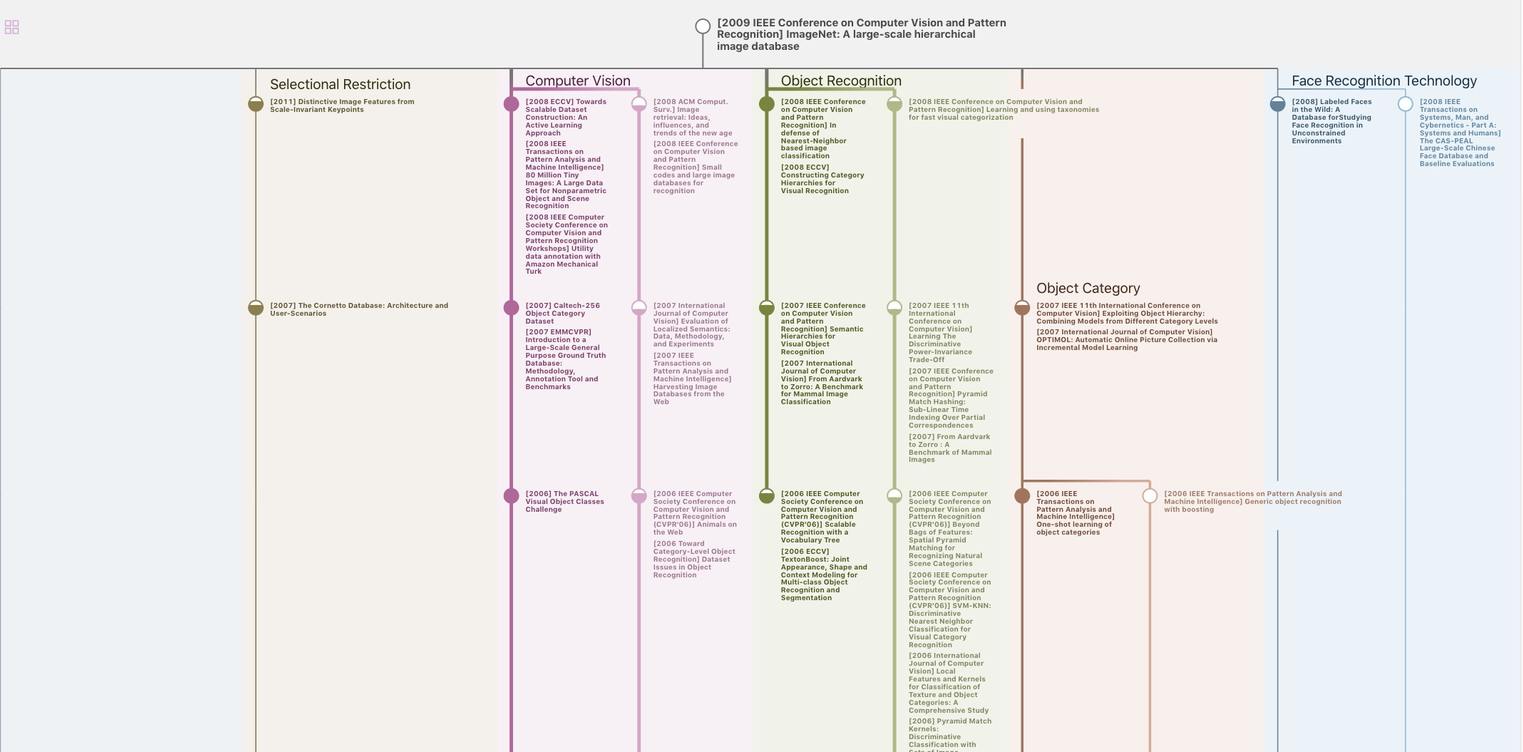
生成溯源树,研究论文发展脉络
Chat Paper
正在生成论文摘要