Motorcycle Detection and Collision Warning Using Monocular Images from a Vehicle
REMOTE SENSING(2023)
摘要
Motorcycle detection and collision warning are essential features in advanced driver assistance systems (ADAS) to ensure road safety, especially in emergency situations. However, detecting motorcycles from videos captured from a car is challenging due to the varying shapes and appearances of motorcycles. In this paper, we propose an integrated and innovative remote sensing and artificial intelligence (AI) methodology for motorcycle detection and distance estimation based on visual data from a single camera installed in the back of a vehicle. Firstly, MD-TinyYOLOv4 is used for detecting motorcycles, refining the neural network through SPP (spatial pyramid pooling) feature extraction, Mish activation function, data augmentation techniques, and optimized anchor boxes for training. The proposed algorithm outperforms eight existing YOLO versions, achieving a precision of 81% at a speed of 240 fps. Secondly, a refined disparity map of each motorcycle's bounding box is estimated by training a Monodepth2 with a bilateral filter for distance estimation. The proposed fusion model (motorcycle's detection and distance from vehicle) is evaluated with depth stereo camera measurements, and the results show that 89% of warning scenes are correctly detected, with an alarm notification time of 0.022 s for each image. Outcomes indicate that the proposed integrated methodology provides an effective solution for ADAS, with promising results for real-world applications, and can be suitable for running on mobility services or embedded computing boards instead of the super expensive and powerful systems used in some high-tech unmanned vehicles.
更多查看译文
关键词
measurements,videos,motorcycle detection,distance estimation,crash prevention,YOLO,monodepth
AI 理解论文
溯源树
样例
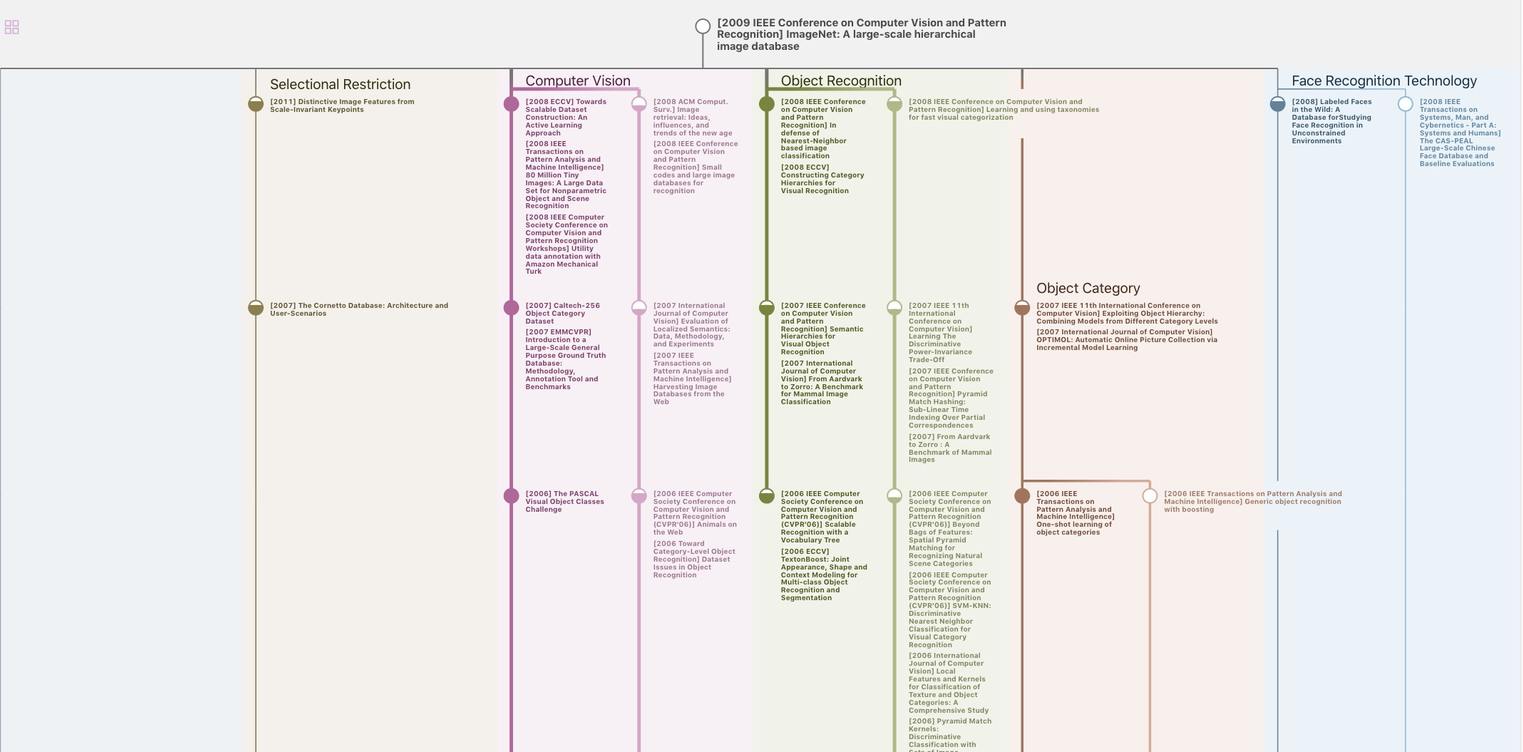
生成溯源树,研究论文发展脉络
Chat Paper
正在生成论文摘要